Skewed target range strategy for multiperiod portfolio optimization using a two-stage least squares Monte Carlo method
JOURNAL OF COMPUTATIONAL FINANCE(2019)
摘要
In this paper, we propose a novel investment strategy for portfolio optimization problems. The proposed strategy maximizes the expected portfolio value bounded within a targeted range, composed of a conservative lower target representing a need for capital protection and a desired upper target representing an investment goal. This strategy favorably shapes the entire probability distribution of returns, as it simultaneously seeks a desired expected return, cuts off downside risk and implicitly caps volatility and higher moments. To illustrate the effectiveness of this investment strategy, we study a multiperiod portfolio optimization problem with transaction costs and develop a two-stage regression approach that improves the classical least squares Monte Carlo (LSMC) algorithm when dealing with difficult payoffs, such as highly concave, abruptly changing or discontinuous functions. Our numerical results show substantial improvements over the classical LSMC algorithm for both the constant relative risk-aversion (CRRA) utility approach and the proposed skewed target range strategy (STRS). Our numerical results illustrate the ability of the STRS to contain the portfolio value within the targeted range. When compared with the CRRA utility approach, the STRS achieves a similar mean-variance efficient frontier while delivering a better downside risk-return trade-off.
更多查看译文
关键词
target-based portfolio optimization,alternative performance measure,multiperiod portfolio optimization,least squares Monte Carlo,two-stage regression
AI 理解论文
溯源树
样例
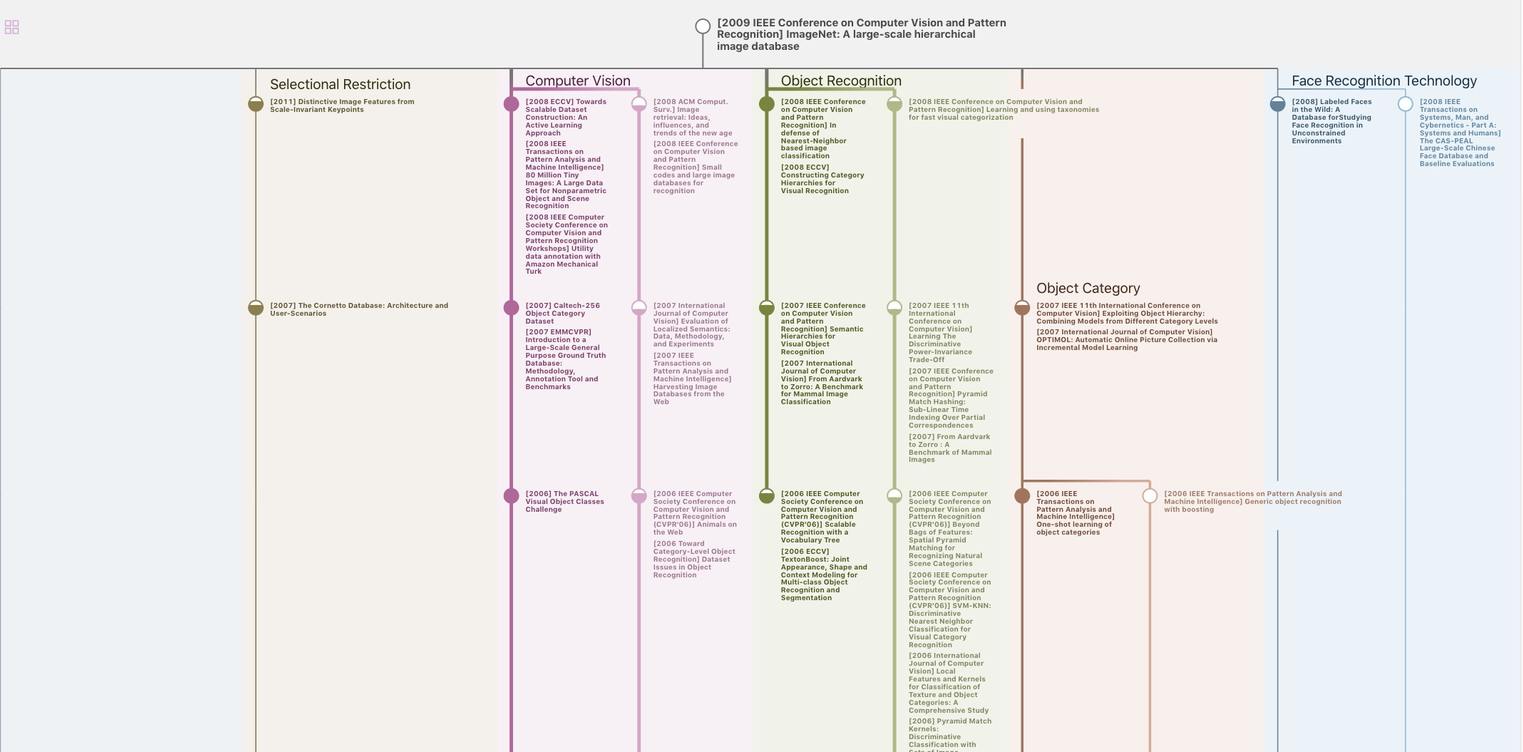
生成溯源树,研究论文发展脉络
Chat Paper
正在生成论文摘要