Evaluation of CT Image Synthesis Methods:From Atlas-based Registration to Deep Learning
arxiv(2019)
摘要
Computed tomography (CT) is a widely used imaging modality for medical diagnosis and treatment. In electroencephalography (EEG), CT imaging is necessary for co-registering with magnetic resonance imaging (MRI) and for creating more accurate head models for the brain electrical activity due to better representation of bone anatomy. Unfortunately, CT imaging exposes patients to potentially harmful sources of ionizing radiation. Image synthesis methods present a solution for avoiding extra radiation exposure. In this paper, we perform image synthesis to create a realistic, synthetic CT image from MRI of the same subject, and we present a comparison of different image synthesis techniques. Using a dataset of 30 paired MRI and CT image volumes, our results compare image synthesis using deep neural network regression, state-of-the-art adversarial deep learning, as well as atlas-based synthesis utilizing image registration. We also present a novel synthesis method that combines multi-atlas registration as a prior to deep learning algorithms, in which we perform a weighted addition of synthetic CT images, derived from atlases, to the output of a deep neural network to obtain a residual type of learning. In addition to evaluating the quality of the synthetic CT images, we also demonstrate that image synthesis methods allow for more accurate bone segmentation using the synthetic CT imaging than would otherwise be possible by segmenting the bone in the MRI directly.
更多查看译文
AI 理解论文
溯源树
样例
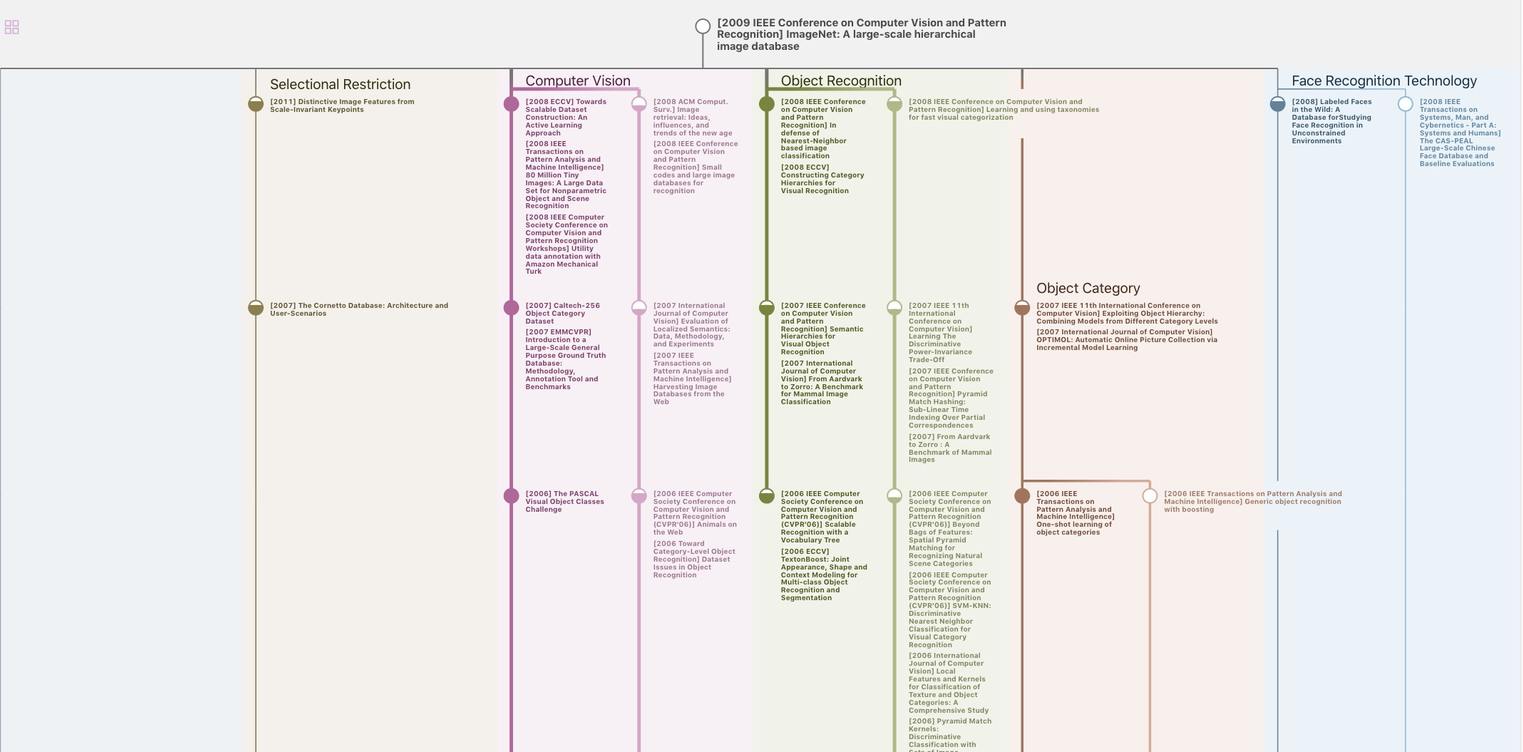
生成溯源树,研究论文发展脉络
Chat Paper
正在生成论文摘要