Learning Force-Relevant Skills from Human Demonstration.
COMPLEXITY(2019)
Abstract
Many human manipulation skills are force relevant, such as opening a bottle cap and assembling furniture. However, it is still a difficult task to endow a robot with these skills, which largely is due to the complexity of the representation and planning of these skills. This paper presents a learning-based approach of transferring force-relevant skills from human demonstration to a robot. First, the force-relevant skill is encapsulated as a statistical model where the key parameters are learned from the demonstrated data (motion, force). Second, based on the learned skill model, a task planner is devised which specifies the motion and/or the force profile for a given manipulation task. Finally, the learned skill model is further integrated with an adaptive controller that offers task-consistent force adaptation during online executions. The effectiveness of the proposed approach is validated with two experiments, i.e., an object polishing task and a peg-in-hole assembly.
MoreTranslated text
Key words
human demonstration,skills,force-relevant
AI Read Science
Must-Reading Tree
Example
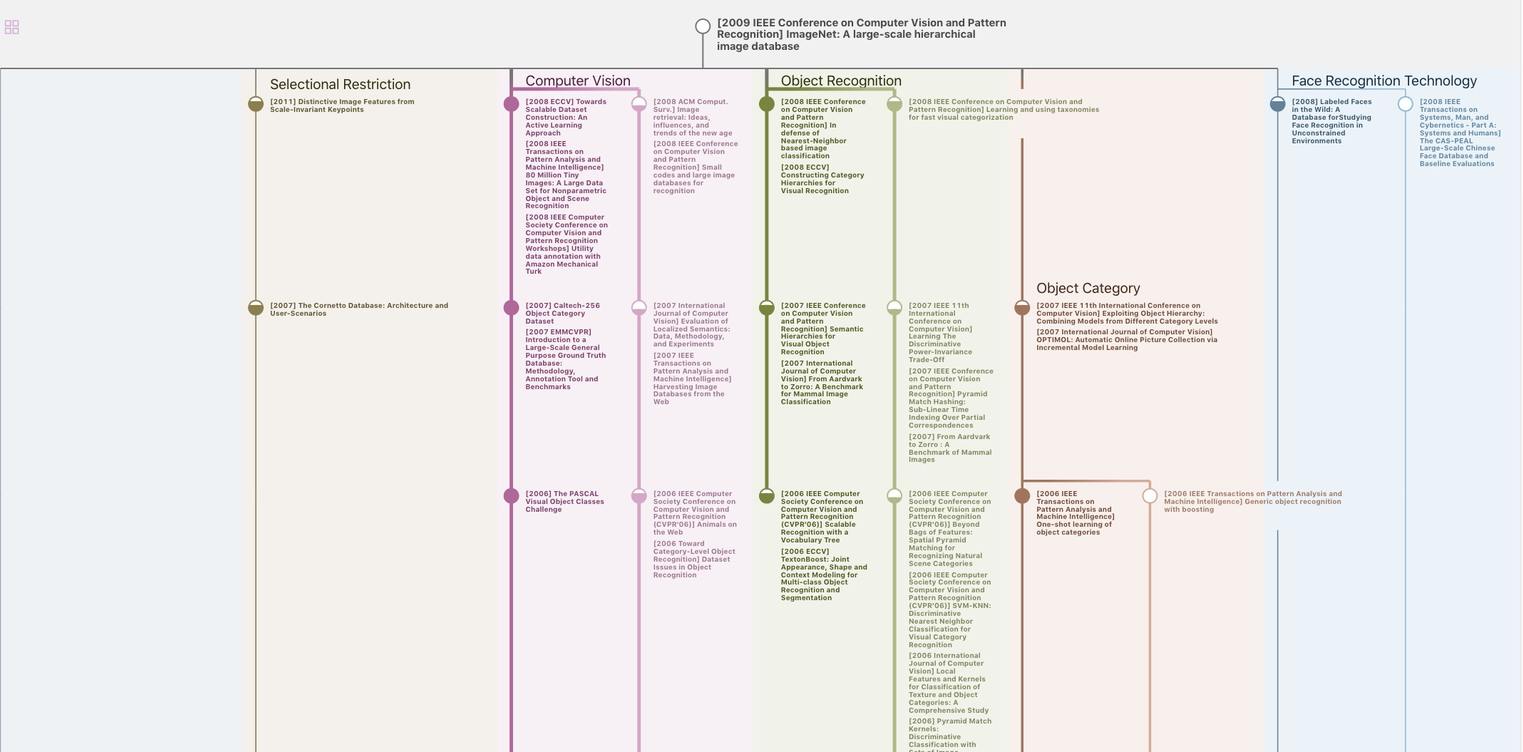
Generate MRT to find the research sequence of this paper
Chat Paper
Summary is being generated by the instructions you defined