Global Optimality Guarantees for Policy Gradient Methods
OPERATIONS RESEARCH(2024)
摘要
Policy gradients methods apply to complex, poorly understood, control problems by performing stochastic gradient descent over a parameterized class of polices. Unfortunately, even for simple control problems solvable by standard dynamic programming techniques, policy gradient algorithms face nonconvex optimization problems and are widely understood to converge only to a stationary point. This work identifies structural properties, shared by several classic control problems, that ensure the policy gradient objective function has no suboptimal stationary points despite being nonconvex. When these conditions are strengthened, this objective satisfies a Polyak-lojasiewicz (gradient dominance) condition that yields convergence rates. We also provide bounds on the optimality gap of any stationary point when some of these conditions are relaxed.
更多查看译文
关键词
reinforcement learning,policy gradient methods,policy iteration,dynamic programming,gradient dominance
AI 理解论文
溯源树
样例
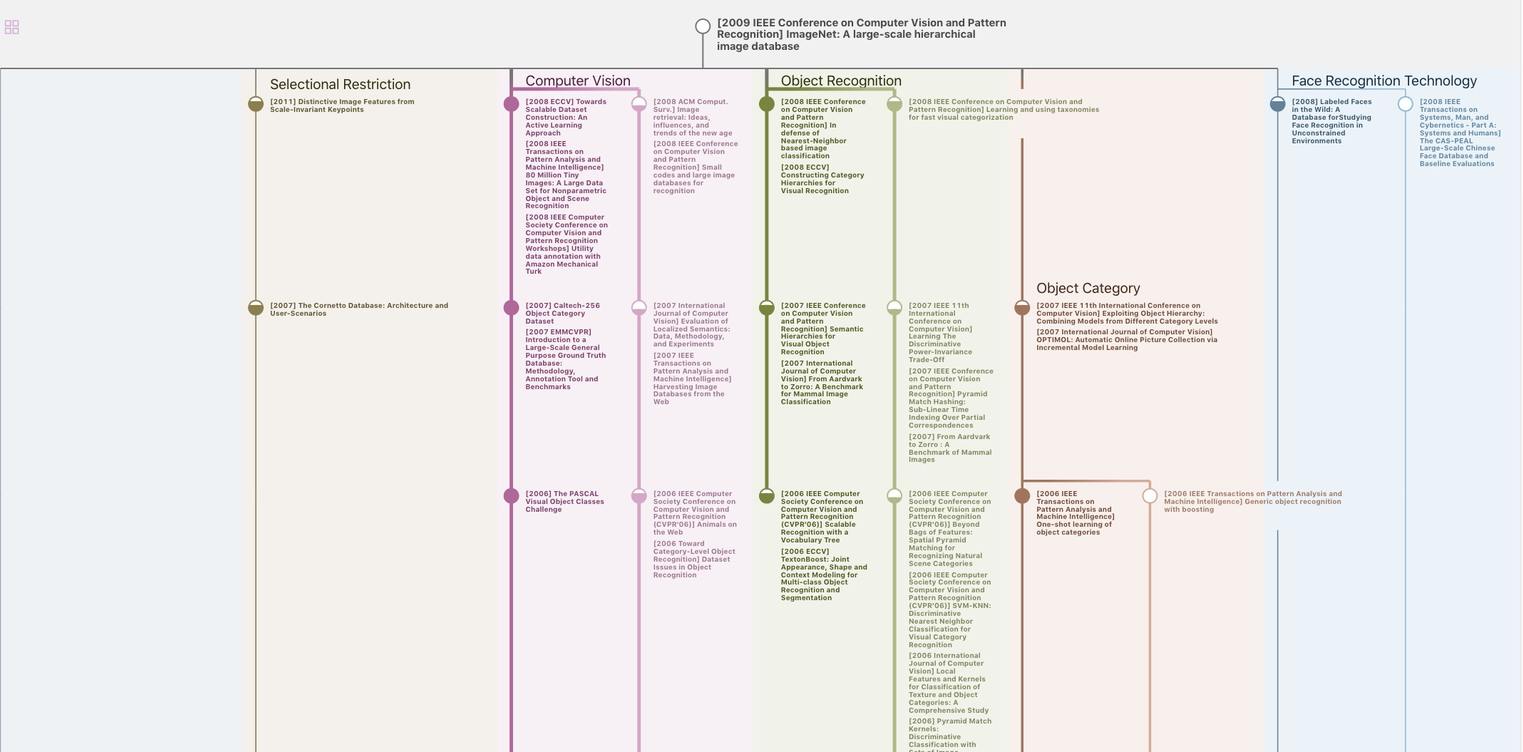
生成溯源树,研究论文发展脉络
Chat Paper
正在生成论文摘要