Quantifying the Alignment of Graph and Features in Deep Learning
IEEE TRANSACTIONS ON NEURAL NETWORKS AND LEARNING SYSTEMS(2022)
摘要
We show that the classification performance of graph convolutional networks (GCNs) is related to the alignment between features, graph, and ground truth, which we quantify using a subspace alignment measure (SAM) corresponding to the Frobenius norm of the matrix of pairwise chordal distances between three subspaces associated with features, graph, and ground truth. The proposed measure is based on the principal angles between subspaces and has both spectral and geometrical interpretations. We showcase the relationship between the SAM and the classification performance through the study of limiting cases of GCNs and systematic randomizations of both features and graph structure applied to a constructive example and several examples of citation networks of different origins. The analysis also reveals the relative importance of the graph and features for classification purposes.
更多查看译文
关键词
Symmetric matrices,Deep learning,Task analysis,Convolution,Training,Nonhomogeneous media,Learning systems,Data alignment,deep learning,graph convolutional networks (GCNs),graph subspaces,principal angles
AI 理解论文
溯源树
样例
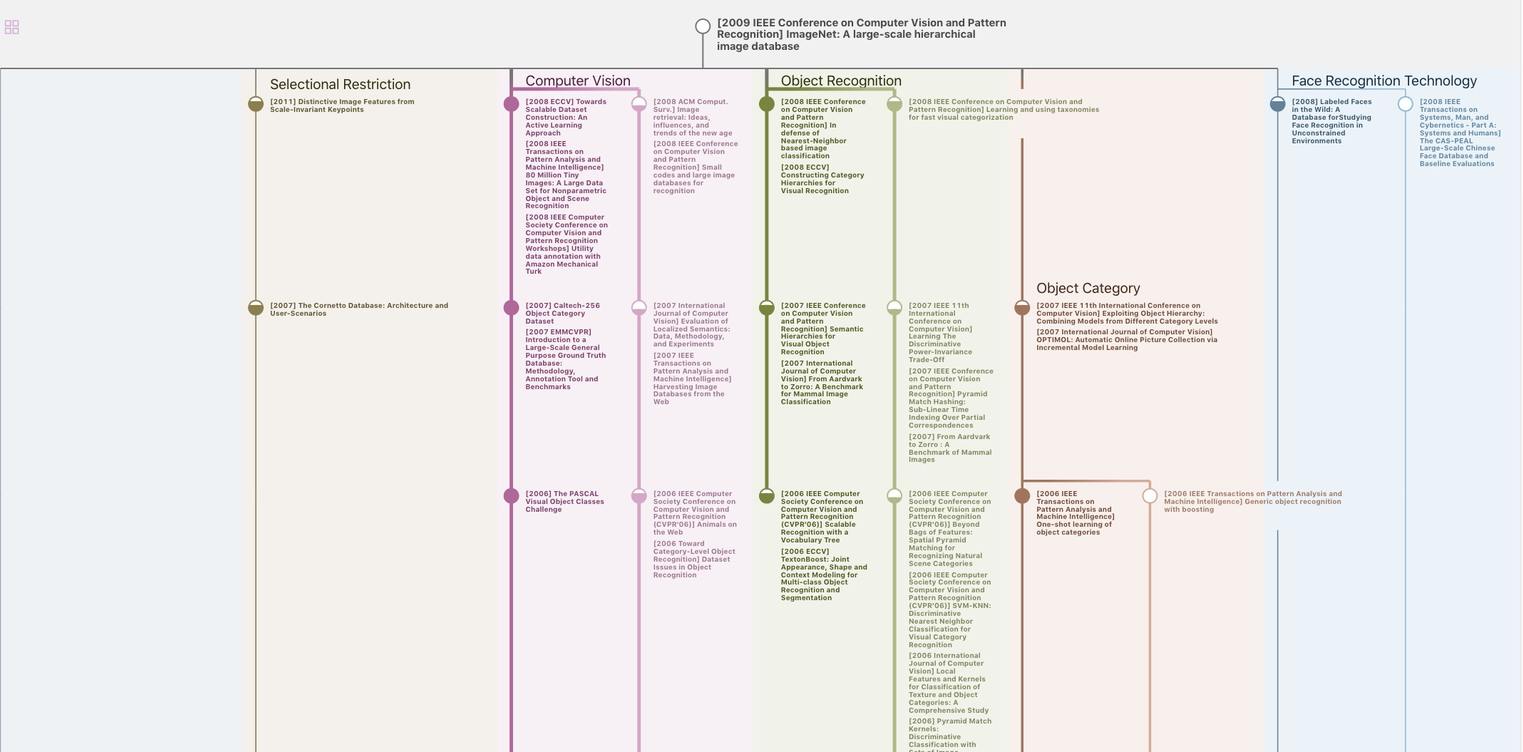
生成溯源树,研究论文发展脉络
Chat Paper
正在生成论文摘要