Challenges of comparing and matching roads from different spatial datasets
Proceedings of the 12th ACM International Conference on PErvasive Technologies Related to Assistive Environments(2019)
摘要
Road network map is one of the datasets that are used in many different applications. Many smart cities have more than one Road Network map from different sources (government authorities, private enterprise, or volunteered). Be that as it may, there is a high chance of mismatches between road maps that represent the same area for different reasons. These reasons include: one of the datasets is not updated; datasets have different names for the same road; and so on. As a result, matching the roads in such datasets with each other is challenging. This paper introduces a framework that demonstrates methods of how two datasets for the same area can be matched to each other even though there are some data discrepancies. In addition, it gives an overview of each component of the framework and focuses mainly on the similarity measurements. These measurements are local divergence measurements and global divergence measurement. Local divergence measurements compare two roads from different datasets to each other to see if they are similar or not by deciding if these two roads have a similar shape as well as the same length. On the other hand, global divergence measurement is used in order to ensure that these two roads are similar in the real world, not different roads that happen to be beside each other having similar length and shape. This paper discusses several types of applications that could utilize this framework not only for matching different road maps and unify the information for smart cities usages but also data enrichment and being up-to-date.
更多查看译文
关键词
data matching, hausdorff distance, road divergence, road networks, road similarity, smart cities, spatial databases
AI 理解论文
溯源树
样例
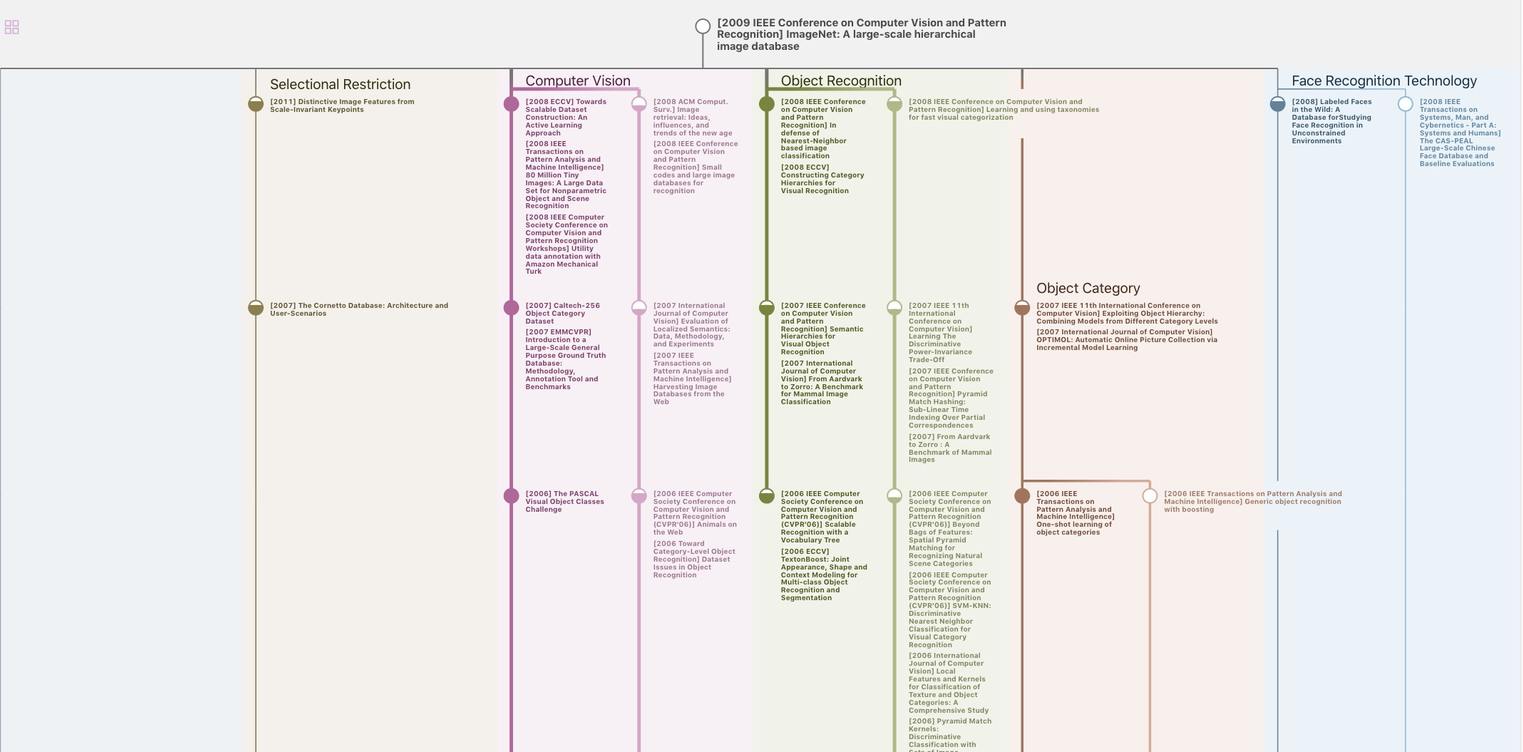
生成溯源树,研究论文发展脉络
Chat Paper
正在生成论文摘要