Crowd Prediction Under Uncertainty.
ADVANCES IN ARTIFICIAL INTELLIGENCE(2019)
摘要
On this paper we use a newly-published method, DFFT, to estimate counts of crowds on unseen environments. Our main objective is to explore the relationship between noise in the input snapshots (of crowds) that a DFFT-based pipeline requires with the errors made on the predictions. If such a relationship exists we could apply our pipeline to the understanding of crowds; this application is extremely important to our industrial partners, that see utility in predicting crowds for objectives such as security of spatial planning. Our explorations indicate the possibility of such a characterization, but it depends on features of the actual environment being studied. Here we present 2 simulated environments of different difficulty, and we show how the predictions DFFT issues are of varying quality. We discuss the reasons we hypothesize are behind these performances and we set the ground for further experiments.
更多查看译文
关键词
Multi-agent systems,AI applications,Crowd behaviour
AI 理解论文
溯源树
样例
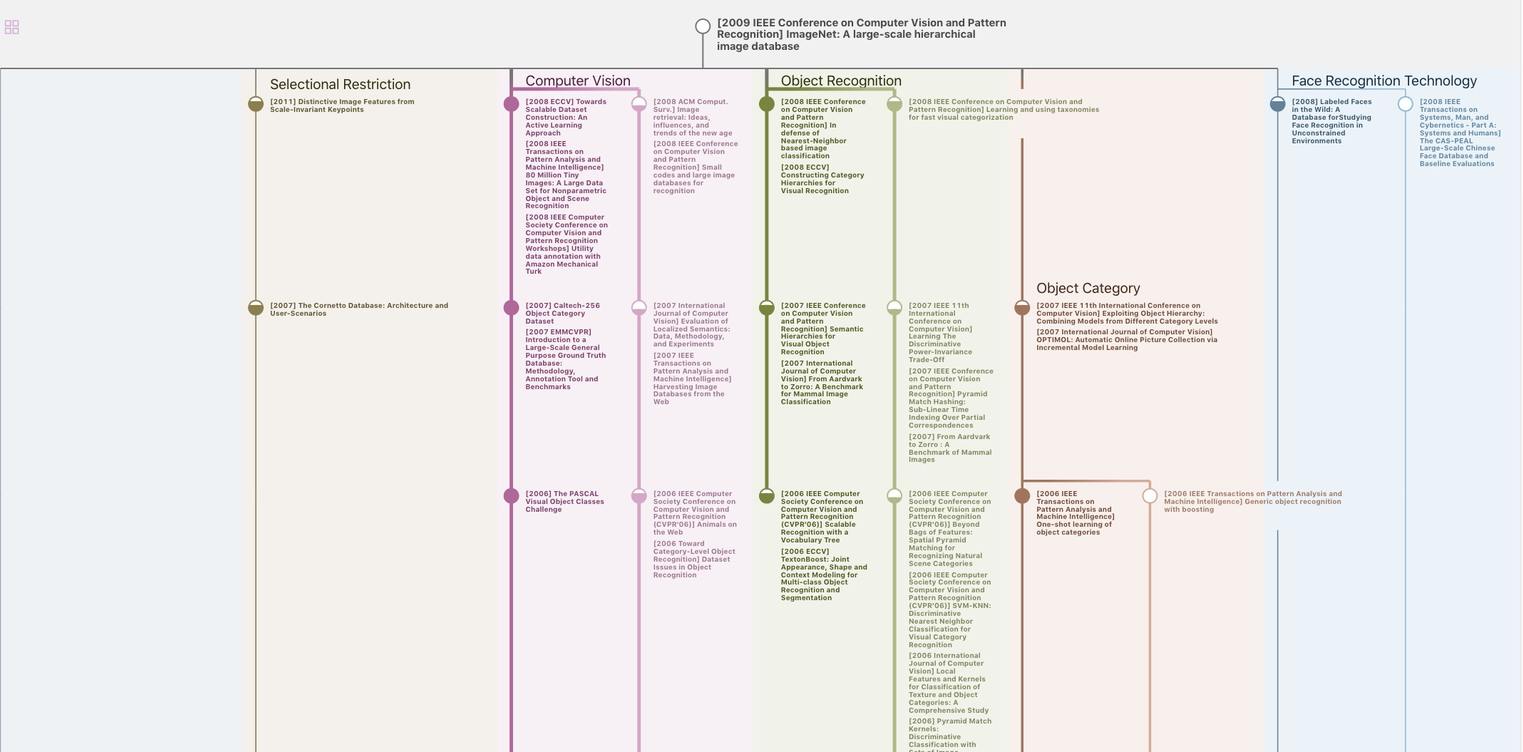
生成溯源树,研究论文发展脉络
Chat Paper
正在生成论文摘要