Application of Off-policy Integral Reinforcement Learning for H∞ , Input Constrained Control of Permanent Magnet Synchronous Machine
2019 IEEE Applied Power Electronics Conference and Exposition (APEC)(2019)
Abstract
In this article, a data-driven integral reinforcement Learning control scheme is applied to Input Constrained Control of Permanent Magnet synchronous machine (PMSM). The proposed controller can maintain high performance since it formulates the machine drive into an H
∞
, input constrained control problem. To this end, a novel algorithm namely integral reinforcement learning is implemented for real-time to learn the solution of the modified Hamilton-Jacobi-Issac (HJI) equation on line.This algorithm is based on policy iteration and implemented using an actor/critic structure, which makes use of neural networks to parametrically represent the value function and control law, tunes the controller gains based on measured system outputs. The proposed controller has been experimentally validated on a 2-KW three-phase PMSM test rig.
MoreTranslated text
Key words
Reinforcement learning,Optimal control,Artificial neural networks,Mathematical model,Uncertain systems,System dynamics,Voltage control
AI Read Science
Must-Reading Tree
Example
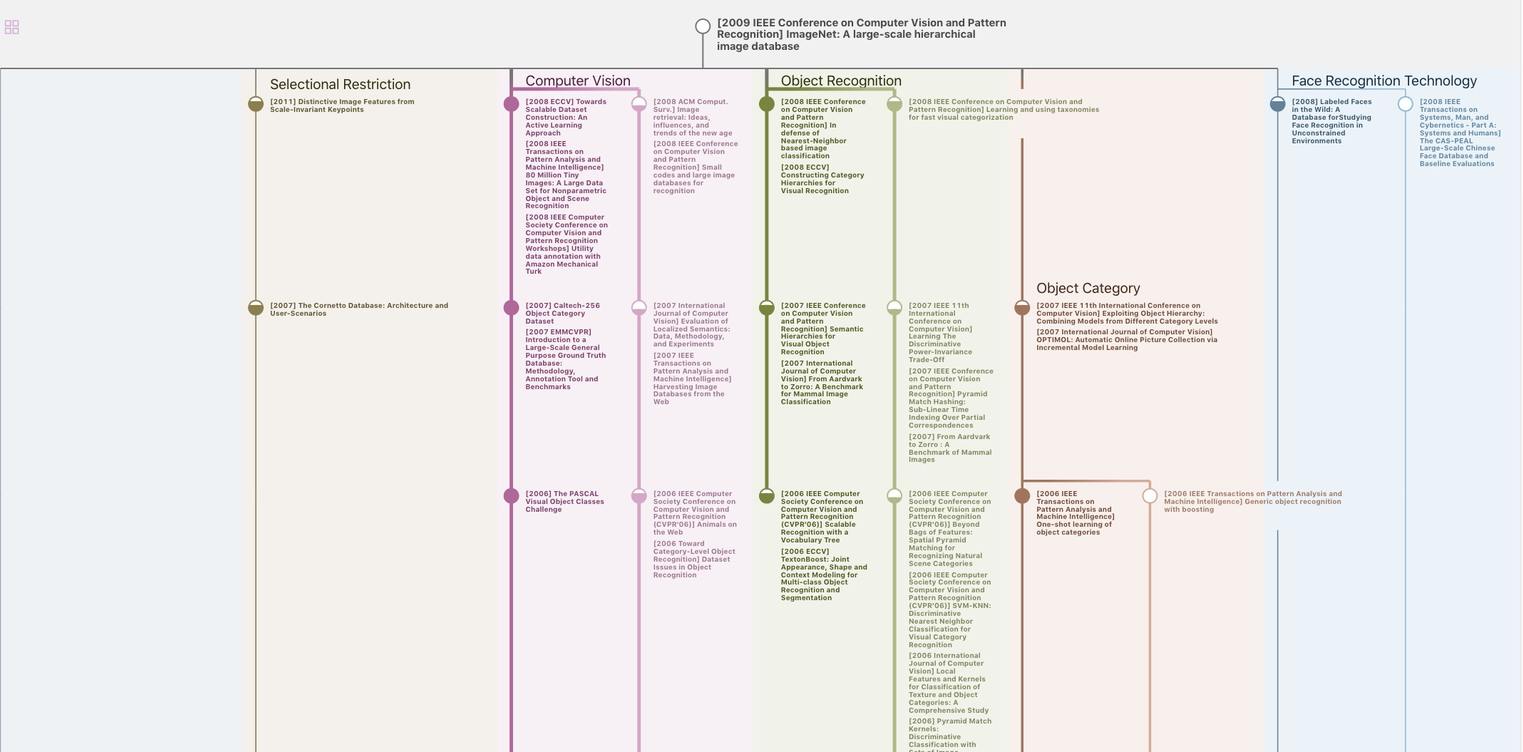
Generate MRT to find the research sequence of this paper
Chat Paper
Summary is being generated by the instructions you defined