Identification of gastrointestinal perforation based on ICD-10 code in a Japanese administrative medical information database and associated drug exposure risk factors.
PHARMACOEPIDEMIOLOGY AND DRUG SAFETY(2019)
摘要
Purpose The purpose of this study is to evaluate the accuracy of gastrointestinal (GI) perforation ICD-10 coding in the Diagnosis Procedure Combination (DPC) database and to examine drug exposure risk factors for GI perforation. Methods A total of 100 patients with GI perforation ICD-10 codes were selected randomly from Kagawa University Hospital's DPC database between April 2011 and December 2016. Two experienced specialist physicians independently reviewed the medical records and classified cases as "definite A," "definite B," "probable," or "no GI perforation." The positive predictive values (PPVs) of "definite A/B" cases were calculated after stratification by sex, age, ICD-10 code, and diagnostic information in the DPC data. The number of prescribed drugs with side effects of GI perforation according to historical data was compared between "definite A/B" and "no GI perforation" cases. Results The overall PPV was 47.0% (95% confidence interval [CI], 36.9-57.2). However, the PPVs for the three categories of diagnostic information in the DPC data ("main diagnosis," "diagnosis causing admission," and "most resource-intensive diagnosis") were each more than 70% after excluding inappropriate patients. Additionally, the PPV focused on these three categories was 76.3% (95% CI, 59.8-88.6). Prescribed drugs with side effects of GI perforation were more frequently detected in "definite A/B" cases (P = .028). Conclusions Although the overall PPV for GI perforation based on ICD-10 code was low, our results suggest that the PPV could be improved by appropriate selection of DPC diagnosis category and that use of multiple medications enhances the risk of GI perforation.
更多查看译文
关键词
Diagnosis Procedure Combination database,gastrointestinal perforation,ICD-10,multiple medications,pharmacoepidemiology,positive predictive value
AI 理解论文
溯源树
样例
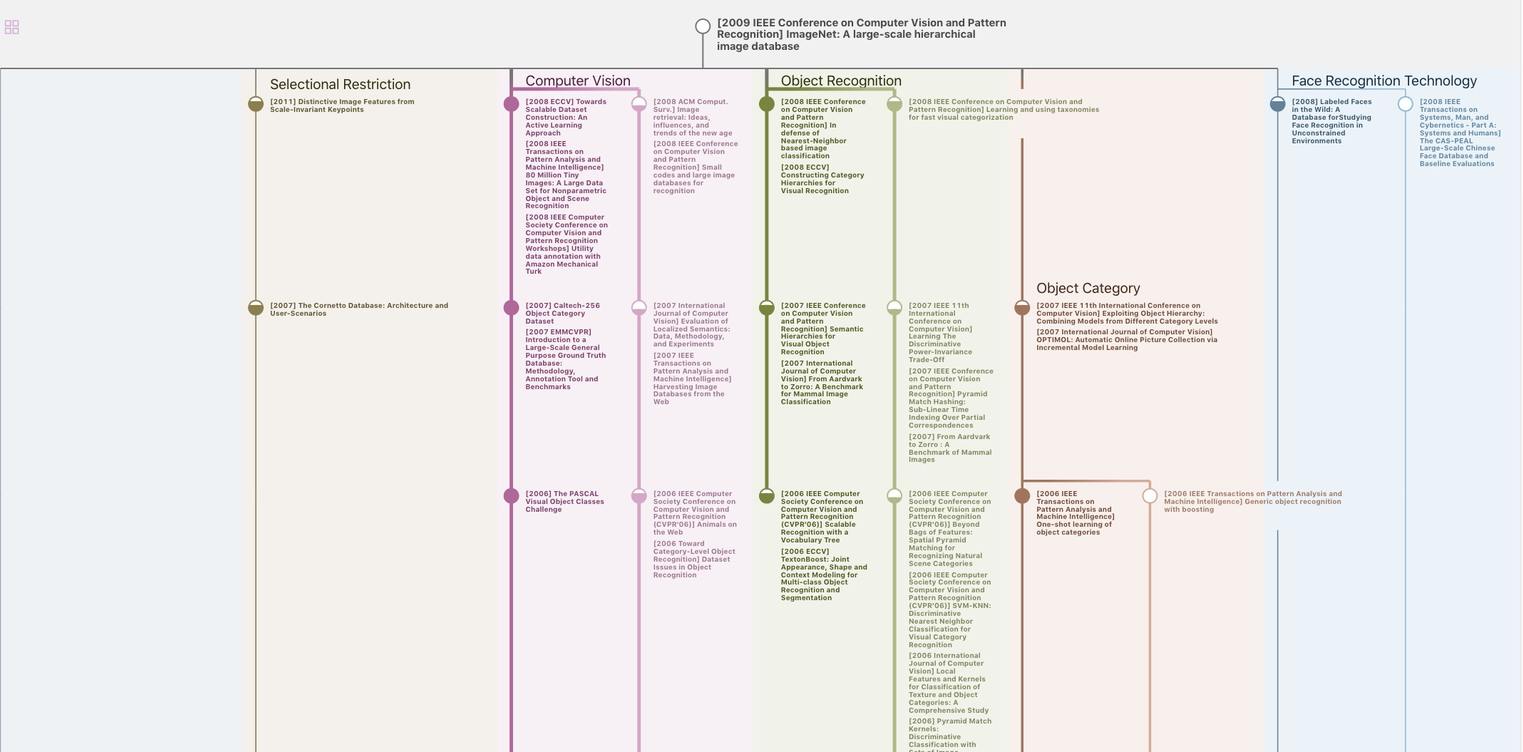
生成溯源树,研究论文发展脉络
Chat Paper
正在生成论文摘要