Zero-Shot Autonomous Vehicle Policy Transfer: From Simulation to Real-World via Adversarial Learning
2020 IEEE 16th International Conference on Control & Automation (ICCA)(2020)
摘要
In this article, we demonstrate a zero-shot transfer of an autonomous driving policy from simulation to University of Delaware's scaled smart city with adversarial multi-agent reinforcement learning, in which an adversary attempts to decrease the net reward by perturbing both the inputs and outputs of the autonomous vehicles during training. We train the autonomous vehicles to coordinate with each other while crossing a roundabout in the presence of an adversary in simulation. The adversarial policy successfully reproduces the simulated behavior and incidentally outperforms, in terms of travel time, both a human-driving baseline and adversary-free trained policies. Finally, we demonstrate that the addition of adversarial training considerably improves the performance of the policies after transfer to the real world compared to Gaussian noise injection.
更多查看译文
关键词
autonomous driving policy,zero-shot autonomous vehicle policy transfer,adversarial training,simulated behavior,adversarial policy,adversarial multiagent reinforcement learning,smart city
AI 理解论文
溯源树
样例
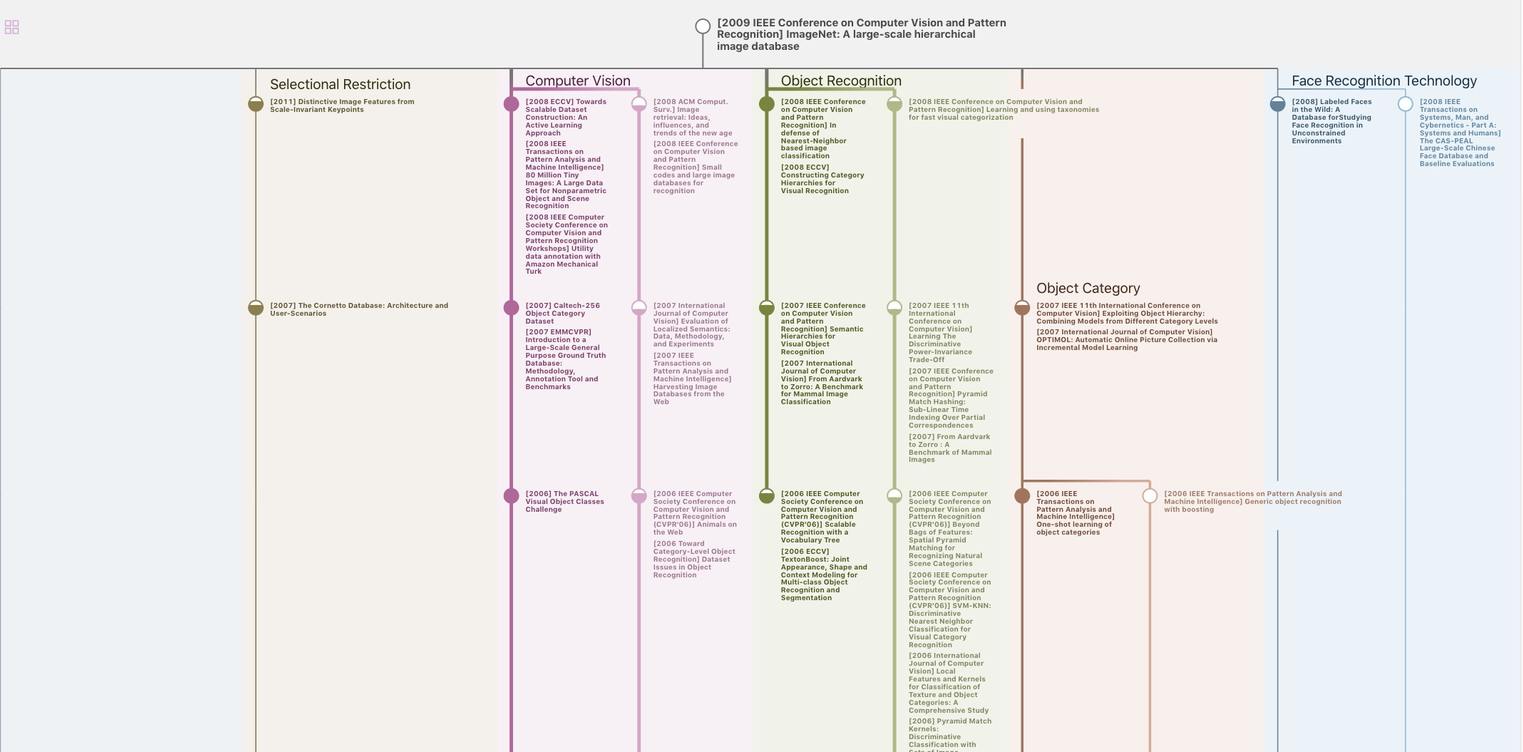
生成溯源树,研究论文发展脉络
Chat Paper
正在生成论文摘要