Deep Visual MPC-Policy Learning for Navigation.
international conference on robotics and automation(2019)
摘要
Humans can routinely follow a trajectory defined by a list of images/landmarks. However, traditional robot navigation methods require accurate mapping of the environment, localization, and planning. Moreover, these methods are sensitive to subtle changes in the environment. In this letter, we propose PoliNet, a deep visual model predictive control-policy learning method that can perform visual navigation while avoiding collisions with unseen objects on the navigation path. PoliNet takes in as input a visual trajectory and 360
$^{\circ }$
images from robot's current view and outputs velocity commands for a planning horizon of
$N$
steps that optimally balance between trajectory following and obstacle avoidance. PoliNet is trained using a differentiable neural image predictive model and a traversability estimation model in an model predictive control setup, with minimal human supervision. PoliNet can be applied to visual trajectory in new scenes without retraining. We show experimentally that the robot can follow a visual trajectory even if it does not start from the exact same position and in the presence of previously unseen obstacles. We validated our algorithm with tests both in a realistic simulation environment and in the real world outperforming state-of-the-art baselines under similar conditions in success rate, coverage rate of the trajectory, and with lower computational load. We also show that we can generate visual trajectory in simulation and execute the corresponding path in the real environment.
更多查看译文
关键词
Visual-Based Navigation,Omnidirectional Vision,Collision Avoidance
AI 理解论文
溯源树
样例
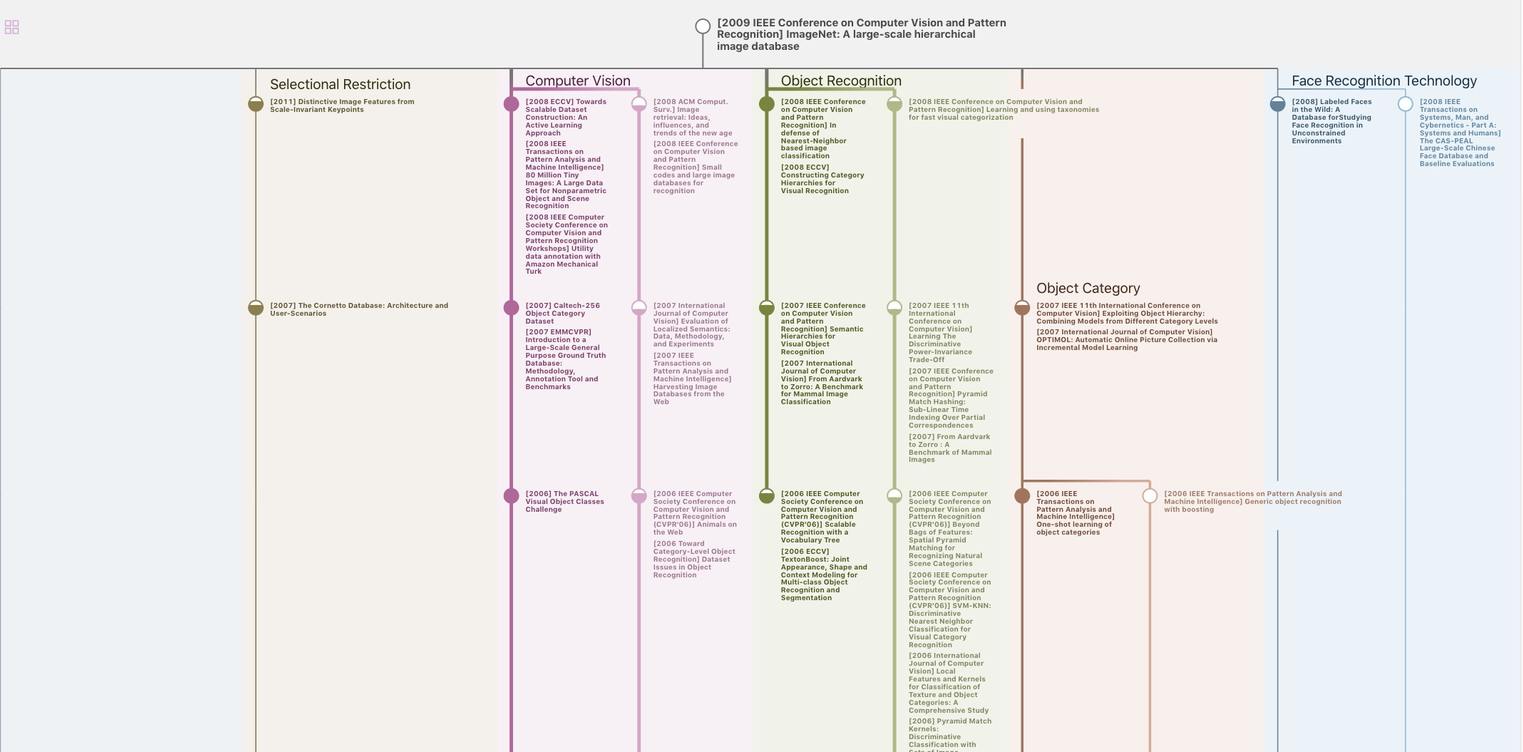
生成溯源树,研究论文发展脉络
Chat Paper
正在生成论文摘要