Exploring simple neural network architectures for eye movement classification
Proceedings of the 11th ACM Symposium on Eye Tracking Research & Applications(2019)
摘要
Analysis of eye-gaze is a critical tool for studying human-computer interaction and visualization. Yet eye tracking systems only report eye-gaze on the scene by producing large volumes of coordinate time series data. To be able to use this data, we must first extract salient events such as eye fixations, saccades, and post-saccadic oscillations (PSO). Manually extracting these events is time-consuming, labor-intensive and subject to variability. In this paper, we present and evaluate simple and fast automatic solutions for eye-gaze analysis based on supervised learning. Similar to some recent studies, we developed different simple neural networks demonstrating that feature learning produces superior results in identifying events from sequences of gaze coordinates. We do not apply any ad-hoc post-processing, thus creating a fully automated end-to-end algorithms that perform as good as current state-of-the-art architectures. Once trained they are fast enough to be run in a near real time setting.
更多查看译文
关键词
deep learning, event detection, eye movement, machine learning
AI 理解论文
溯源树
样例
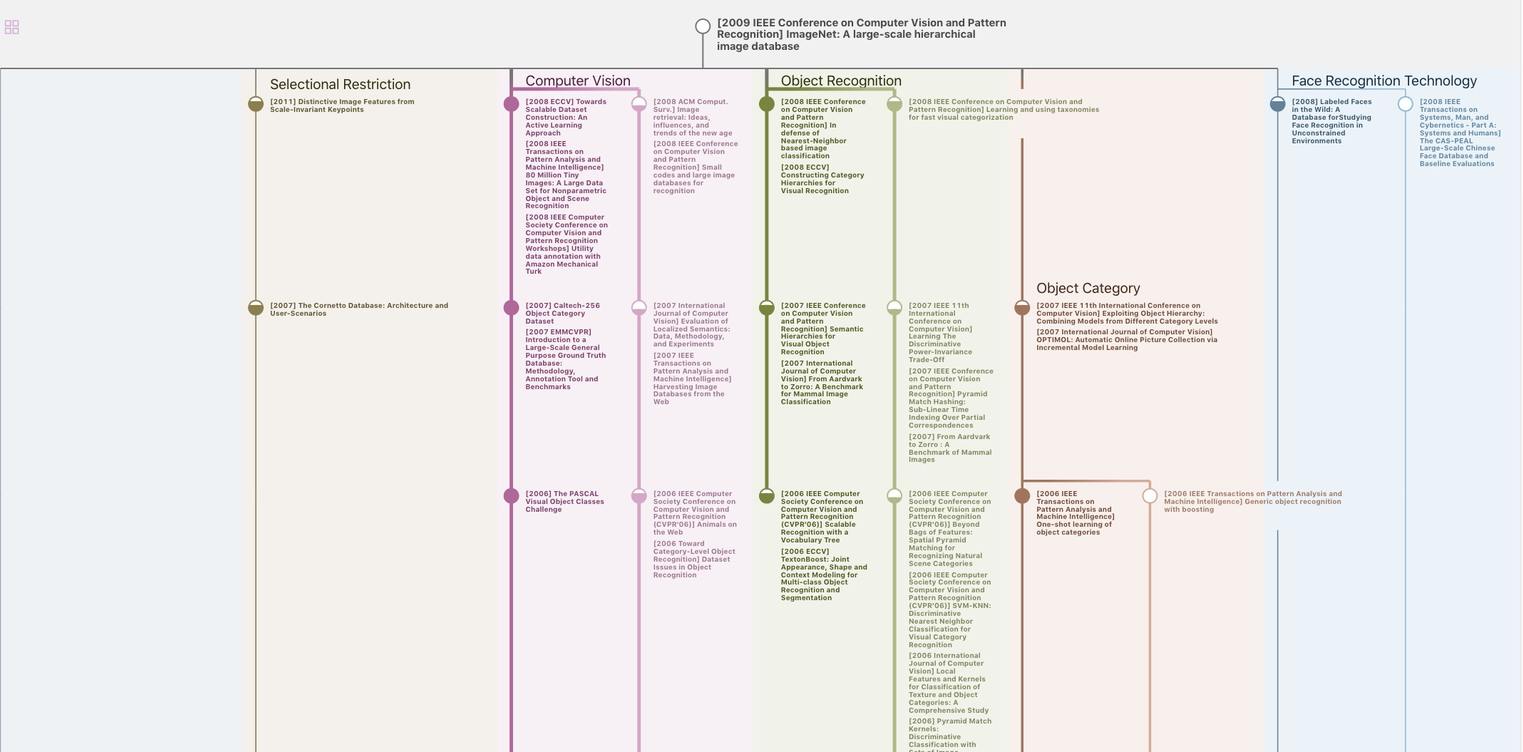
生成溯源树,研究论文发展脉络
Chat Paper
正在生成论文摘要