Intelligent Localization Of Transformer Internal Degradations Combining Deep Convolutional Neural Networks And Image Segmentation
IEEE ACCESS(2019)
Abstract
Industrial 4.0 placed higher demands on the field of intelligent equipment monitoring. The transformer is one of the critical power devices, its intelligent monitoring and fault positioning require indepth studies. In this study, an efficient fault localization method for transformer internal thermal faults was proposed by introducing different deep convolutional neural networks (CNNs) and image segmentation. First, the transformer monitoring images of temperature and velocity fields in fault conditions were simulated using the lattice Boltzmann method (LBM), and the images were also used to highlight features information. In practice, transformer degradation does not frequently occur, so that the fault samples for deep learning are insufficient. To solve this problem, a transfer learning method was employed. Subsequently, fault locations were defined as classification labels, and different CNN's were used to classify the labels to achieve the fault localization results. Next, image segmentation was performed to extract the features of fault areas and simplify the data volumes. Likewise, the CNN's were employed to perform the fault localization again. Afterward, since the monitoring sensors were not located everywhere in a transformer in practical applications, information of partial monitoring areas where the monitoring sensors located was trained following a similar procedure. After image segmentation, the average fault localization accuracy using the information obtained by sensors decreased from 97.95% to 94.42%, while the data volume was reduced to nearly 1% of the original one. Besides, the average calculation time per iteration decreased by 8.816%, while the loss value was reduced by 37.68%. Finally, the Friedman hypothesis test and Nemenyi post hoc test were performed to compare the evaluation indicators of different networks, and the performance of GoogLeNet in this case was considered the best.
MoreTranslated text
Key words
Condition monitoring,fault diagnostics,convolutional neural networks (CNNs),image segmentation,lattice Boltzmann method (LBM),level set,transformers,Friedman test
AI Read Science
Must-Reading Tree
Example
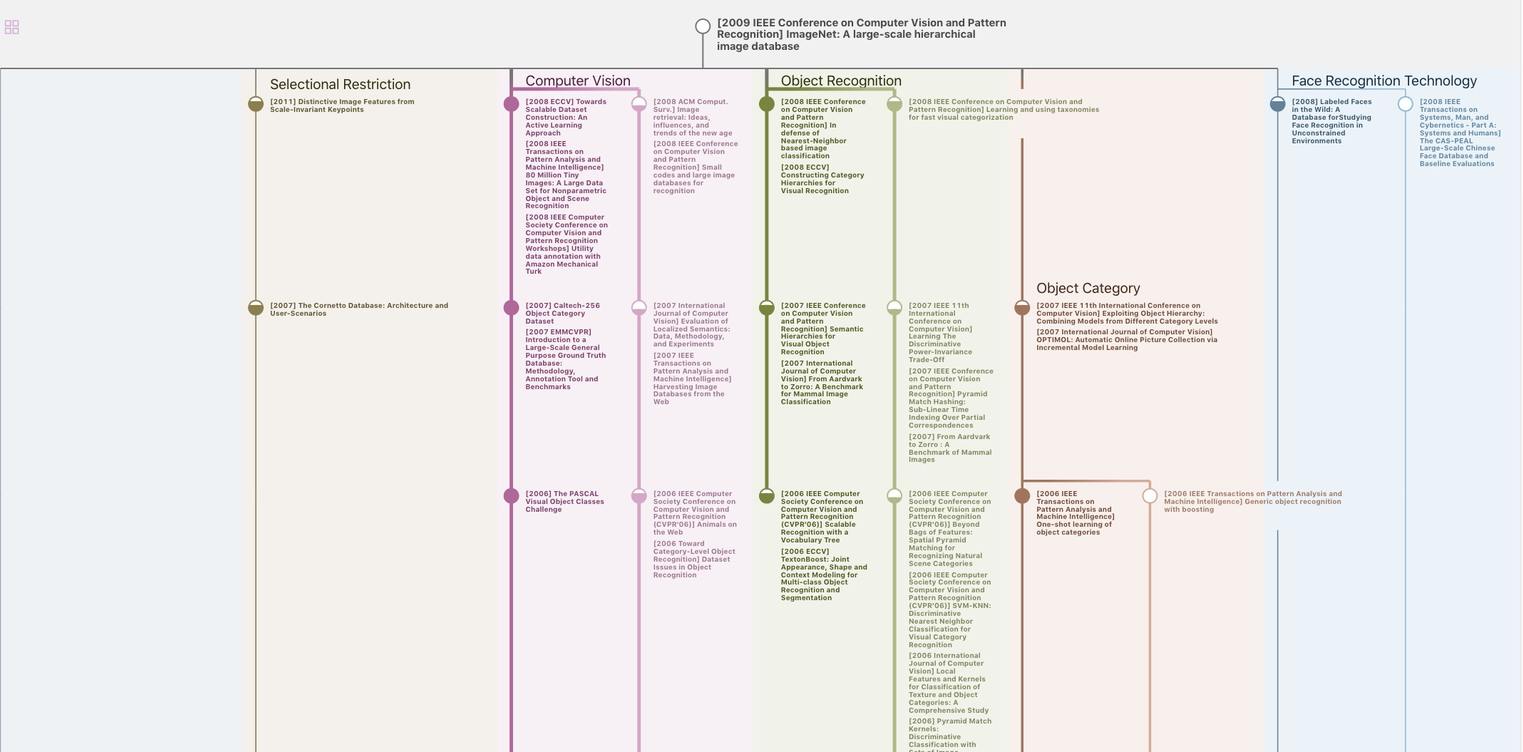
Generate MRT to find the research sequence of this paper
Chat Paper
Summary is being generated by the instructions you defined