An Annotation Saved Is An Annotation Earned: Using Fully Synthetic Training For Object Detection
arXiv: Computer Vision and Pattern Recognition(2019)
摘要
Deep learning methods typically require vast amounts of training data to reach their full potential. While some publicly available datasets exists, domain specific data always needs to be collected and manually labeled, an expensive, time consuming and error prone process. Training with synthetic data is therefore very lucrative, as dataset creation and labeling comes for free. We propose a novel method for creating purely synthetic training data for object detection. We leverage a large dataset of 3D background models and densely render them using full domain randomization. This yields background images with realistic shapes and texture on top of which we render the objects of interest. During training, the data generation process follows a curriculum strategy guaranteeing that all foreground models are presented to the network equally under all possible poses and conditions with increasing complexity. As a result, we entirely control the underlying statistics and we create optimal training samples at every stage of training. Using a challenging evaluation dataset with 64 retail objects, we demonstrate that our approach enables the training of detectors that compete favorably with models trained on real data while being at least two orders of magnitude more time and cost effective with respect to data annotation. Finally, our approach performs significantly better on the YCB-Video Dataset [34] than DOPE [32] - a state-of-the-art method in learning from synthetic data.
更多查看译文
关键词
Synthetic Data,Object Detection,Deep Learning
AI 理解论文
溯源树
样例
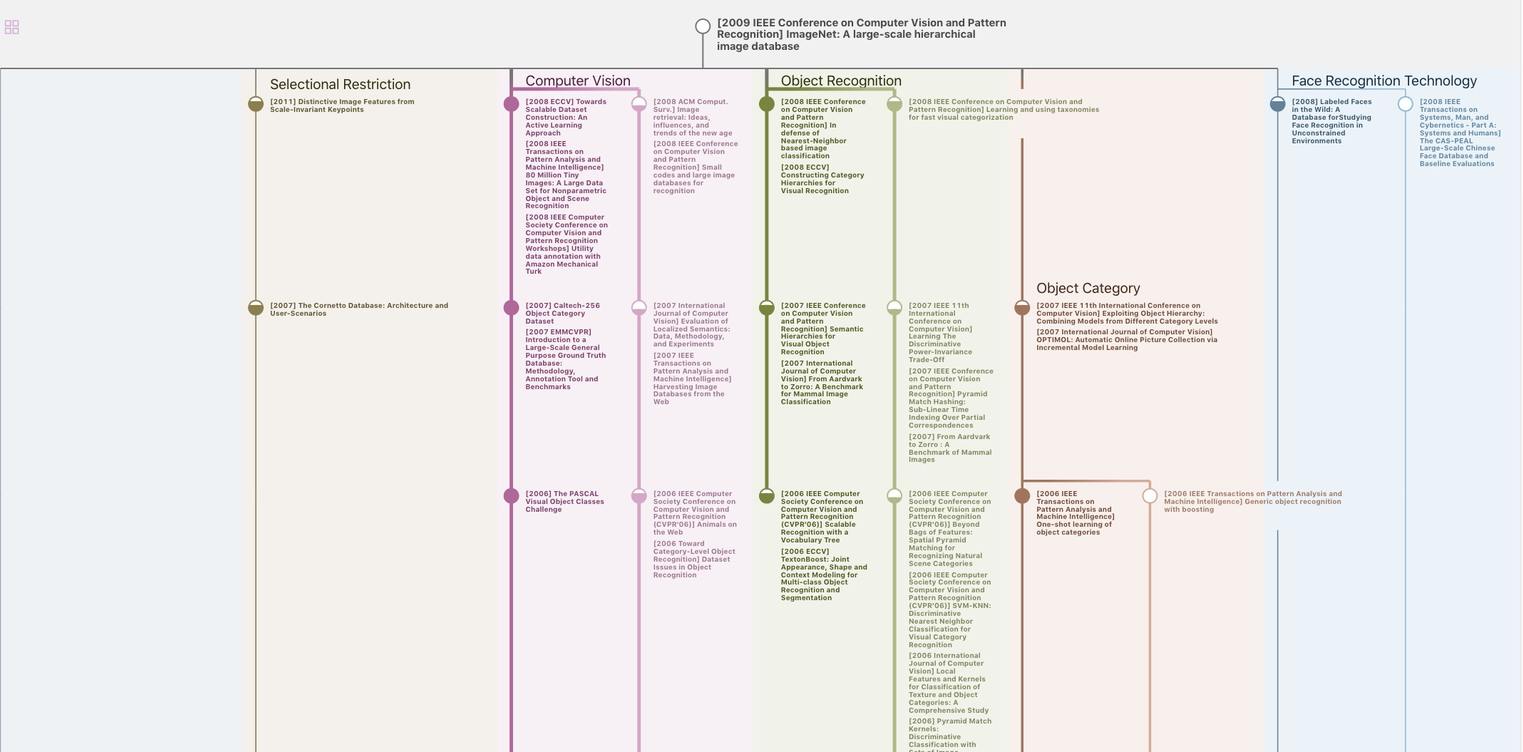
生成溯源树,研究论文发展脉络
Chat Paper
正在生成论文摘要