Image analysis and machine learning for detecting malaria
Translational Research(2018)
Abstract
Malaria remains a major burden on global health, with roughly 200 million cases worldwide and more than 400,000 deaths per year. Besides biomedical research and political efforts, modern information technology is playing a key role in many attempts at fighting the disease. One of the barriers toward a successful mortality reduction has been inadequate malaria diagnosis in particular. To improve diagnosis, image analysis software and machine learning methods have been used to quantify parasitemia in microscopic blood slides. This article gives an overview of these techniques and discusses the current developments in image analysis and machine learning for microscopic malaria diagnosis. We organize the different approaches published in the literature according to the techniques used for imaging, image preprocessing, parasite detection and cell segmentation, feature computation, and automatic cell classification. Readers will find the different techniques listed in tables, with the relevant articles cited next to them, for both thin and thick blood smear images. We also discussed the latest developments in sections devoted to deep learning and smartphone technology for future malaria diagnosis.
MoreTranslated text
Key words
GLRLM,HoG,HSV,IEEE,LBP,LED,NIH,NLM,NM,P,PCR,PLOS,QFT,QPI,RDT,RGB,RNA,SBFSEM,SEM,SightDx,SROFM,SUSAN,SVM,WHO
AI Read Science
Must-Reading Tree
Example
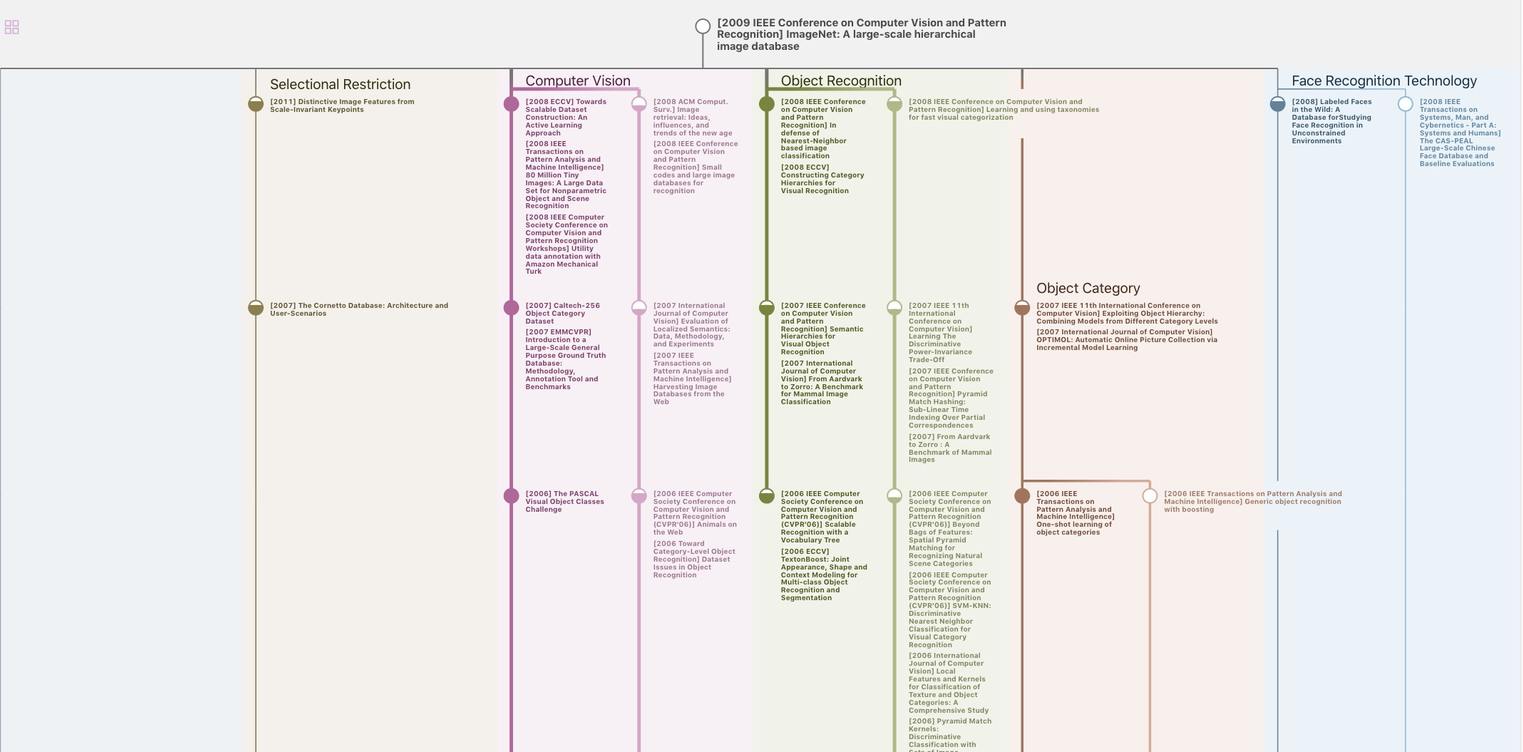
Generate MRT to find the research sequence of this paper
Chat Paper
Summary is being generated by the instructions you defined