Adaptive Neural Signal Detection for Massive MIMO
IEEE Trans. Wirel. Commun.(2020)
摘要
Traditional symbol detection algorithms either perform poorly or are impractical to implement for Massive Multiple-Input Multiple-Output (MIMO) systems. Recently, several learning-based approaches have achieved promising results on simple channel models (e.g., i.i.d. Gaussian channel coefficients), but as we show, their performance degrades on real-world channels with spatial correlation. We propose MMNet, a deep learning MIMO detection scheme that significantly outperforms existing approaches on realistic channels with the same or lower computational complexity. MMNet 's design builds on the theory of iterative soft-thresholding algorithms, and uses a novel training algorithm that leverages temporal and spectral correlation in real channels to accelerate training. These innovations make it practical to train MMNet online for every realization of the channel. On i.i.d. Gaussian channels, MMNet requires two orders of magnitude fewer operations than existing deep learning schemes but achieves near-optimal performance. On spatially-correlated channels, it achieves the same error rate as the next-best learning scheme (OAMPNet) at 2.5dB lower signal-to-noise ratio (SNR), and with at least 10x less computational complexity. MMNet is also 4-8dB better overall than a classic linear scheme like the minimum mean square error (MMSE) detector.
更多查看译文
关键词
Massive MIMO,signal detection,deep learning,online adaptation,spatial channel correlation
AI 理解论文
溯源树
样例
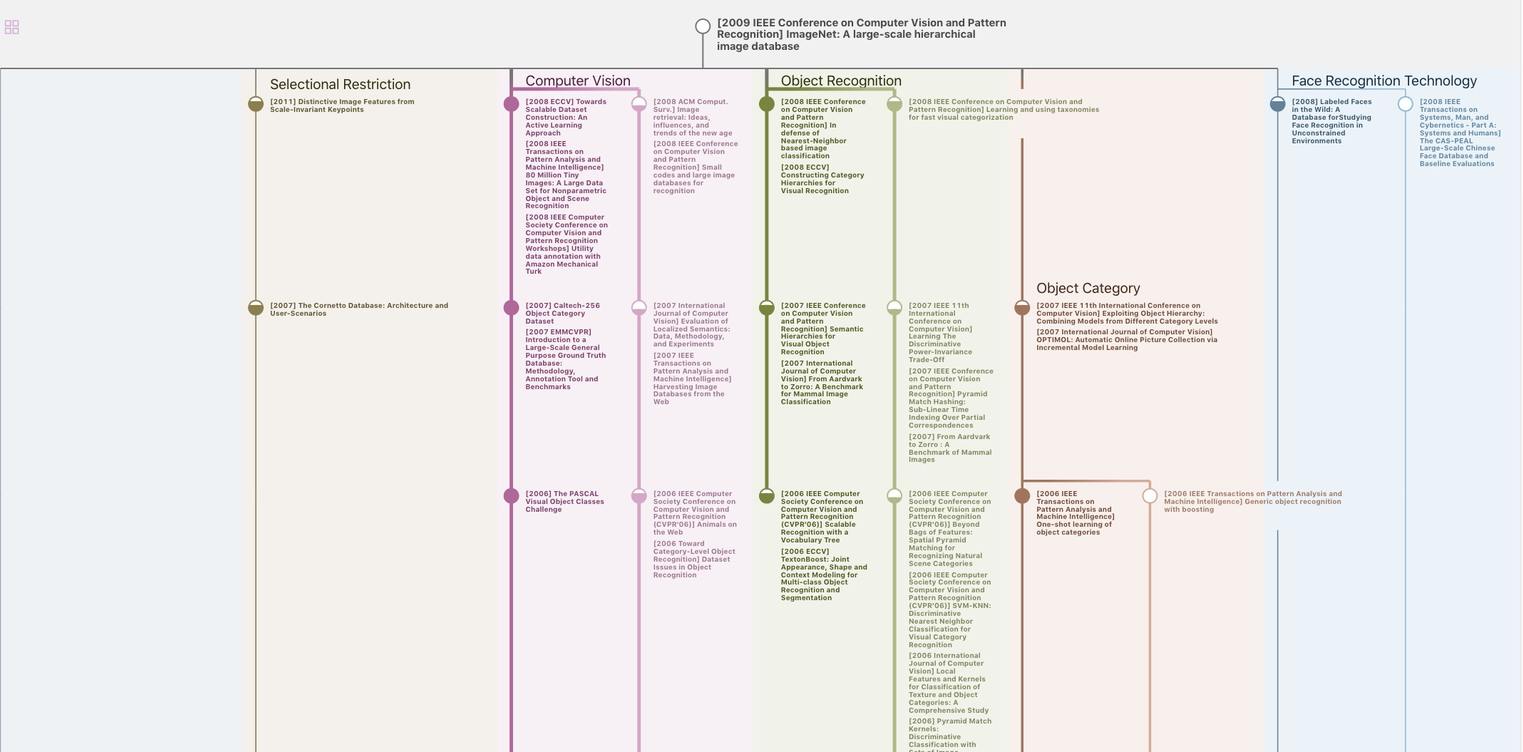
生成溯源树,研究论文发展脉络
Chat Paper
正在生成论文摘要