General solutions for nonlinear differential equations: a rule-based self-learning approach using deep reinforcement learning
Computational Mechanics(2019)
摘要
A universal rule-based self-learning approach using deep reinforcement learning (DRL) is proposed for the first time to solve nonlinear ordinary differential equations and partial differential equations. The solver consists of a deep neural network-structured actor that outputs candidate solutions, and a critic derived only from physical rules (governing equations and boundary and initial conditions). Solutions in discretized time are treated as multiple tasks sharing the same governing equation, and the current step parameters provide an ideal initialization for the next owing to the temporal continuity of the solutions, which shows a transfer learning characteristic and indicates that the DRL solver has captured the intrinsic nature of the equation. The approach is verified through solving the Schrödinger, Navier–Stokes, Burgers’, Van der Pol, and Lorenz equations and an equation of motion. The results indicate that the approach gives solutions with high accuracy, and the solution process promises to get faster.
更多查看译文
关键词
Nonlinear differential equations, Rule-based solving method, Deep reinforcement learning, General solution, Transfer learning
AI 理解论文
溯源树
样例
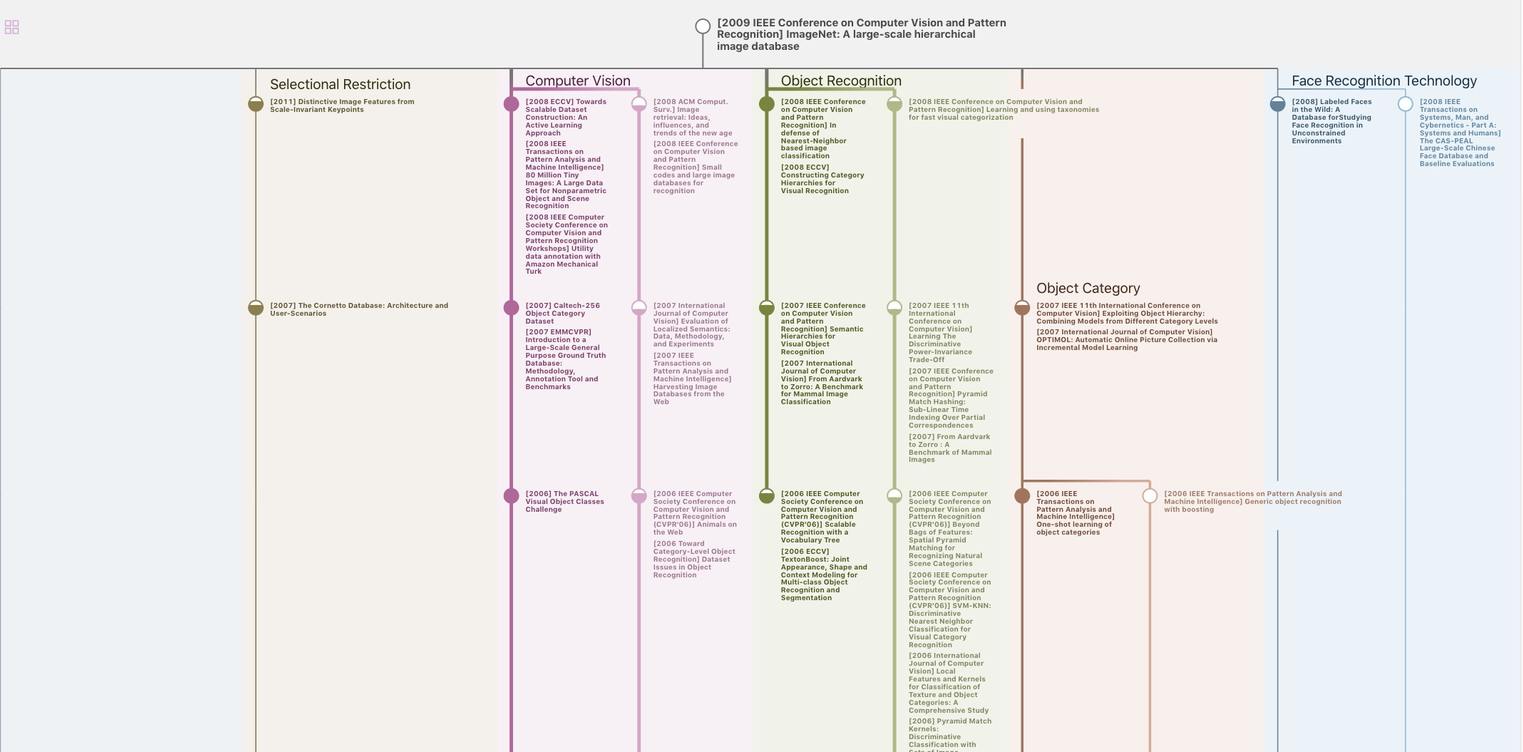
生成溯源树,研究论文发展脉络
Chat Paper
正在生成论文摘要