Valid machine learning algorithms for multiparameter methods
Accreditation and Quality Assurance(2019)
摘要
In the light of recent food fraud cases, the issue of food authenticity is receiving increasing attention. New analytical methods and evaluation approaches are currently being proposed. In this framework, the evaluation of mass spectral profiles constitutes a promising avenue, e.g. for the determination of food origin. Relevant evaluation approaches include principal component analysis, artificial neural networks, random forests, support vector machines, etc. The aim is to derive decision rules for the assignation of unknown samples to different classes. These decision rules are derived on the basis of samples whose origin is known—the training set. Typically, a reliable evaluation requires that the number of samples should be considerably larger than the number of features. However, in the framework of multiparameter mass spectrometry methods, the required ratio between sample and parameter numbers is inverted, with, e.g. 100 samples versus 10 000 features. In this paper, two approaches for the establishment of reliable decision rules in spite of low sample numbers are discussed.
更多查看译文
关键词
Principal component analysis, Artificial neural networks, Non-target sampling, Food fingerprinting, Food authenticity, Food fraud, Single-class classification, Decision rule
AI 理解论文
溯源树
样例
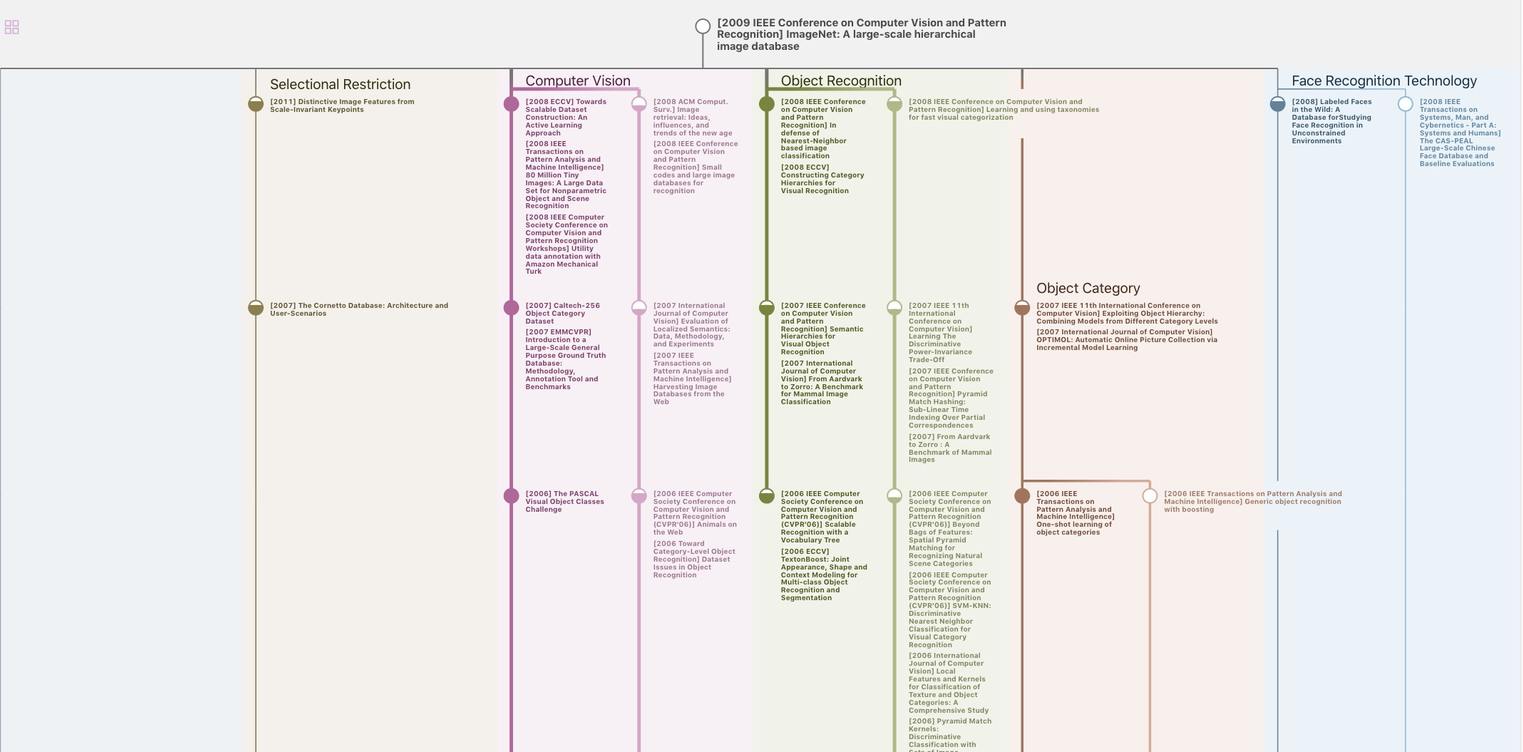
生成溯源树,研究论文发展脉络
Chat Paper
正在生成论文摘要