Application of artificial neural networks in micromechanics for polycrystalline metals
International Journal of Plasticity(2019)
摘要
Machine learning techniques are widely used to understand and predict data trends and therefore can provide a huge computational advantage over conventional numerical techniques. In this work, an artificial neural network (ANN) model is coupled with a rate-dependant crystal plasticity finite element method (CPFEM) formulation to predict the stress-strain behavior and texture evolution in AA6063-T6 under uniaxial tension and simple shear. Firstly, stress-strain and texture evolution results from the crystal plasticity simulations were verified with experimental observations for AA6063-T6 under simple shear and tension. Next, results from crystal plasticity simulations were used to train, validate and test the ANN model. The proposed ANN framework, was successfully applied on single crystal simulation results to predict stress-strain and texture data. Then, the proposed ANN framework was applied to predict the stress-strain curves and texture evolution of AA6063-T6 during uniaxial tension and simple shear. The flexibility of the proposed ANN model was also tested, for simple shear, with a completely new data set and the predicted results showed excellent agreement with corresponding crystal plasticity simulations. Finally, the predictive capability of the proposed model was further demonstrated by successfully validating the ANN model for non-proportional loading paths such as uniaxial tension followed by simple shear and simple shear followed by tension. The results presented in this research clearly demonstrate that the proposed ANN model provided significant computational time improvements without any major sacrifice in accuracy.
更多查看译文
关键词
Artificial neural network,Crystal plasticity,Texture,Aluminium alloys
AI 理解论文
溯源树
样例
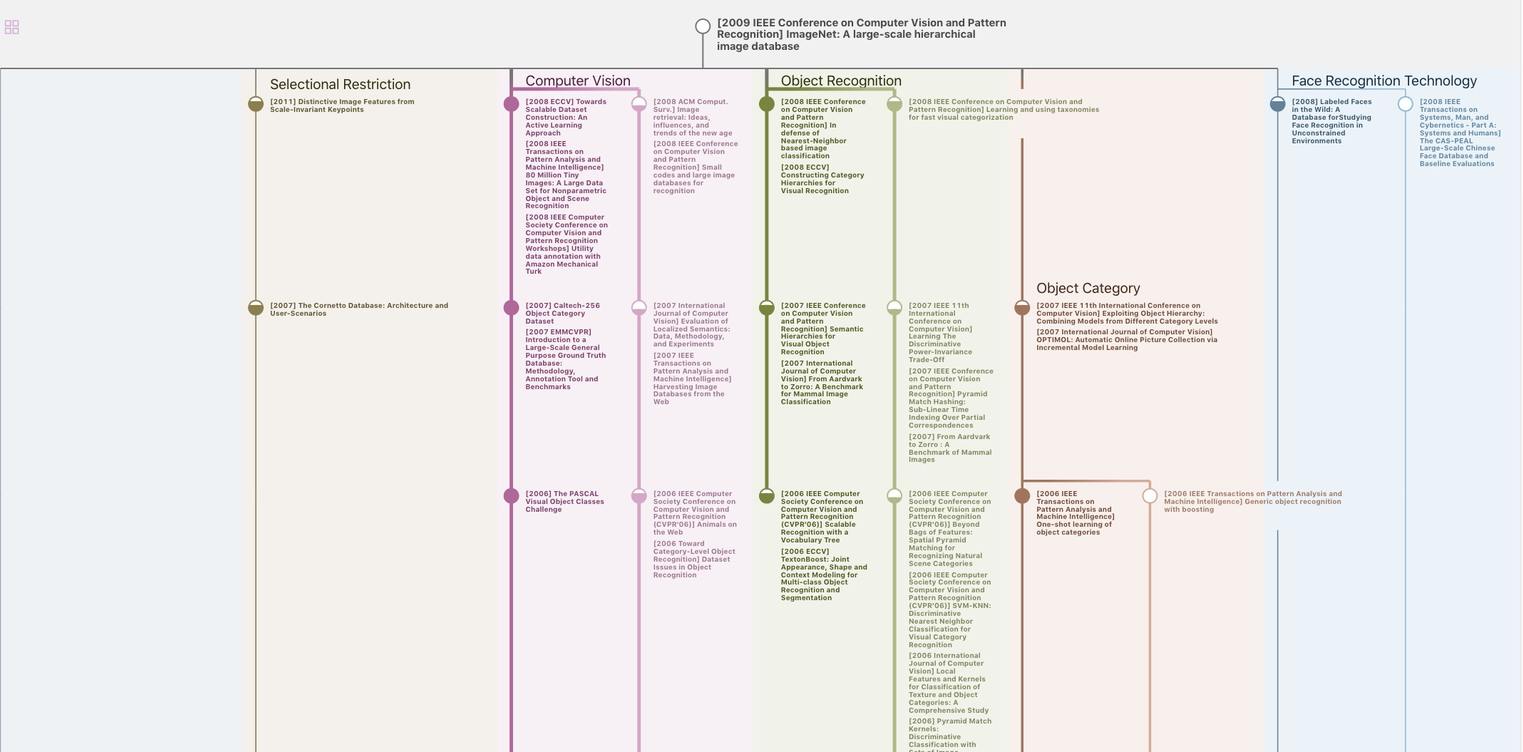
生成溯源树,研究论文发展脉络
Chat Paper
正在生成论文摘要