C3-Flow: Compute Compression Co-Design Flow for Deep Neural Networks
Proceedings of the 56th Annual Design Automation Conference 2019(2019)
摘要
Existing approaches to neural network compression have failed to holistically address algorithmic (training accuracy) and computational (inference performance) demands of real-world systems, particularly on resource-constrained devices. We present C3-Flow, a new approach adding non-uniformity to low-rank approximations and designed specifically to enable highly-efficient computation on common hardware architectures while retaining more accuracy than competing methods. Evaluation on two state-of-the-art acoustic models (versus existing work, empirical limit study approaches, and hand-tuned models) demonstrates up to 60% lower error. Finally, we show that our co-design approach achieves up to 14X inference speedup across three Haswell- and Broadwell-based platforms.
更多查看译文
关键词
C3-FIow,deep neural networks,neural network compression,training accuracy,computational demands,inference performance,real-world systems,resource-constrained devices,low-rank approximations,highly-efficient computation,common hardware architectures,state-of-the-art acoustic models,hand-tuned models,co-design approach,inference speedup,compute compression co-design fiow,algorithmic demands
AI 理解论文
溯源树
样例
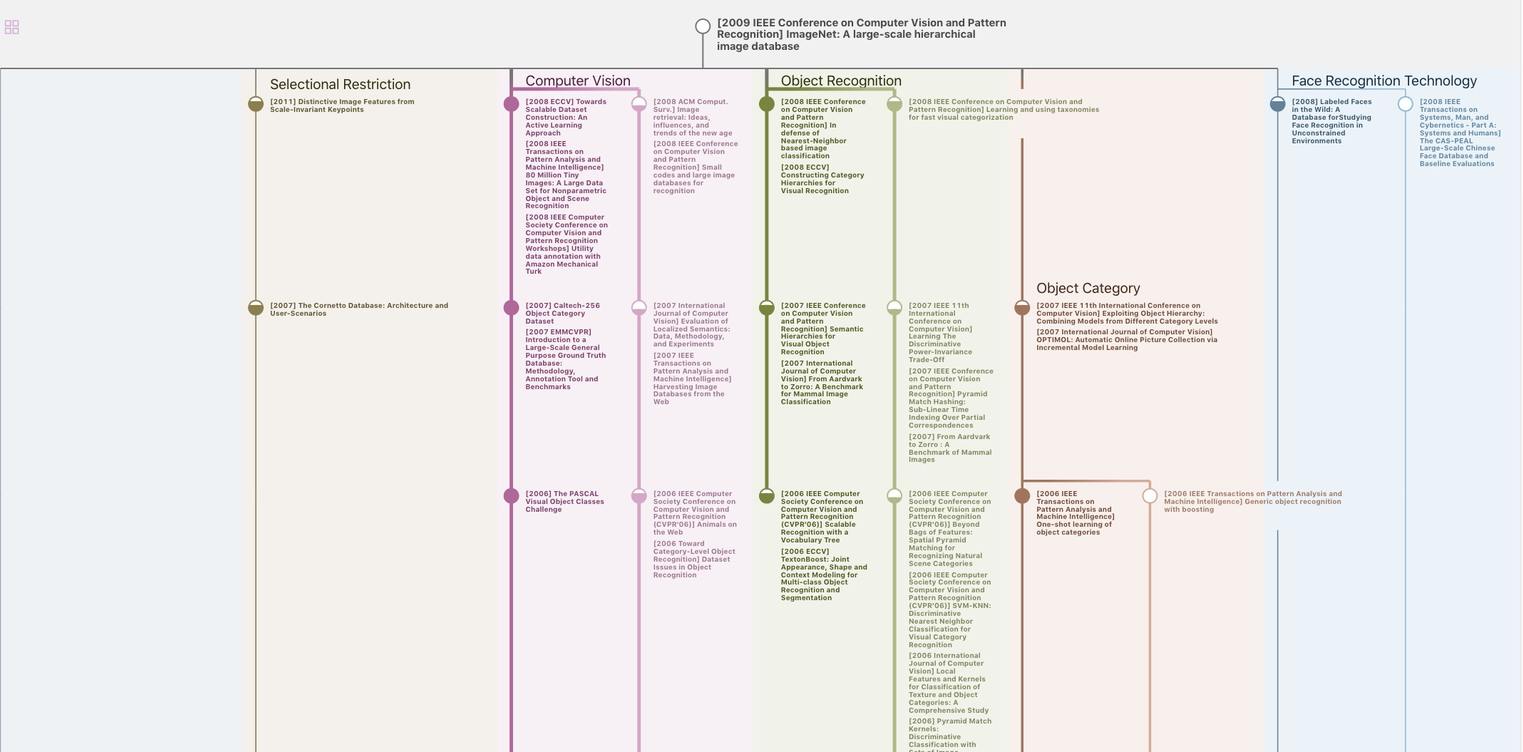
生成溯源树,研究论文发展脉络
Chat Paper
正在生成论文摘要