Training Neural Response Selection for Task-Oriented Dialogue Systems
57TH ANNUAL MEETING OF THE ASSOCIATION FOR COMPUTATIONAL LINGUISTICS (ACL 2019)(2019)
Abstract
Despite their popularity in the chatbot literature, retrieval-based models have had modest impact on task-oriented dialogue systems, with the main obstacle to their application being the low-data regime of most task-oriented dialogue tasks. Inspired by the recent success of pretraining in language modelling, we propose an effective method for deploying response selection in task-oriented dialogue. To train response selection models for task-oriented dialogue tasks, we propose a novel method which: 1) pretrains the response selection model on large general-domain conversational corpora; and then 2) fine-tunes the pretrained model for the target dialogue domain, relying only on the small in-domain dataset to capture the nuances of the given dialogue domain. Our evaluation on six diverse application domains, ranging from e-commerce to banking, demonstrates the effectiveness of the proposed training method.
MoreTranslated text
Key words
dialogue systems,neural response selection,task-oriented
AI Read Science
Must-Reading Tree
Example
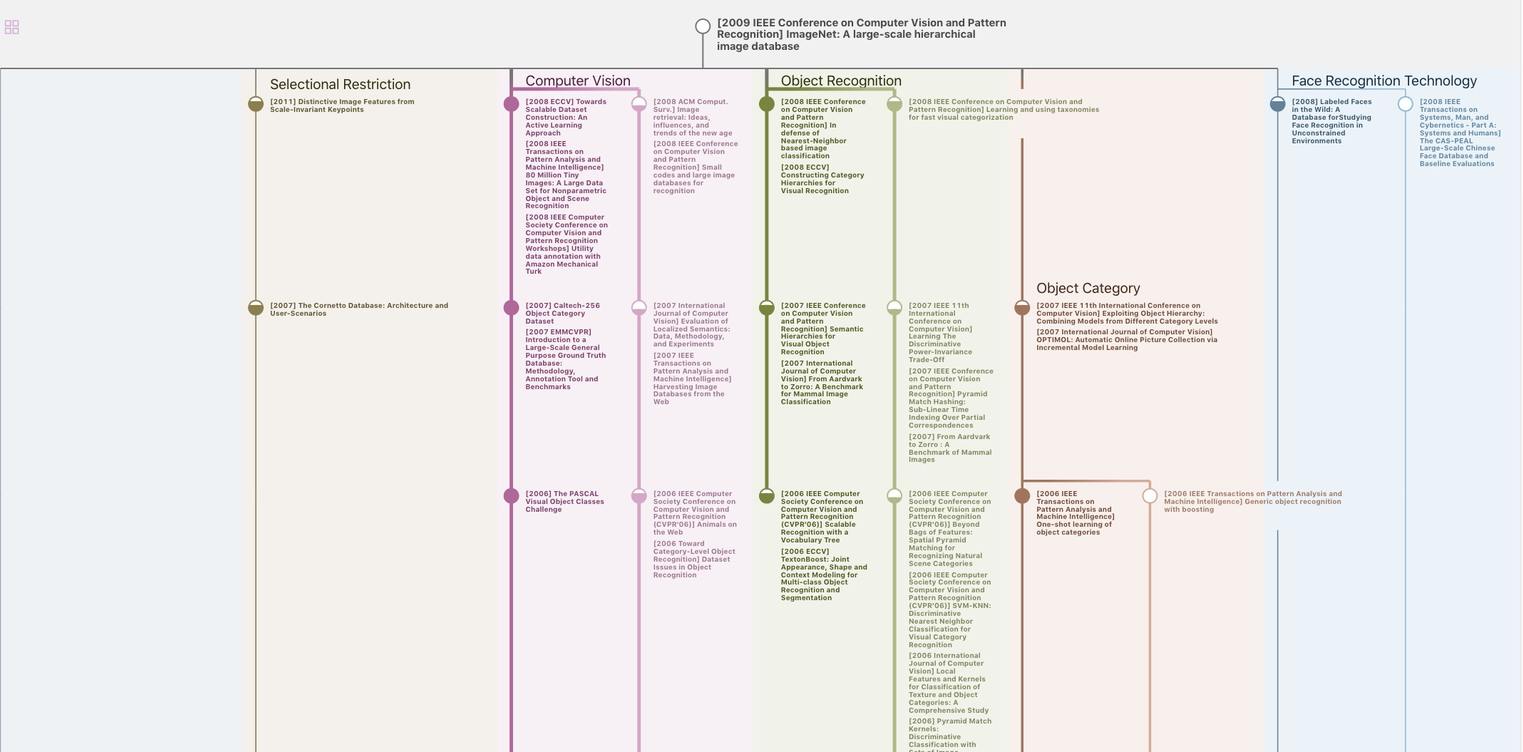
Generate MRT to find the research sequence of this paper
Chat Paper
Summary is being generated by the instructions you defined