SLIND: Identifying stable links in online social networks
Lecture Notes in Computer Science (including subseries Lecture Notes in Artificial Intelligence and Lecture Notes in Bioinformatics)(2018)
摘要
Link stability detection has been an important and long-standing problem in the link prediction domain. However, it is often easily overlooked as being trivial and has not been adequately dealt with in link prediction [1]. In this demo, we introduce an innovative link stability detection system, called SLIND (Stable LINk Detection), that adopts a Multi-Variate Vector Autoregression analysis (MVVA) approach using link dynamics to establish stability confidence scores of links within a clique of nodes in online social networks (OSN) to improve detection accuracy and the representation of stable links. SLIND is also able to determine stable links through the use of partial feature information and potentially scales well to much larger datasets with very little accuracy to performance trade-offs using random walk Monte-Carlo estimates. © Springer International Publishing AG, part of Springer Nature 2018.
更多查看译文
关键词
Link stability, Graph theory, Online social networks, Hamiltonian Monte Carlo (HMC)
AI 理解论文
溯源树
样例
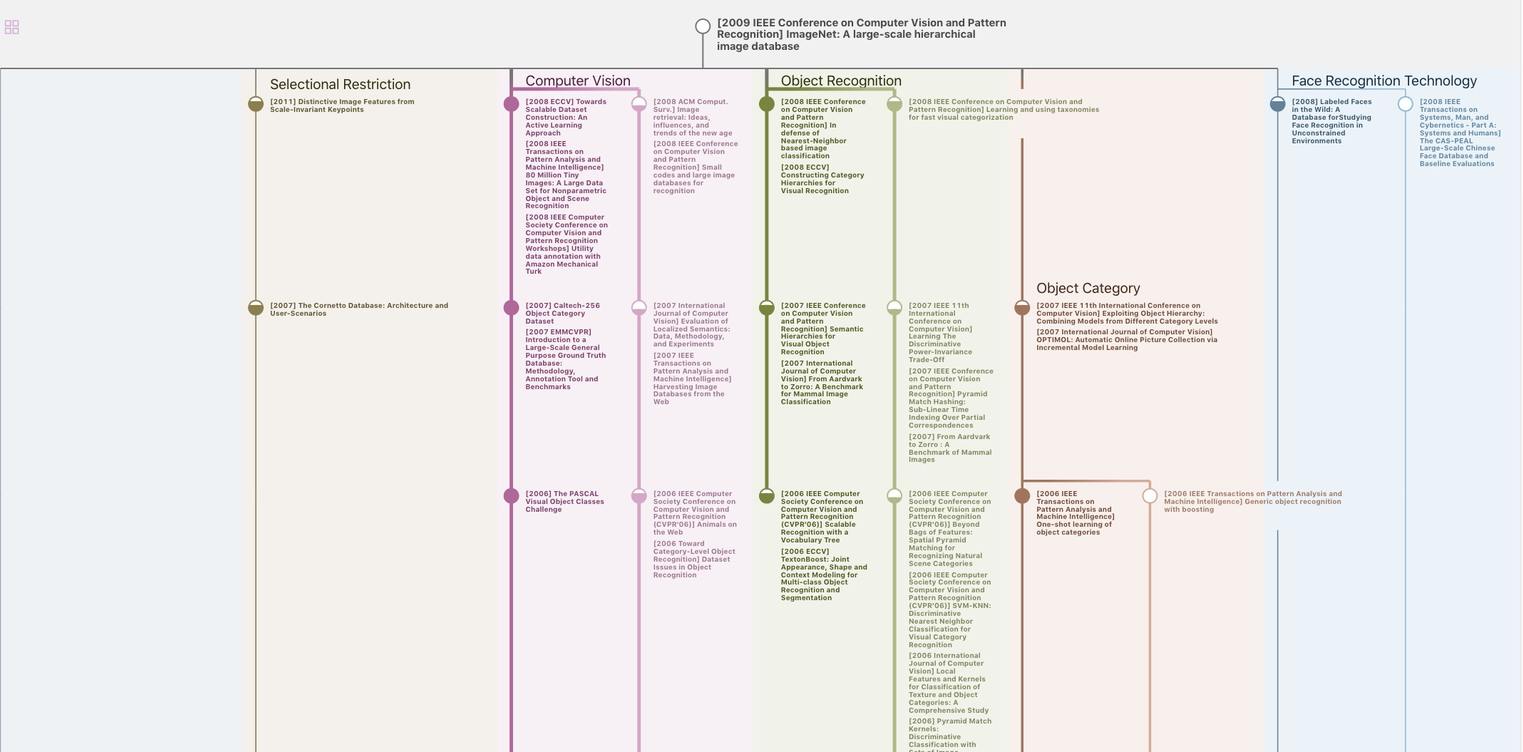
生成溯源树,研究论文发展脉络
Chat Paper
正在生成论文摘要