Fingerprinting Big Data: The Case of KNN Graph Construction
2019 IEEE 35th International Conference on Data Engineering (ICDE)(2019)
Abstract
We propose fingerprinting, a new technique that consists in constructing compact, fast-to-compute and privacy-preserving binary representations of datasets. We illustrate the effectiveness of our approach on the emblematic big data problem of K-Nearest-Neighbor (KNN) graph construction and show that fingerprinting can drastically accelerate a large range of existing KNN algorithms, while efficiently obfuscating the original data, with little to no overhead. Our extensive evaluation of the resulting approach (dubbed GoldFinger) on several realistic datasets shows that our approach delivers speedups of up to 78.9% compared to the use of raw data while only incurring a negligible to moderate loss in terms of KNN quality.
MoreTranslated text
Key words
Motion pictures,Force,Fingerprint recognition,Indexes,Hash functions,Big Data,Acceleration
AI Read Science
Must-Reading Tree
Example
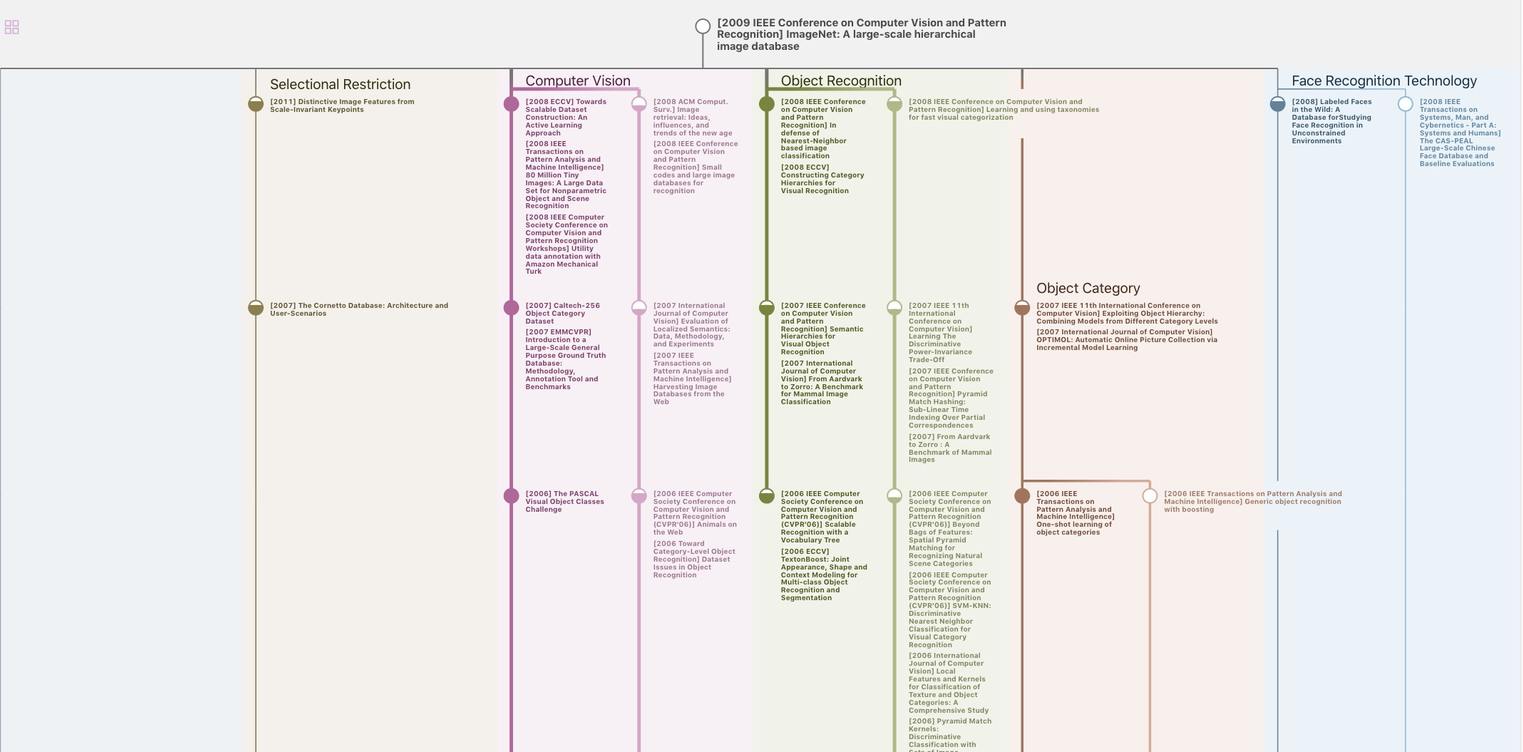
Generate MRT to find the research sequence of this paper
Chat Paper
Summary is being generated by the instructions you defined