Revealing architectural order with polarized light imaging and deep neural networks
bioRxiv(2019)
摘要
Quantitative imaging of biological architecture with fluorescent labels is not as scalable as genomic or proteomic measurements. Here, we combine quantitative label-free imaging and deep neural networks for scalable analysis of complex structures. We reconstruct quantitative three-dimensional density, anisotropy, and orientation in live cells and tissue slices from polarization- and depth-resolved images. We report a computationally efficient variant of U-Net architecture that predicts a 3D fluorescent structure from its morphology and physical properties. We evaluate the performance of our models by predicting F-actin and nuclei in mouse kidney tissue. Further, we report label-free imaging of axon tracts and predict level of myelination in human brain tissue sections. We demonstrate the model’s ability to rescue inconsistent labeling. We anticipate that the proposed approach will enable quantitative analysis of architectural order across scales of organelles to tissues.
更多查看译文
关键词
Label-free Microscopy,Polarization,Phase,Computational Imaging,Deep Learning
AI 理解论文
溯源树
样例
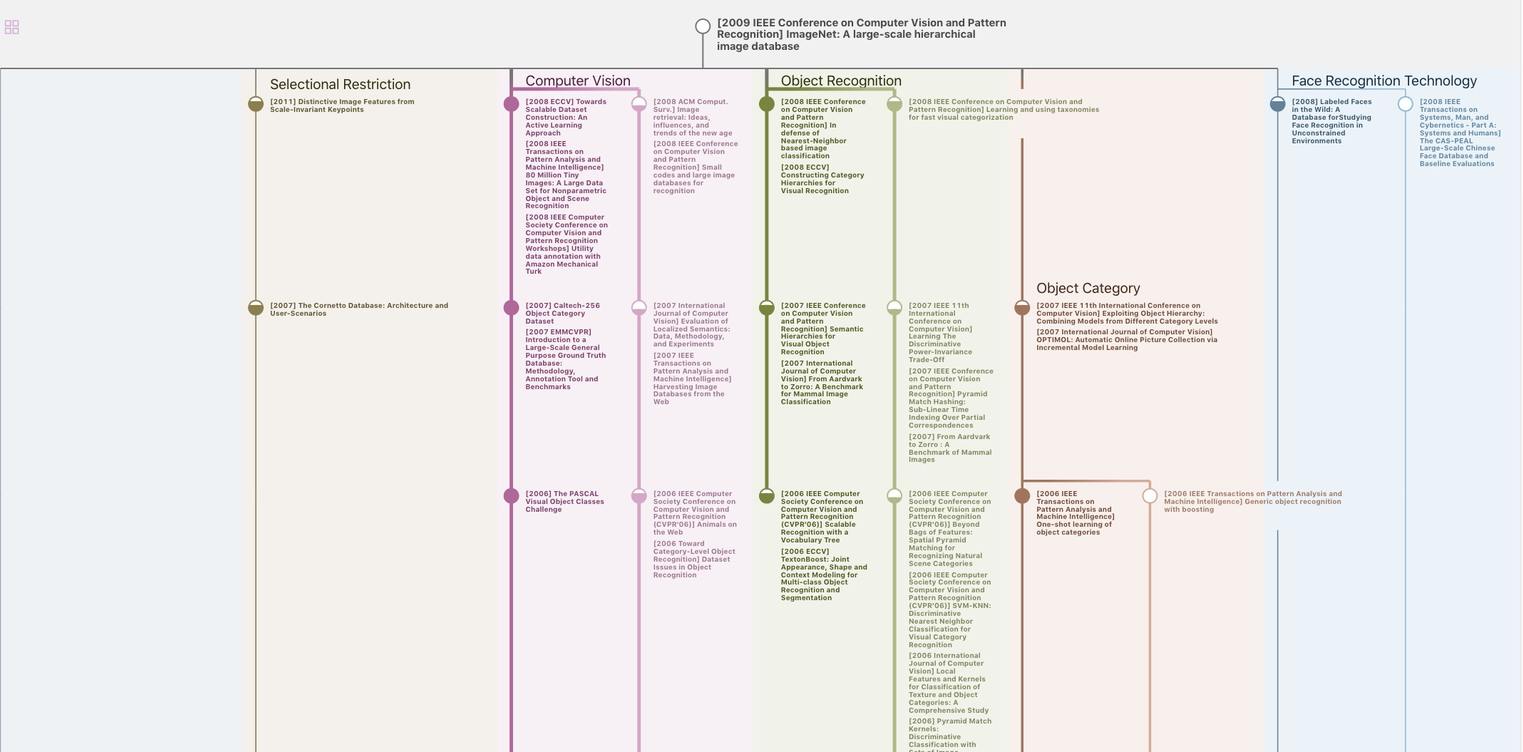
生成溯源树,研究论文发展脉络
Chat Paper
正在生成论文摘要