Incremental Few-Shot Learning for Pedestrian Attribute Recognition
PROCEEDINGS OF THE TWENTY-EIGHTH INTERNATIONAL JOINT CONFERENCE ON ARTIFICIAL INTELLIGENCE(2019)
摘要
Pedestrian attribute recognition has received increasing attention due to its important role in video surveillance applications. However, most existing methods are designed for a fixed set of attributes. They are unable to handle the incremental few-shot learning scenario, i.e. adapting a well-trained model to newly added attributes with scarce data, which commonly exists in the real world. In this work, we present a meta learning based method to address this issue. The core of our framework is a meta architecture capable of disentangling multiple attribute information and generalizing rapidly to new coming attributes. By conducting extensive experiments on the benchmark dataset PETA and RAP under the incremental few-shot setting, we show that our method is able to perform the task with competitive performances and low resource requirements.
更多查看译文
关键词
Multiple Object Tracking,Object Tracking,Visual Tracking,Action Recognition,Motion Detection
AI 理解论文
溯源树
样例
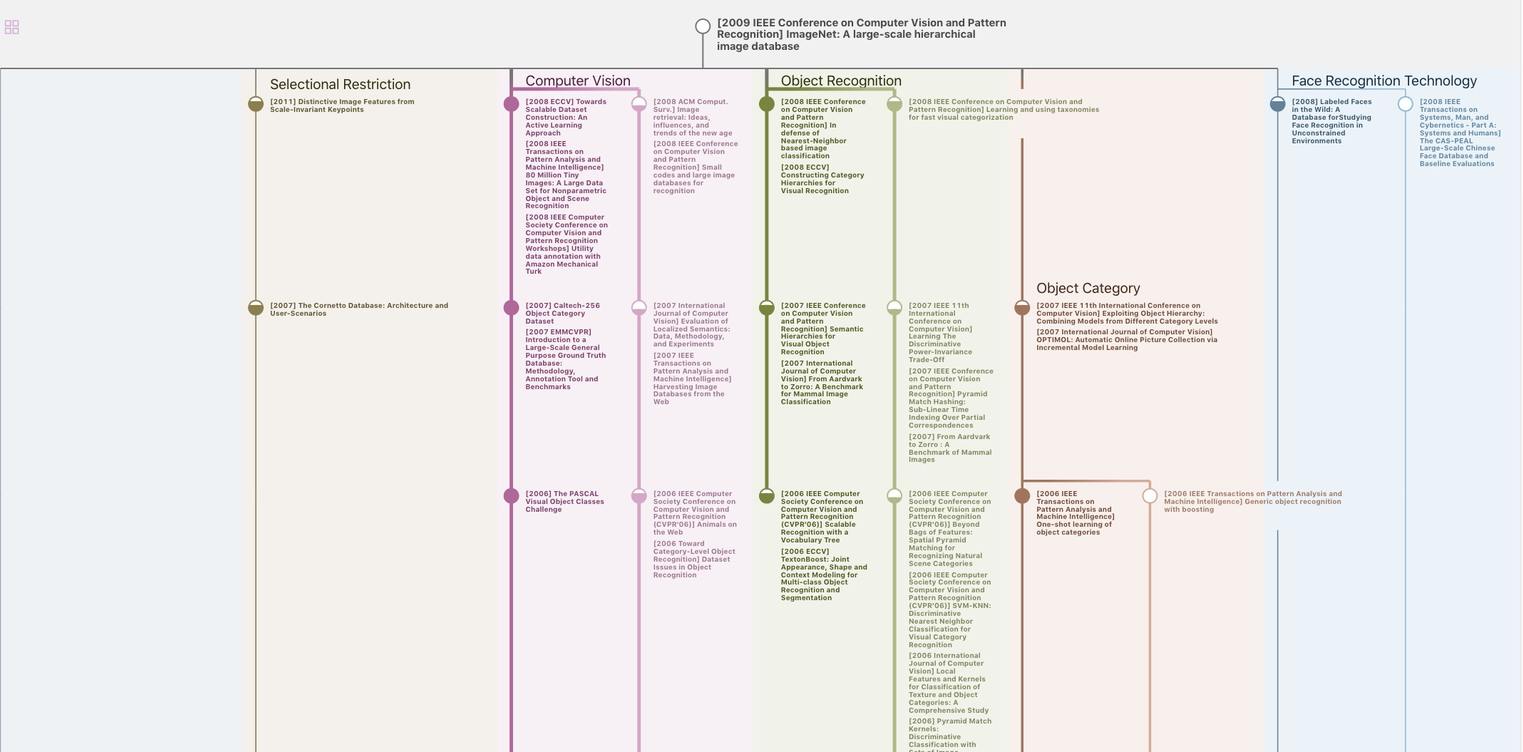
生成溯源树,研究论文发展脉络
Chat Paper
正在生成论文摘要