Enhanced Variational Inference with Dyadic Transformation.
arXiv: Learning(2019)
Abstract
Variational autoencoder is a powerful deep generative model with variational inference. The practice of modeling latent variables in the VAEu0027s original formulation as normal distributions with a diagonal covariance matrix limits the flexibility to match the true posterior distribution. We propose a new transformation, dyadic transformation (DT), that can model a multivariate normal distribution. DT is a single-stage transformation with low computational requirements. We demonstrate empirically on MNIST dataset that DT enhances the posterior flexibility and attains competitive results compared to other VAE enhancements.
MoreTranslated text
Key words
dyadic transformation
AI Read Science
Must-Reading Tree
Example
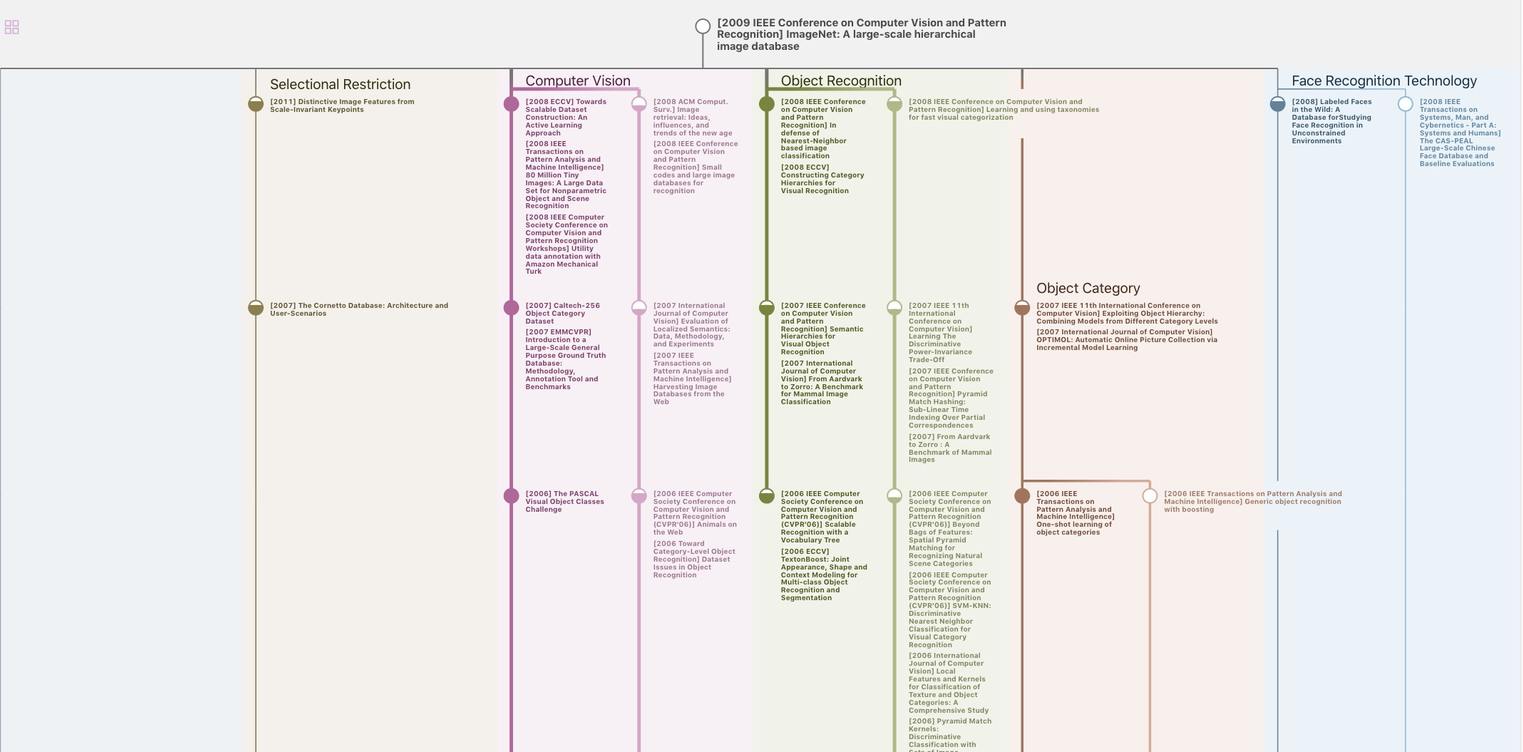
Generate MRT to find the research sequence of this paper
Chat Paper
Summary is being generated by the instructions you defined