CodedPrivateML: A Fast and Privacy-Preserving Framework for Distributed Machine Learning
IEEE Journal on Selected Areas in Information Theory(2021)
Abstract
How to train a machine learning model while keeping the data private and secure? We present CodedPrivateML, a fast and scalable approach to this critical problem. CodedPrivateML keeps both the data and the model information-theoretically private, while allowing efficient parallelization of training across distributed workers. We characterize CodedPrivateML's privacy threshold and prove its convergence for logistic (and linear) regression. Furthermore, via extensive experiments on Amazon EC2, we demonstrate that CodedPrivateML provides significant speedup over cryptographic approaches based on multi-party computing (MPC).
MoreTranslated text
Key words
Distributed training,privacy-preserving machine learning
AI Read Science
Must-Reading Tree
Example
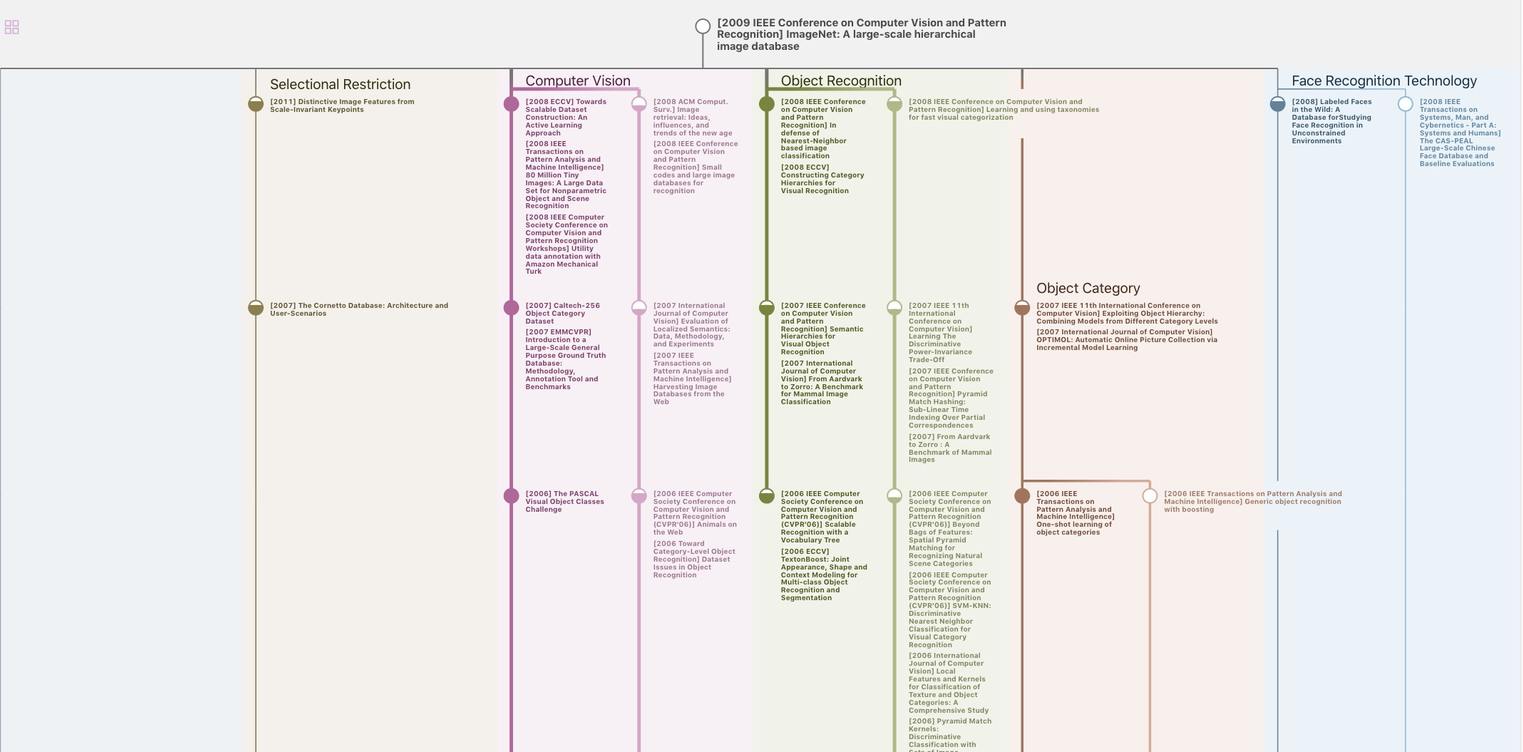
Generate MRT to find the research sequence of this paper
Chat Paper
Summary is being generated by the instructions you defined