Transformed central quantile subspace
STATISTICS(2021)
摘要
Quantile regression (QR) is a well-established method of tail analysis. Application of QR can become very challenging when dealing with high-dimensional data, thus requiring dimension reduction techniques. While the current literature on these techniques focuses on extracting linear combinations of the predictor variables that contain all the information about the conditional quantile, non-linear features can potentially achieve greater dimension reduction. We, therefore, present the first application of transformed dimension reduction for conditional quantiles, which serves as an intermediate step between linear and nonlinear dimension reduction. The idea is to transform the predictors monotonically and then look for low-dimensional projections by applying linear dimension reduction techniques. The performance of the proposed methodology is demonstrated through simulation examples and a real data application.
更多查看译文
关键词
Dimension reduction,quantile regression,transformed predictors,Value-at-Risk
AI 理解论文
溯源树
样例
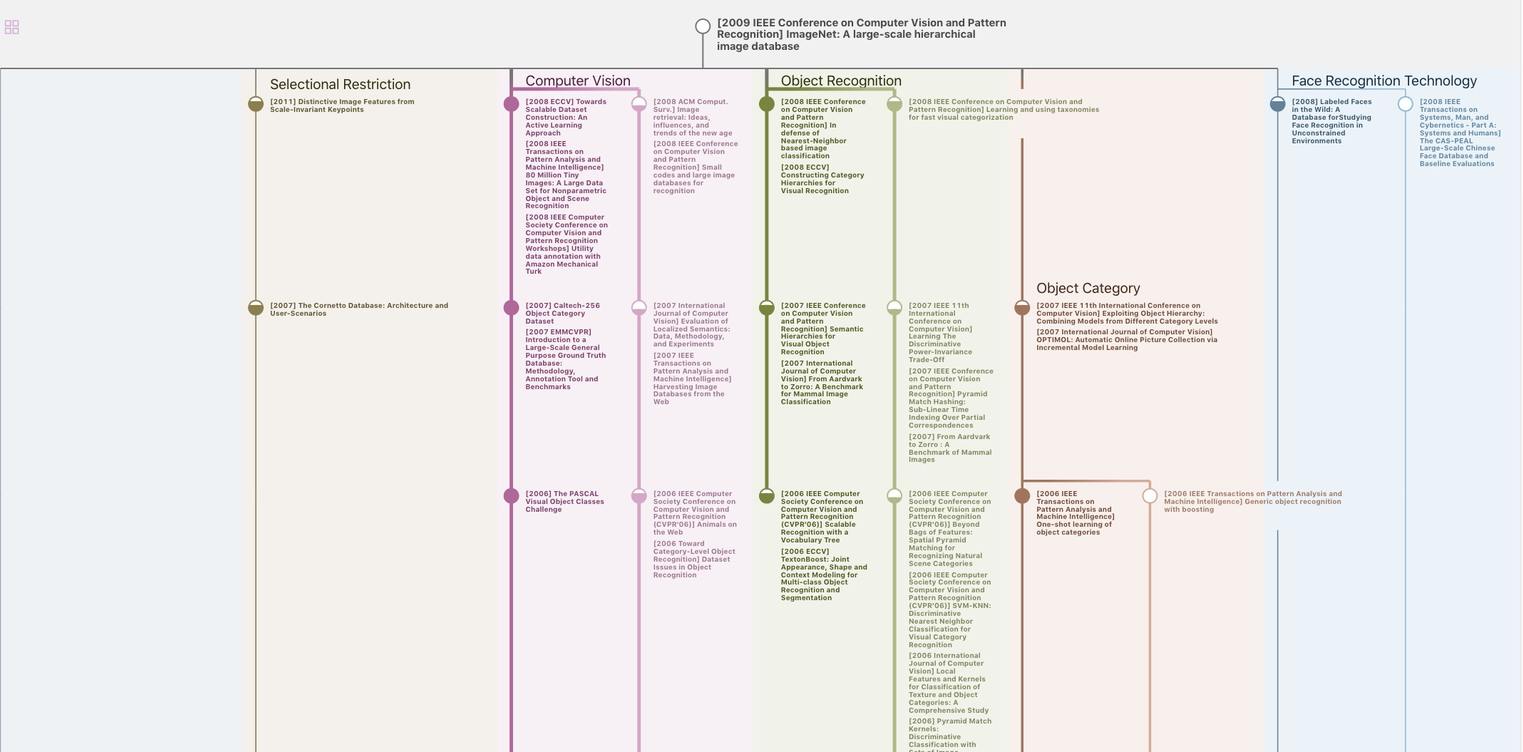
生成溯源树,研究论文发展脉络
Chat Paper
正在生成论文摘要