Dual-Channel Reconstruction Network for Image Compressive Sensing.
SENSORS(2019)
摘要
The existing compressive sensing (CS) reconstruction algorithms require enormous computation and reconstruction quality that is not satisfying. In this paper, we propose a novel Dual-Channel Reconstruction Network (DC-Net) module to build two CS reconstruction networks: the first one recovers an image from its traditional random under-sampling measurements (RDC-Net); the second one recovers an image from its CS measurements acquired by a fully connected measurement matrix (FDC-Net). Especially, the fully connected under-sampling method makes CS measurements represent original images more effectively. For the two proposed networks, we use a fully connected layer to recover a preliminary reconstructed image, which is a linear mapping from CS measurements to the preliminary reconstructed image. The DC-Net module is used to further improve the preliminary reconstructed image quality. In the DC-Net module, a residual block channel can improve reconstruction quality and dense block channel can expedite calculation, whose fusion can improve the reconstruction performance and reduce runtime simultaneously. Extensive experiments manifest that the two proposed networks outperform state-of-the-art CS reconstruction methods in PSNR and have excellent visual reconstruction effects.
更多查看译文
关键词
compressive sensing (CS),reconstruction,dual-channel
AI 理解论文
溯源树
样例
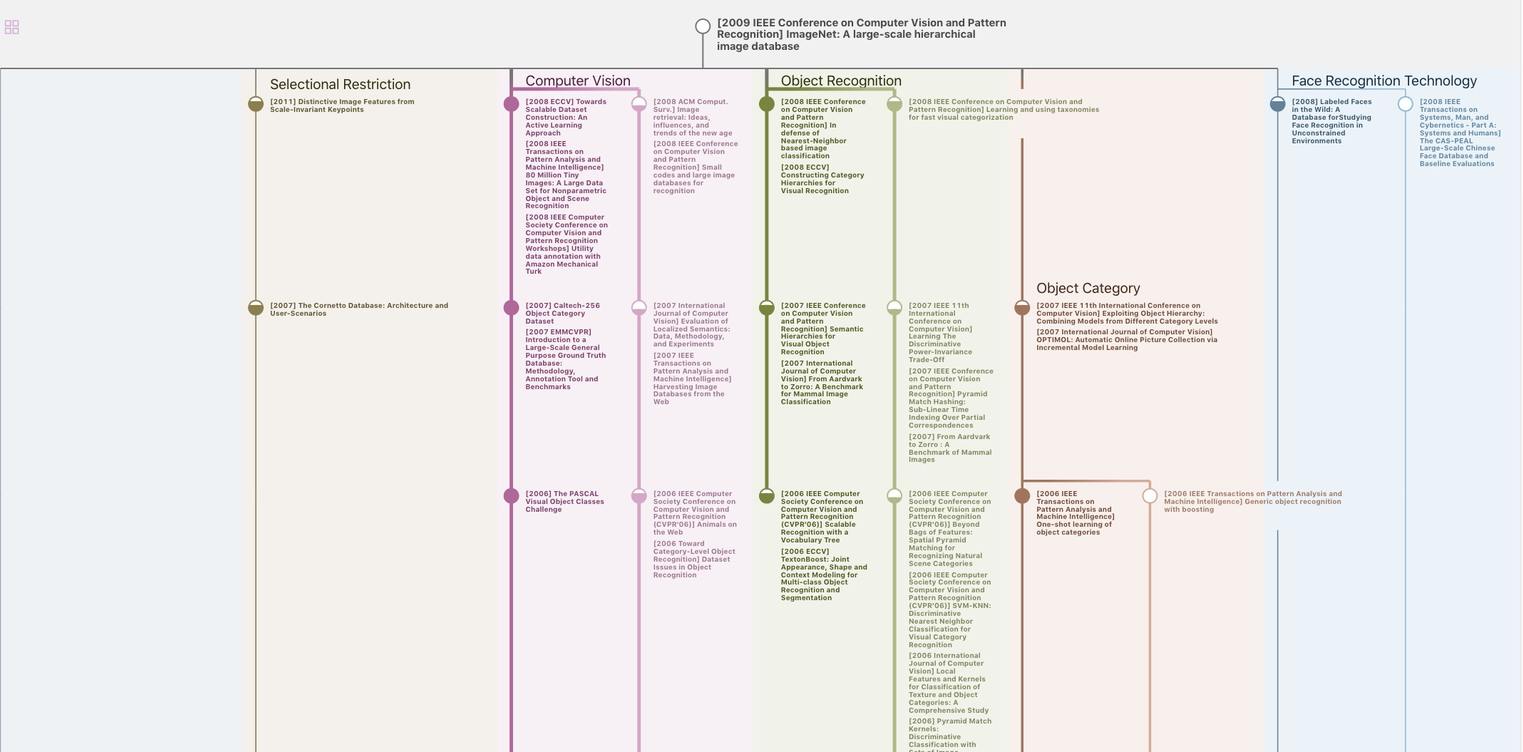
生成溯源树,研究论文发展脉络
Chat Paper
正在生成论文摘要