Clustering Bioactive Molecules in 3D Chemical Space with Unsupervised Deep Learning.
arXiv: Biomolecules(2019)
摘要
Unsupervised clustering has broad applications in data stratification, pattern investigation and new discovery beyond existing knowledge. In particular, clustering of bioactive molecules facilitates chemical space mapping, structure-activity studies, and drug discovery. These tasks, conventionally conducted by similarity-based methods, are complicated by data complexity and diversity. We ex-plored the superior learning capability of deep autoencoders for unsupervised clustering of 1.39 mil-lion bioactive molecules into band-clusters in a 3-dimensional latent chemical space. These band-clusters, displayed by a space-navigation simulation software, band molecules of selected bioactivity classes into individual band-clusters possessing unique sets of common sub-structural features beyond structural similarity. These sub-structural features form the frameworks of the literature-reported pharmacophores and privileged fragments. Within each band-cluster, molecules are further banded into selected sub-regions with respect to their bioactivity target, sub-structural features and molecular scaffolds. Our method is potentially applicable for big data clustering tasks of different fields.
更多查看译文
AI 理解论文
溯源树
样例
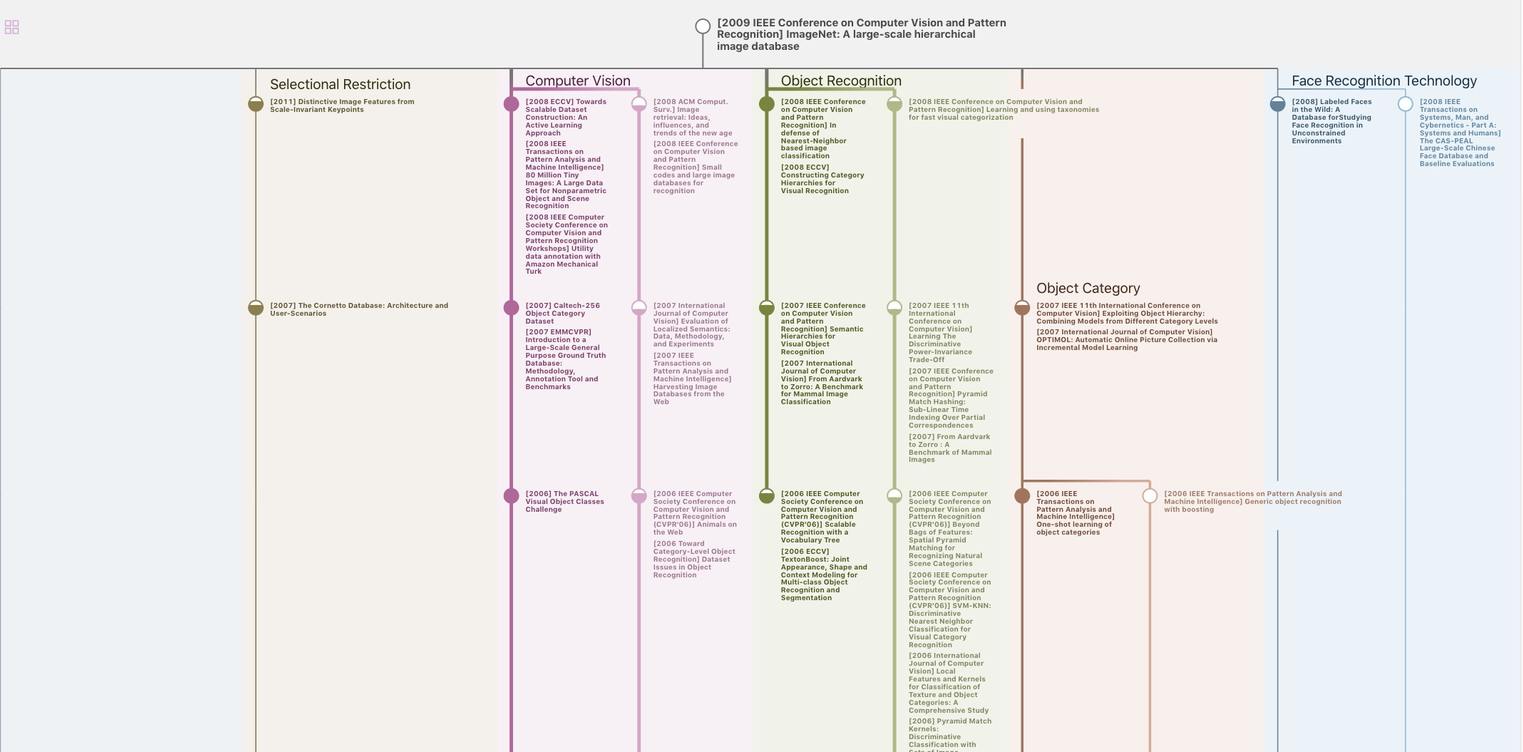
生成溯源树,研究论文发展脉络
Chat Paper
正在生成论文摘要