ABCD Neurocognitive Prediction Challenge 2019: Predicting Individual Residual Fluid Intelligence Scores from Cortical Grey Matter Morphology
Adolescent Brain Cognitive Development Neurocognitive Prediction Lecture Notes in Computer Science(2019)
Abstract
We predicted fluid intelligence from T1-weighted MRI data available as part of the ABCD NP Challenge 2019, using morphological similarity of grey-matter regions across the cortex. Individual structural covariance networks (SCN) were abstracted into graph-theory metrics averaged over nodes across the brain and in data-driven communities/modules. Metrics included degree, path length, clustering coefficient, centrality, rich club coefficient, and small-worldness. These features derived from the training set were used to build various regression models for predicting residual fluid intelligence scores, with performance evaluated both using cross-validation within the training set and using the held-out validation set. Our predictions on the test set were generated with a support vector regression model trained on the training set. We found minimal improvement over predicting a zero residual fluid intelligence score across the sample population, implying that structural covariance networks calculated from T1-weighted MR imaging data provide little information about residual fluid intelligence.
MoreTranslated text
Key words
Support vector regression,Fluid intelligence,MRI,Structural covariance networks,Graph theory features
AI Read Science
Must-Reading Tree
Example
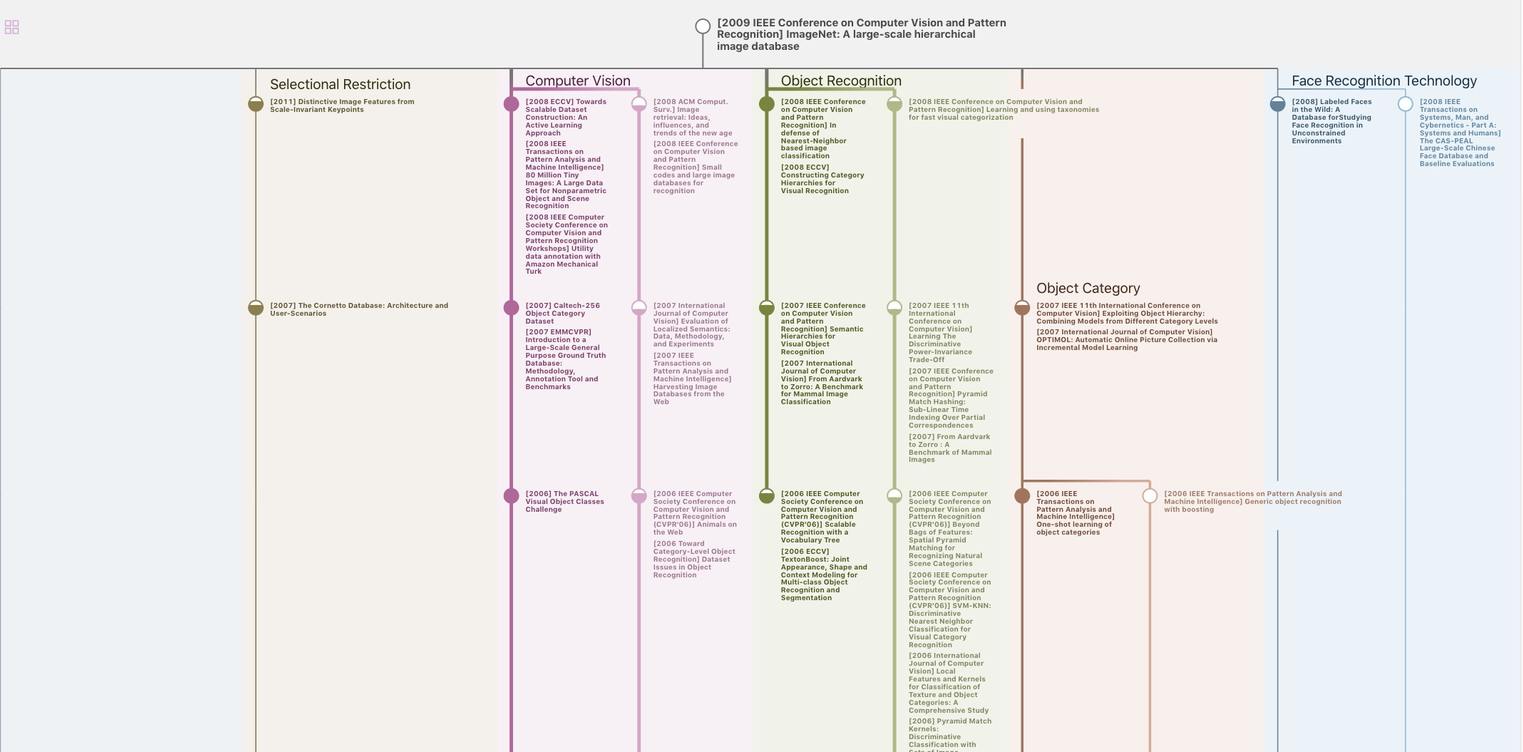
Generate MRT to find the research sequence of this paper
Chat Paper
Summary is being generated by the instructions you defined