On a scalable problem transformation method for multi-label learning.
arXiv: Information Retrieval(2019)
摘要
Binary relevance is a simple approach to solve multi-label learning problems where an independent binary classifier is built per each label. A common challenge with this in real-world applications is that the label space can be very large, making it difficult to use binary relevance to larger scale problems. In this paper, we propose a scalable alternative to this, via transforming the multi-label problem into a single binary classification. We experiment with a few variations of our method and show that our method achieves higher precision than binary relevance and faster execution times on a top-K recommender system task.
更多查看译文
AI 理解论文
溯源树
样例
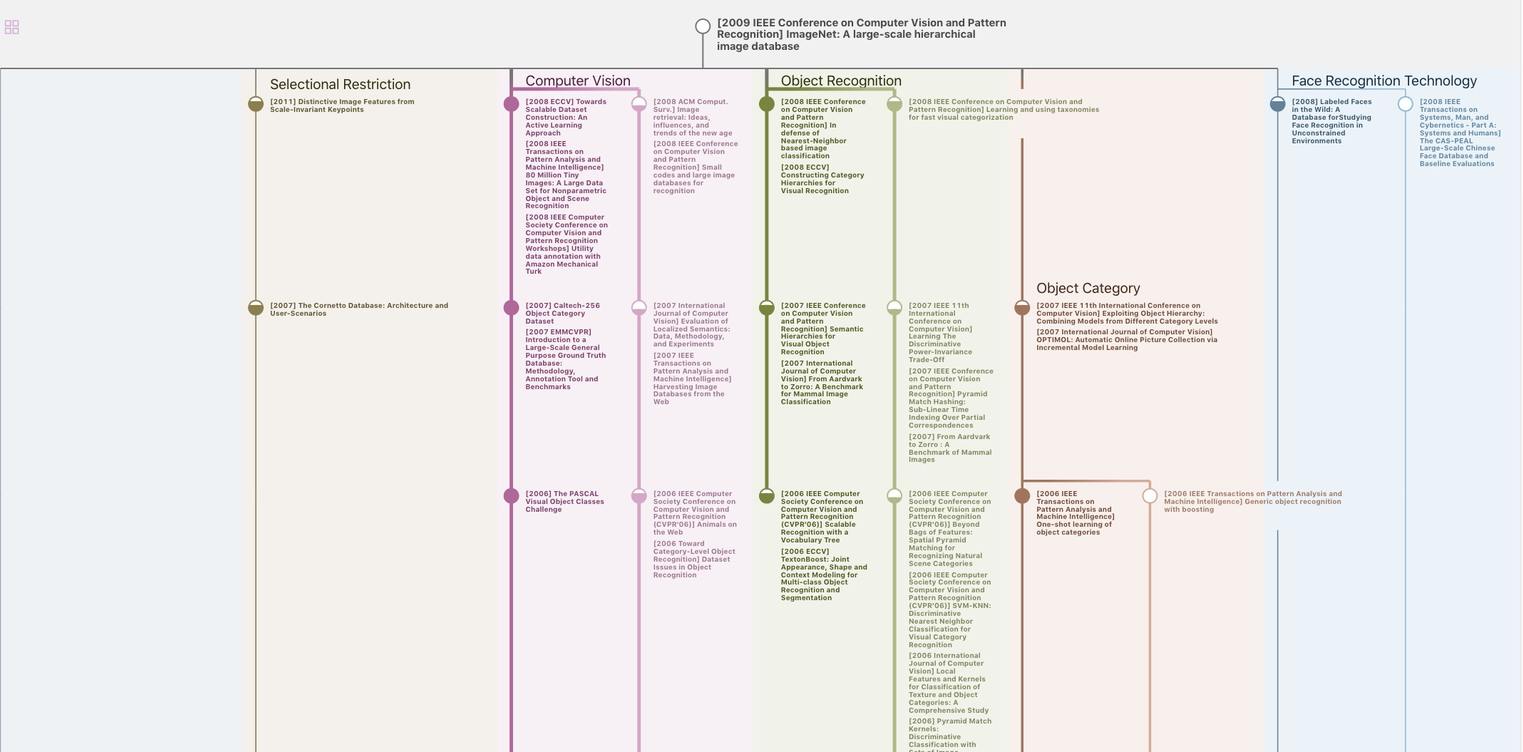
生成溯源树,研究论文发展脉络
Chat Paper
正在生成论文摘要