Adaptive Visual Interaction Based Multi-Target Future State Prediction For Autonomous Driving Vehicles
IEEE Transactions on Vehicular Technology(2019)
摘要
Predicting the state of dynamic objects in a real traffic environment is a key issue in autonomous driving vehicles. Various approaches have been proposed to learn the dynamics from visual observations with static background. However, minimal research has been conducted in a real traffic environment due to the complicated and changeable scenes. This paper proposes an adaptive multi-target future state prediction (position/velocity) method under autonomous driving conditions. In particular, an adaptive visual interaction method and control mechanism are introduced to overcome the change in the number of objects in continuous driving frames. In addition, a two-stream architecture with stage-wise learning is utilized for accurate object state prediction by simultaneously complementing spatial and temporal information. Experiments on two public challenging datasets, namely Udacity (CrowdAI) and Udacity (Autti), demonstrate the effectiveness of the proposed method on multi-target dynamic state prediction in a real traffic environment.
更多查看译文
关键词
Visualization,Vehicle dynamics,Task analysis,Autonomous vehicles,Automobiles,Dynamics,Streaming media
AI 理解论文
溯源树
样例
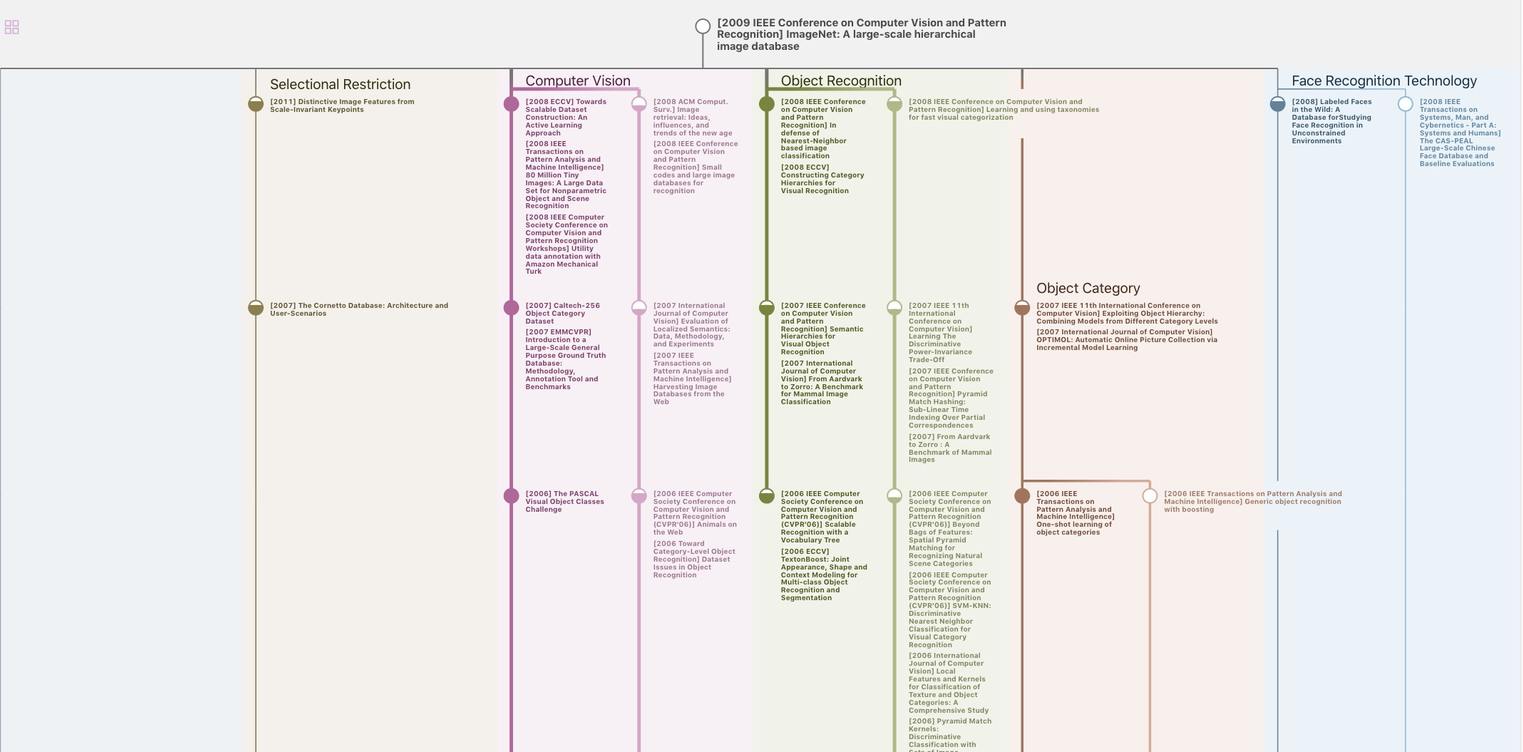
生成溯源树,研究论文发展脉络
Chat Paper
正在生成论文摘要