General classification of light curves using extreme boosting
arxiv(2019)
摘要
A significant degree of misclassification of variable stars through the application of machine learning methods to survey data motivates a search for more reliable and accurate machine learning procedures, especially in light of the very large data cubes that will be generated by future surveys and the need for immediate production of accurate, formalised catalogues of variable behaviour to enable science to proceed. In this study, the efficiency of an ensemble machine learning procedure utilising extreme boosting was determined by application to a large sample of data from the OGLE III and IV surveys and from the \textit{Kepler} mission. Through recursive training of classifiers, the study developed a variable star classification workflow which produced an average efficiency determined with the average precision of the model (0.81 for \textit{Kepler} and 0.91 for OGLE) and the $f-score$ of predictions on the test sets. This suggests that extreme boosting can be presented as one of the favourable shallow learning methods in developing a variable star classifier for future large survey projects.
更多查看译文
AI 理解论文
溯源树
样例
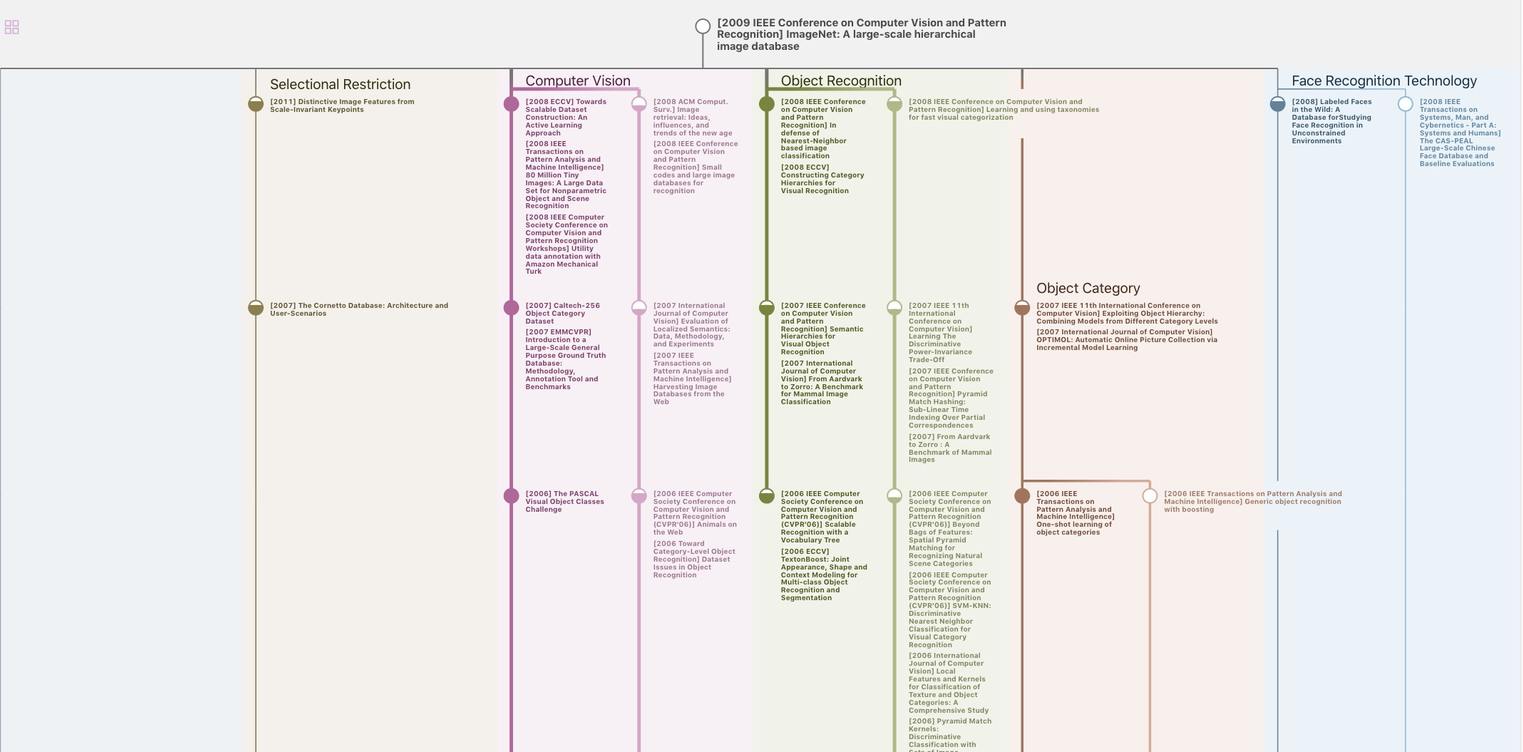
生成溯源树,研究论文发展脉络
Chat Paper
正在生成论文摘要