Development and application of a high throughput natural language processing architecture to convert all clinical documents in a clinical data warehouse into standardized medical vocabularies.
JOURNAL OF THE AMERICAN MEDICAL INFORMATICS ASSOCIATION(2019)
摘要
Objective: Natural language processing (NLP) engines such as the clinical Text Analysis and Knowledge Extraction System are a solution for processing notes for research, but optimizing their performance for a clinical data warehouse remains a challenge. We aim to develop a high throughput NLP architecture using the clinical Text Analysis and Knowledge Extraction System and present a predictive model use case. Materials and Methods: The CDW was comprised of 1 103 038 patients across 10 years. The architecture was constructed using the Hadoop data repository for source data and 3 large-scale symmetric processing servers for NLP. Each named entity mention in a clinical document was mapped to the Unified Medical Language System concept unique identifier (CUI). Results: The NLP architecture processed 83 867 802 clinical documents in 13.33 days and produced 37 721 886 606 CUIs across 8 standardized medical vocabularies. Performance of the architecture exceeded 500 000 documents per hour across 30 parallel instances of the clinical Text Analysis and Knowledge Extraction System including 10 instances dedicated to documents greater than 20 000 bytes. In a use-case example for predicting 30-day hospital readmission, a CUI-based model had similar discrimination to n-grams with an area under the curve receiver operating characteristic of 0.75 (95% CI, 0.74-0.76). Discussion and Conclusion: Our health system's high throughput NLP architecture may serve as a benchmark for large-scale clinical research using a CUI-based approach.
更多查看译文
关键词
natural language processing,unstructured data,clinical text and knowledge extraction system,data architecture,unified medical language system
AI 理解论文
溯源树
样例
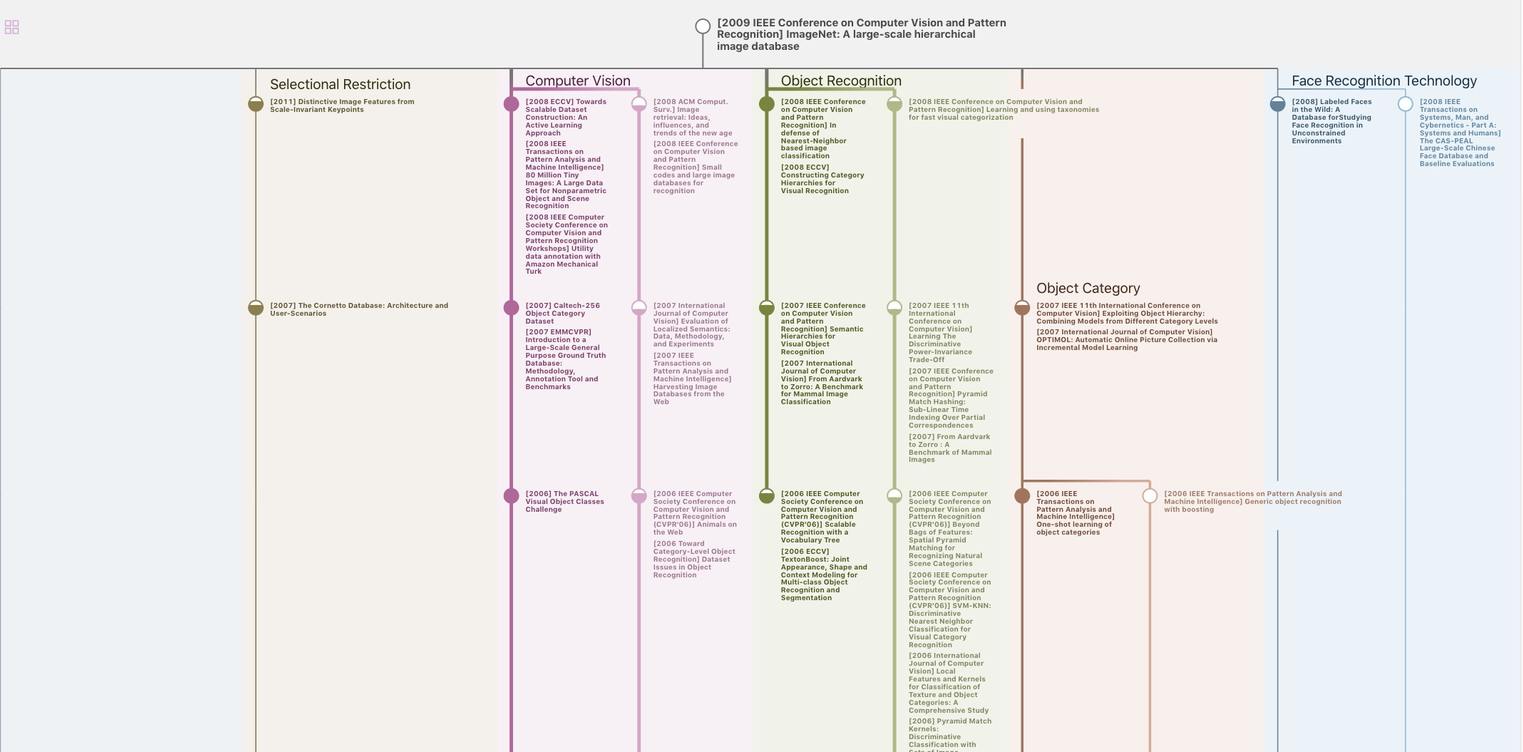
生成溯源树,研究论文发展脉络
Chat Paper
正在生成论文摘要