Uni-Detect: A Unified Approach to Automated Error Detection in Tables
Proceedings of the 2019 International Conference on Management of Data(2019)
Abstract
Data errors are ubiquitous in tables. Extensive research in this area has resulted in a rich variety of techniques, each often targeting a specific type of errors, e.g., numeric outliers, constraint violations, etc. While these diverse techniques clearly improve data quality, it places a significant burden on humans to configure these techniques with suitable rules and parameters for each data set. For example, an expert is expected to define suitable functional-dependencies between column pairs, or tune appropriate thresholds for outlier-detection algorithms, all of which are specific to one individual data set. As a result, users today often hire experts to cleanse only their high-value data sets. We propose \sj, a unified framework to automatically detect diverse types of errors. Our approach employs a novel "what-if'' analysis that performs local data perturbations to reason about data abnormality, leveraging classical hypothesis-tests on a large corpus of tables. We test \sj on a wide variety of tables including Wikipedia tables, and make surprising discoveries of thousands of FD violations, numeric outliers, spelling mistakes, etc., with better accuracy than existing algorithms specifically designed for each type of errors. For example, for spelling mistakes, \sj outperforms the state-of-the-art spell-checker from a commercial search engine.
MoreTranslated text
Key words
constraints, data quality, error detection, outliers
AI Read Science
Must-Reading Tree
Example
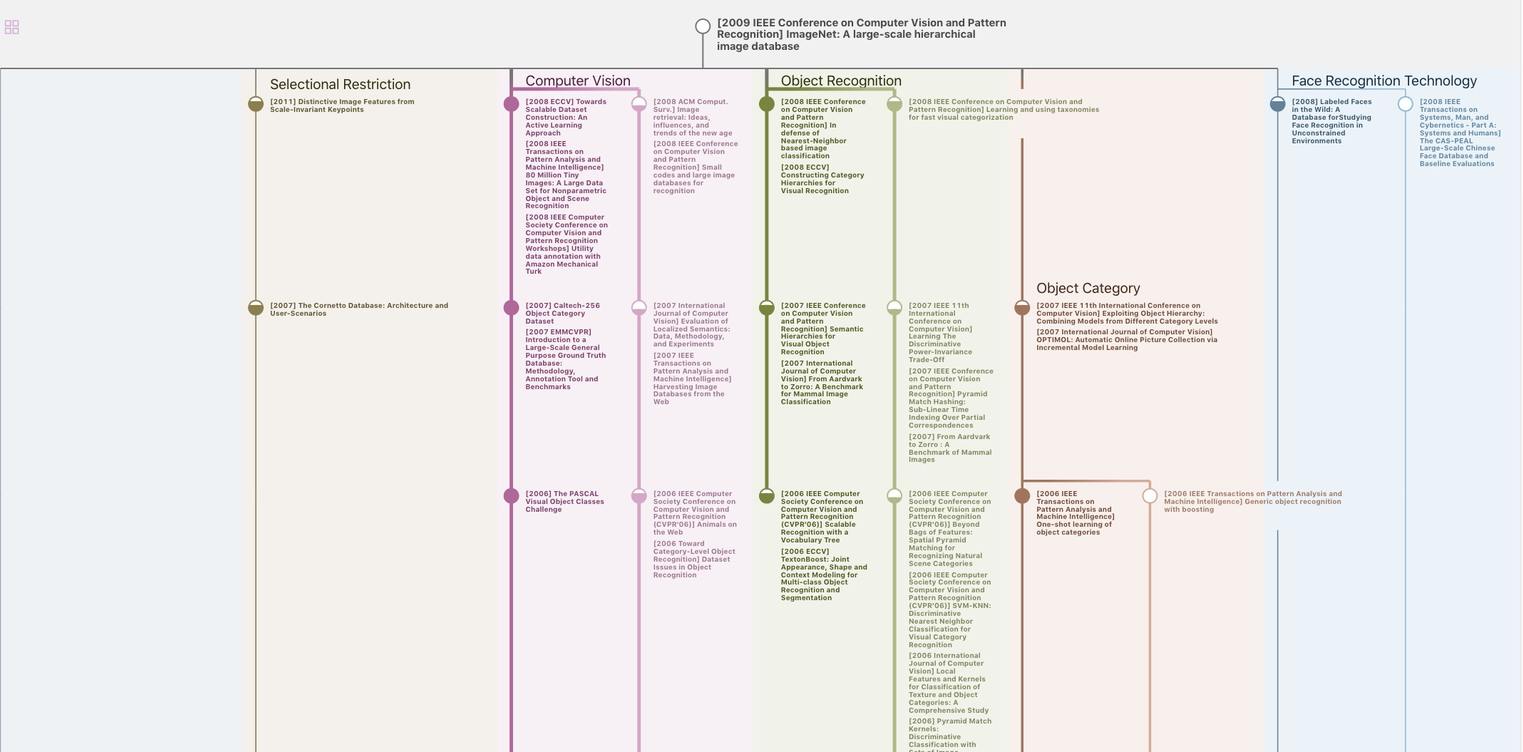
Generate MRT to find the research sequence of this paper
Chat Paper
Summary is being generated by the instructions you defined