Addressing Overfitting on Pointcloud Classification using Atrous XCRF.
CoRR(2019)
摘要
Abstract Advances in techniques for automated classification of point cloud data introduce great opportunities for many new and existing applications. However, with a limited number of labelled points, automated classification by a machine learning model is prone to overfitting and poor generalization. The present paper addresses this problem by inducing controlled noise (on a trained model) generated by invoking conditional random field similarity penalties using nearby features. The method is called Atrous XCRF and works by forcing a trained model to respect the similarity penalties provided by unlabeled data. In a benchmark study carried out using the ISPRS 3D labeling dataset, our technique achieves 85.0% in term of overall accuracy, and 71.1% in term of F1 score. The result is on par with the current best model for the benchmark dataset and has the highest value in term of F1 score. Additionally, transfer learning using the Bergen 2018 dataset, without model retraining, was also performed. Even though our proposal provides a consistent 3% improvement in term of accuracy, more work still needs to be done to alleviate the generalization problem on the domain adaptation and the transfer learning field.
更多查看译文
AI 理解论文
溯源树
样例
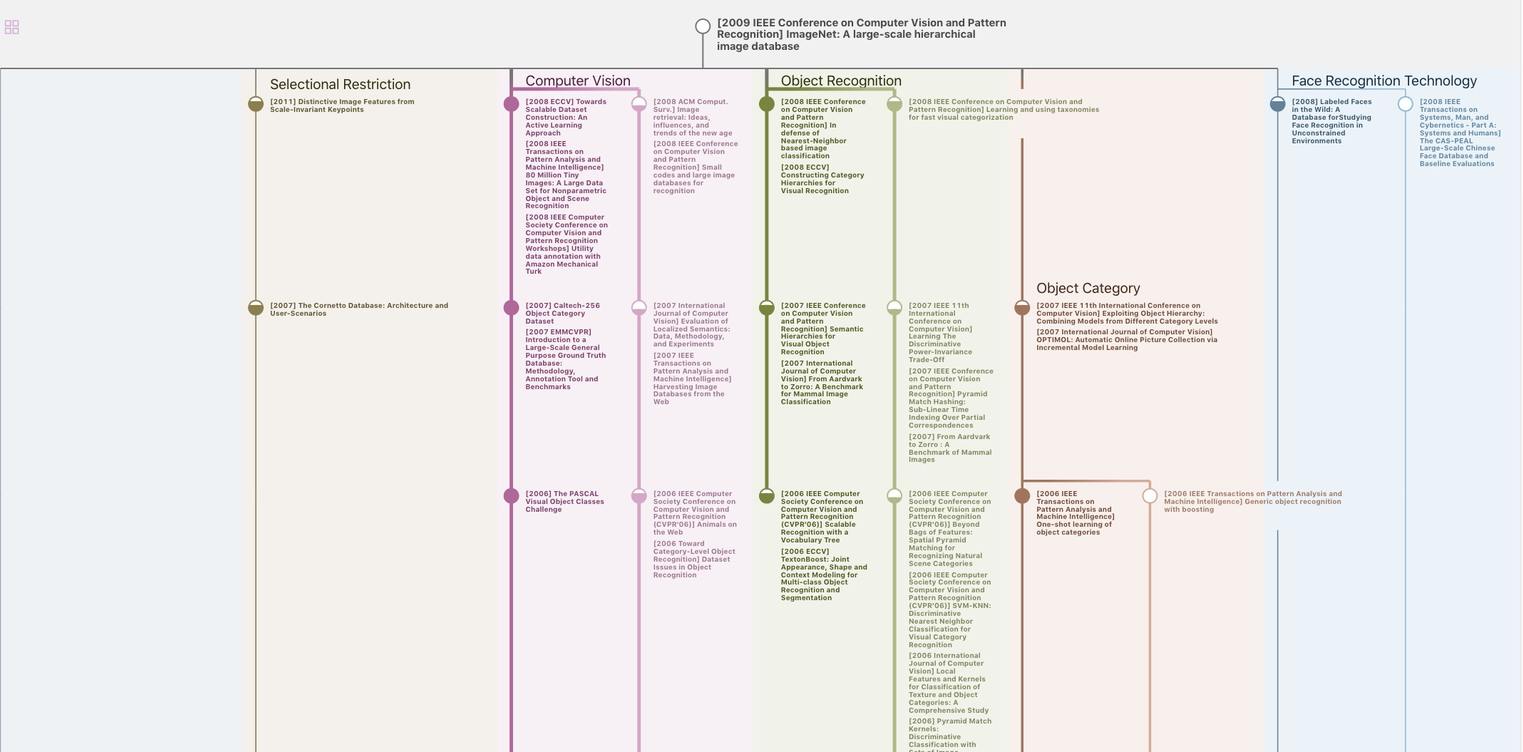
生成溯源树,研究论文发展脉络
Chat Paper
正在生成论文摘要