Convolutional Neural Network Based Models for Improving Super-Resolution Imaging.
IEEE ACCESS(2019)
Abstract
Many fields, such as remote sensing, medical imaging, and biological detection, pose a technical challenge for achieving super-resolution imaging. Convolutional neural networks (CNNs) are considered one of the potential solutions to realize the super-resolution. In this paper three-layer, CNN-based models are proposed to reconstruct the super-resolution images using four optimization algorithms, i.e., stochastic gradient descent, adaptive gradient (AdaGrad), root mean square prop (RMSprop), and adaptive moment estimation (ADAM). Among these four optimizations, ADAM is considered to have the best performance. To further verify the impact of the number of convolution layers on performance, a selection of CNN-based models with four convolutional layers is then proposed, each of which is named with the convolution parameters. All the four-layer models are optimized with ADAM, and the experimental results indicate that the 9-3-3-5 model achieves the best performance in the super-resolution reconstruction task.
MoreTranslated text
Key words
Super-resolution imaging,deep learning,convolutional neural networks,adaptive moment estimation
AI Read Science
Must-Reading Tree
Example
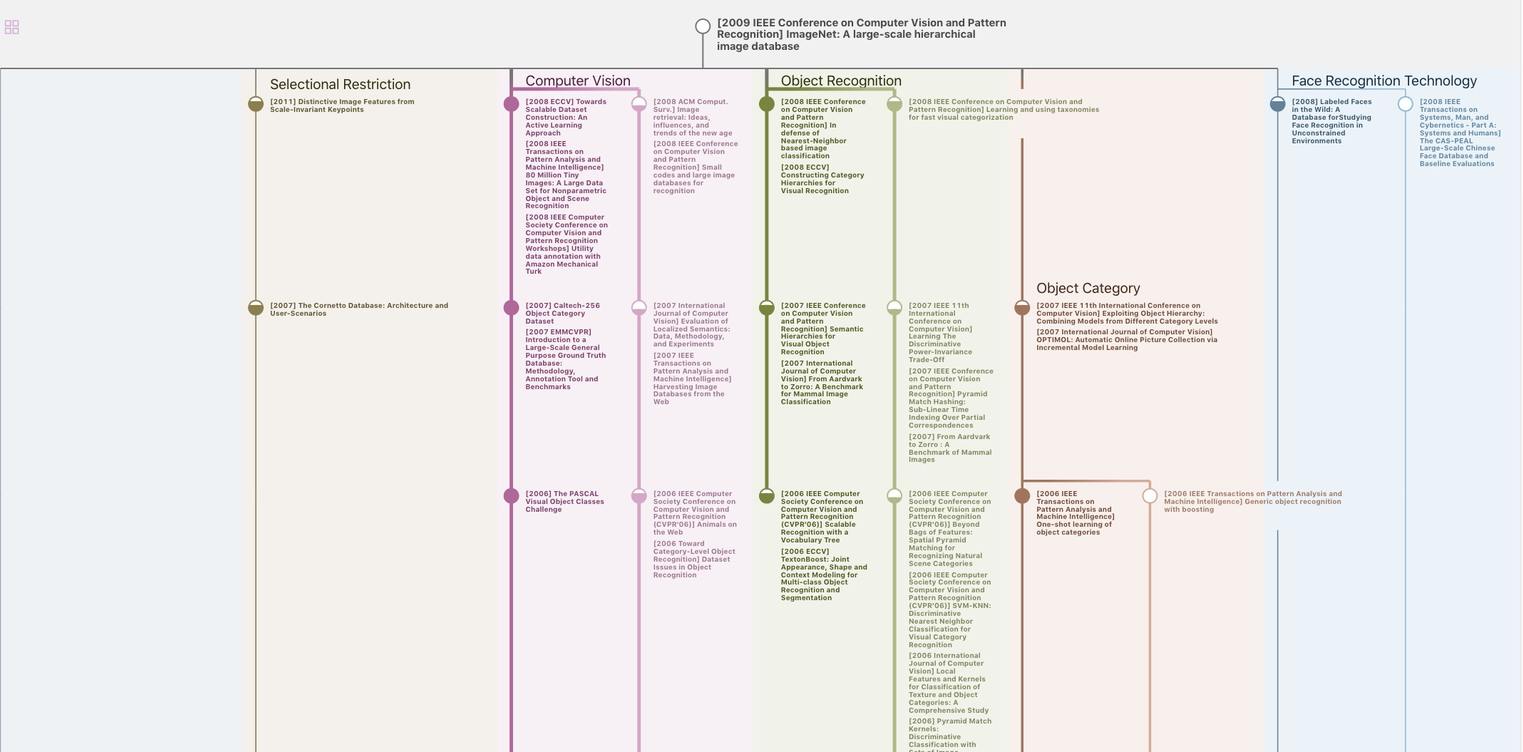
Generate MRT to find the research sequence of this paper
Chat Paper
Summary is being generated by the instructions you defined