Improving the linear relaxation of maximum k -cut with semidefinite-based constraints
EURO Journal on Computational Optimization(2019)
Abstract
We consider the maximum k -cut problem that involves partitioning the vertex set of a graph into k subsets such that the sum of the weights of the edges joining vertices in different subsets is maximized. The associated semidefinite programming (SDP) relaxation is known to provide strong bounds, but it has a high computational cost. We use a cutting-plane algorithm that relies on the early termination of an interior point method, and we study the performance of SDP and linear programming (LP) relaxations for various values of k and instance types. The LP relaxation is strengthened using combinatorial facet-defining inequalities and SDP-based constraints. Our computational results suggest that the LP approach, especially with the addition of SDP-based constraints, outperforms the SDP relaxations for graphs with positive-weight edges and k ≥ 7 .
MoreTranslated text
Key words
Maximum k-cut,Graph partitioning,Semidefinite programming,Eigenvalue constraint,Semi-infinite formulation
AI Read Science
Must-Reading Tree
Example
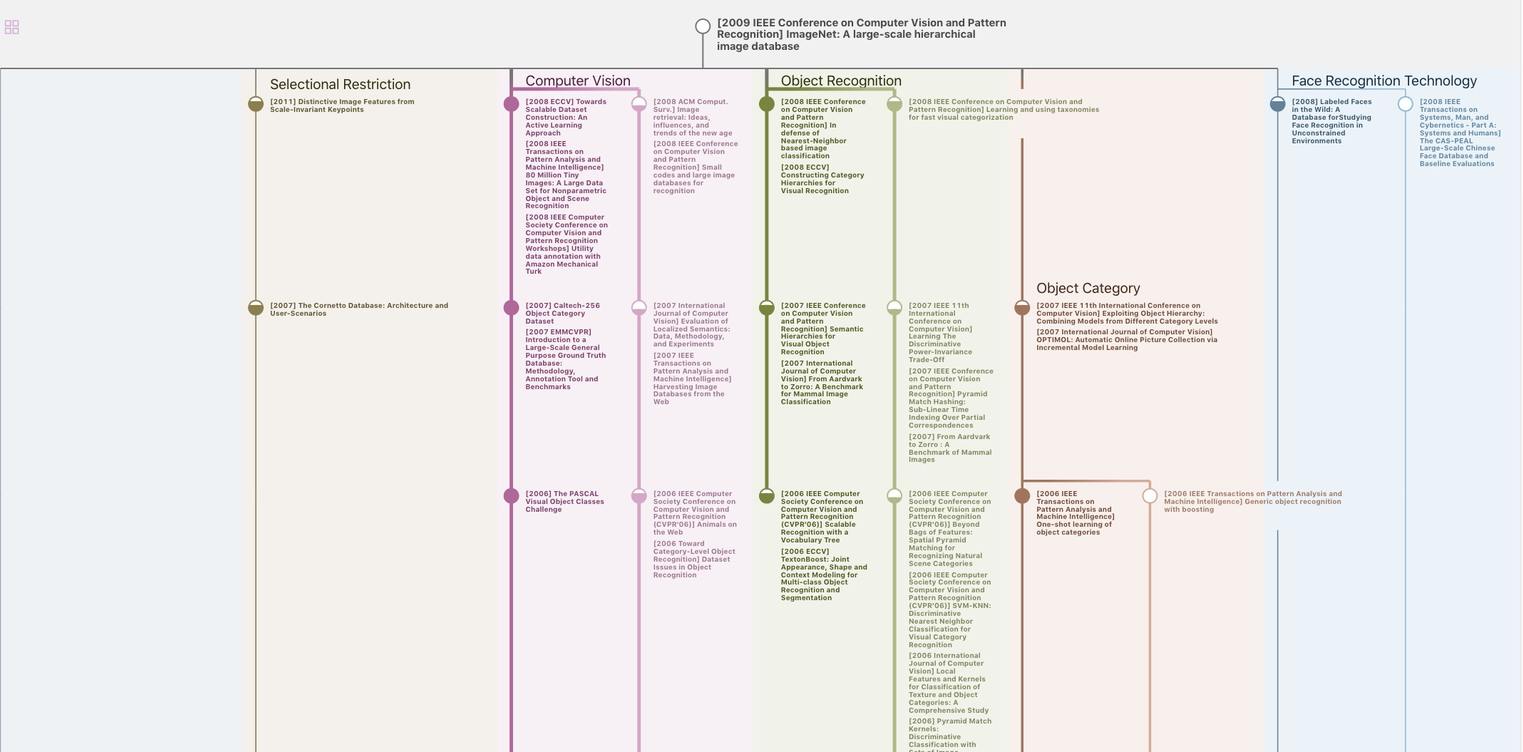
Generate MRT to find the research sequence of this paper
Chat Paper
Summary is being generated by the instructions you defined