A deep learning approach for robust head pose independent eye movements recognition from videos
Proceedings of the 11th ACM Symposium on Eye Tracking Research & Applications(2019)
摘要
Recognizing eye movements is important for gaze behavior understanding like in human communication analysis (human-human or robot interactions) or for diagnosis (medical, reading impairments). In this paper, we address this task using remote RGB-D sensors to analyze people behaving in natural conditions. This is very challenging given that such sensors have a normal sampling rate of 30 Hz and provide low-resolution eye images (typically 36×60 pixels), and natural scenarios introduce many variabilities in illumination, shadows, head pose, and dynamics. Hence gaze signals one can extract in these conditions have lower precision compared to dedicated IR eye trackers, rendering previous methods less appropriate for the task. To tackle these challenges, we propose a deep learning method that directly processes the eye image video streams to classify them into fixation, saccade, and blink classes, and allows to distinguish irrelevant noise (illumination, low-resolution artifact, inaccurate eye alignment, difficult eye shapes) from true eye motion signals. Experiments on natural 4-party interactions demonstrate the benefit of our approach compared to previous methods, including deep learning models applied to gaze outputs.
更多查看译文
关键词
blink, convolutional neural network, eye movements, remote sensors, saccade, video processing
AI 理解论文
溯源树
样例
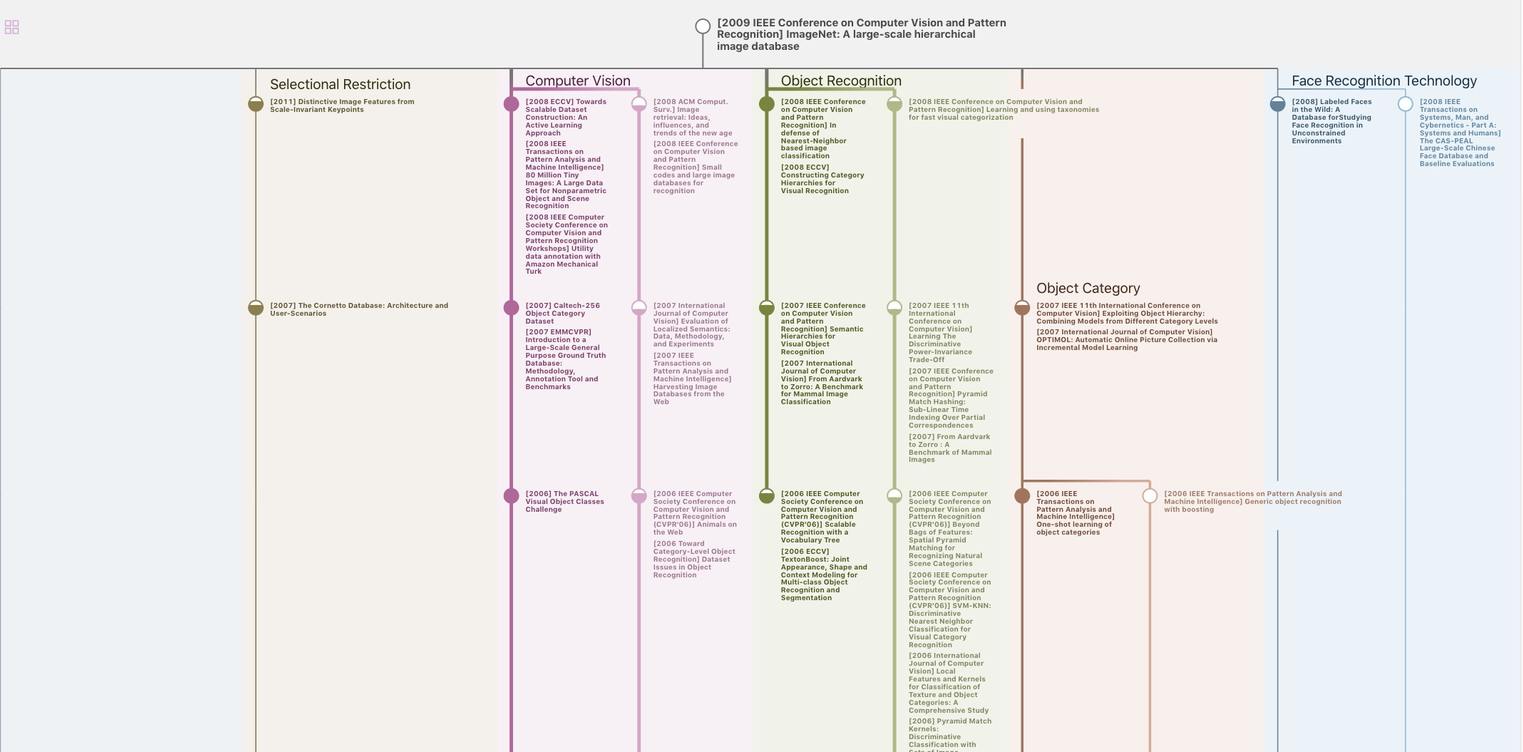
生成溯源树,研究论文发展脉络
Chat Paper
正在生成论文摘要