USE OF MACHINE LEARNING ALGORITHMS TO CLASSIFY SURGICAL MANEUVERS
The Journal of Urology(2019)
摘要
You have accessJournal of UrologySurgical Technology & Simulation: Training & Skills Assessment I (PD27)1 Apr 2019PD27-03 USE OF MACHINE LEARNING ALGORITHMS TO CLASSIFY SURGICAL MANEUVERS Brady Miller*, David Azari, Yu H Yu, Robert Radwin, and Brian Le Brady Miller*Brady Miller* More articles by this author , David AzariDavid Azari More articles by this author , Yu H YuYu H Yu More articles by this author , Robert RadwinRobert Radwin More articles by this author , and Brian LeBrian Le More articles by this author View All Author Informationhttps://doi.org/10.1097/01.JU.0000555939.51490.43AboutPDF ToolsAdd to favoritesDownload CitationsTrack CitationsPermissionsReprints ShareFacebookLinked InTwitterEmail Abstract INTRODUCTION AND OBJECTIVES: Technology allowing automatic interpretation of complex surgical tasks have wide ranging applications in teaching, robotics and cloud-based computing. Computer vision recognition of surgical maneuvers (e.g. suturing, tying, etc.) can expedite video review and objective analysis. Here, we explore how common machine learning techniques can predict surgical maneuvers from a continuous video record of surgical benchtop simulations. METHODS: Hand movements of 37 clinicians performing simple and running subcuticular suturing benchtop simulations without sensors were recorded via digital video. Three machine learning techniques (decision trees, random forests, and hidden markov models) were selected to classify surgical maneuvers every two seconds (60 frames) of video. Twenty percent of all video segments were randomly selected to serve as a test set for random forest prediction across all tasks, while thirty percent of participants from each experience group (medical students, junior residents, senior residents, attendings) comprised a testing set of twelve participants (ie, participant-controlled). This allowed for both random selection and within-user population accuracy prediction estimates. RESULTS: Applying the following machine learning techniques, computers were able to accurately classify surgical maneuvers. Random forest predictions of surgical video correctly classified 74% of all video segments into suturing, tying, and transition states for a randomly selected test set. By comparison, decision tree analysis correctly classified 64% of video segments. Hidden markov model adjustments improved the random forest predictions to 79% for simple interrupted suturing on a subset of randomly selected participants. CONCLUSIONS: Random forest predictions aided by hidden markov modeling provided the best prediction of surgical maneuvers. Marker-less video motion tracking combined with machine learning can accurately predict surgical maneuvers from a continuous video record and may enable more efficient video review of surgical procedures. Source of Funding: Society of Academic Urologists Madison, WI© 2019 by American Urological Association Education and Research, Inc.FiguresReferencesRelatedDetails Volume 201Issue Supplement 4April 2019Page: e481-e482 Advertisement Copyright & Permissions© 2019 by American Urological Association Education and Research, Inc.MetricsAuthor Information Brady Miller* More articles by this author David Azari More articles by this author Yu H Yu More articles by this author Robert Radwin More articles by this author Brian Le More articles by this author Expand All Advertisement PDF downloadLoading ...
更多查看译文
关键词
machine learning algorithms,machine learning
AI 理解论文
溯源树
样例
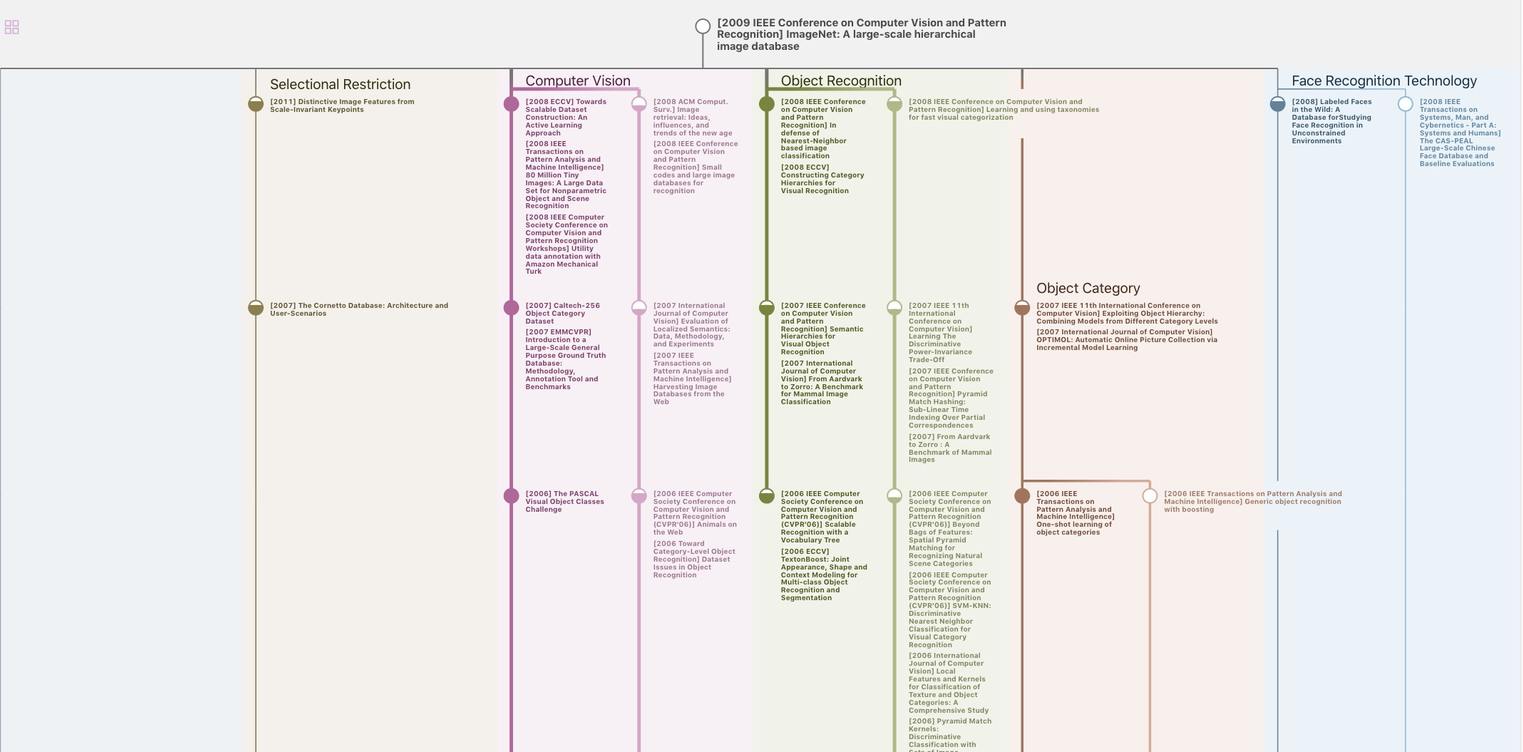
生成溯源树,研究论文发展脉络
Chat Paper
正在生成论文摘要