Differential Description Length for Hyperparameter Selection in Machine Learning.
arXiv: Learning(2019)
摘要
This paper introduces a new method for model selection and more generally hyperparameter selection in machine learning. The paper first proves a relationship between generalization error and a difference of description lengths of the training data; we call this difference differential description length (DDL). This allows prediction of generalization error from the training data emph{alone} by performing encoding of the training data. This can now be used for model selection by choosing the model that has the smallest predicted generalization error. We show how this encoding can be done for linear regression and neural networks. We provide experiments showing that this leads to smaller generalization error than cross-validation and traditional MDL and Bayes methods.
更多查看译文
关键词
hyperparameter selection,machine learning,length,description
AI 理解论文
溯源树
样例
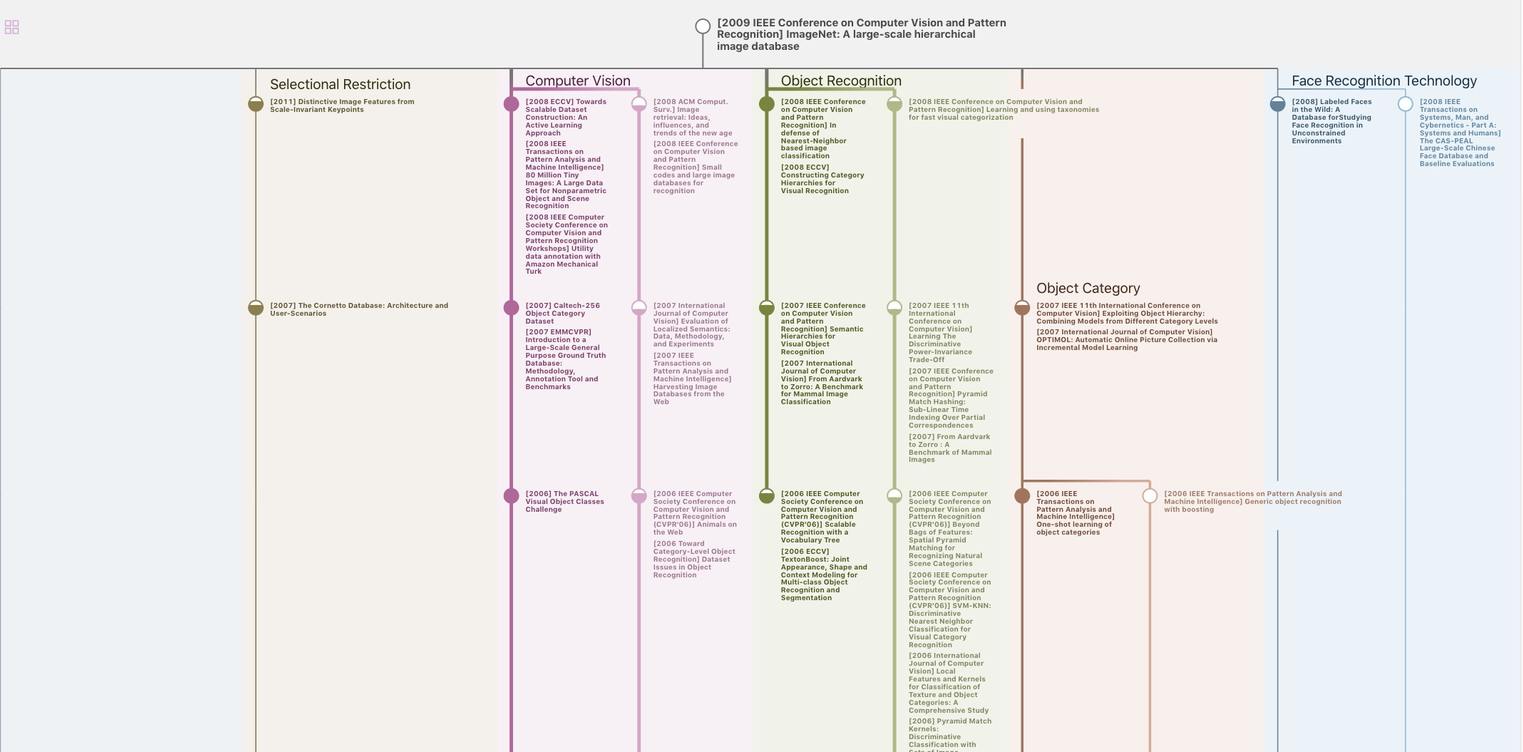
生成溯源树,研究论文发展脉络
Chat Paper
正在生成论文摘要