Nested Dilation Network (NDN) for Multi-Task Medical Image Segmentation.
IEEE ACCESS(2019)
摘要
The deep convolutional network has shown excellent performance in medical image analysis. However, almost all network variants are presented for one specific task, e.g., segment pancreas on computerized tomography (CT). In this paper, we propose a nested dilation network (NDN) which is applied to multiple segmentation tasks even for different modalities, including CT, magnetic resonance imaging (MRI), and endoscopic images. We design residual blocks nested with dilations (RnD Blocks) that catch larger receptive field in the first few layers to boost shallow semantic information. Besides, we apply the modified focal loss to help the network to provide more accurate segmentation results. We evaluate our method on five subtasks from medical segmentation decathlon challenge and GIANA2018, and the results show that our method achieves a better performance than the latest methods in each task. A lot of research works have been done recently to strengthen the learning power of the convolutional neural network (CNN) to get better performance.
更多查看译文
关键词
Deep learning,medical segmentation,residual blocks nested with dilations,multi-task,focal loss
AI 理解论文
溯源树
样例
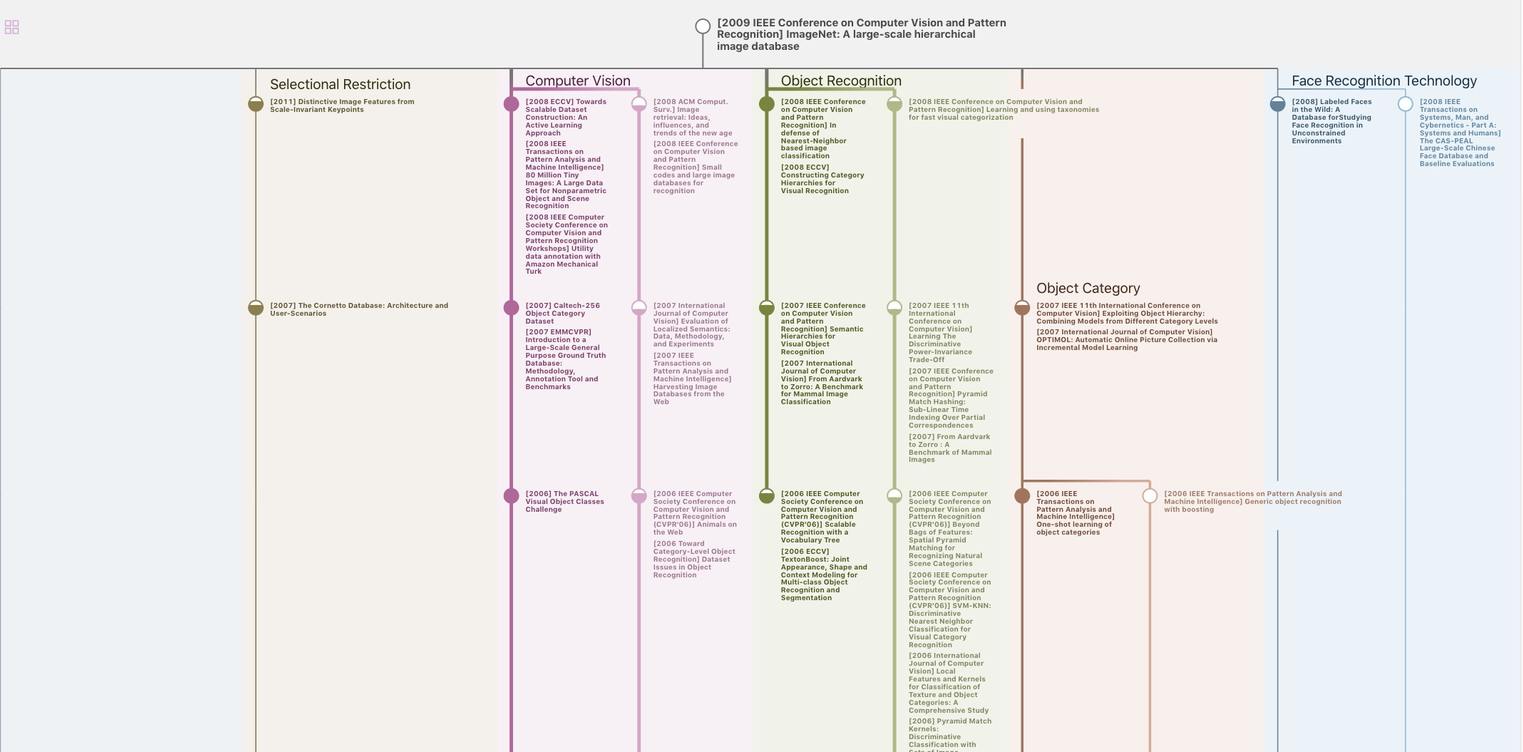
生成溯源树,研究论文发展脉络
Chat Paper
正在生成论文摘要