Tdn: An Integrated Representation Learning Model Of Knowledge Graphs
IEEE ACCESS(2019)
摘要
Knowledge graph (KG) is playing an important role in many artificial intelligence applications. Representation learning of KGs aims to project both entities and relations into a continuous low-dimensional space. The representation learning technique based on embedding has been used to implement the KG completion, which aims to predict potential triples (head, relation, and tail) in KG. Most current methods concentrate on learning representations based on triple information while ignoring integrating the textual knowledge and network topology of KG. This leads to ambiguous completions. To address this problem and implement more accurate KG completion, we propose a new representation learning model, TDN model, which integratedly embeds the information of triples, text descriptions, and network structure of KG in a low-dimensional vector space. The framework of TDN is defined and the methodology of implementing TDN embedding is explored. To verify the effectiveness of the proposed model, we evaluate TDN via the experiments of link prediction on the real-world datasets. The experimental results confirm the above claims and show that TDN-based embedding significantly outperforms other baselines.
更多查看译文
关键词
Artificial intelligence, embedding, knowledge graph, knowledge representation
AI 理解论文
溯源树
样例
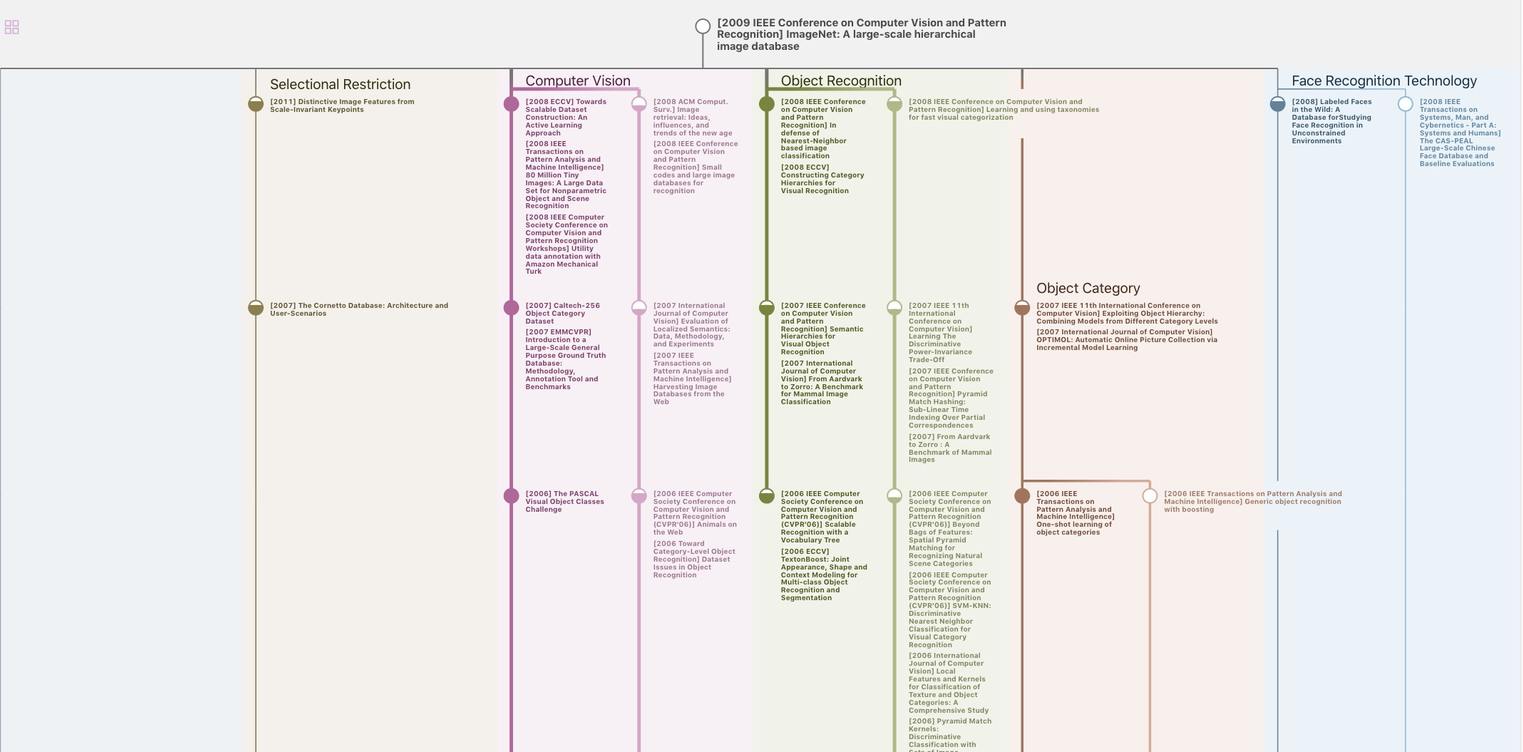
生成溯源树,研究论文发展脉络
Chat Paper
正在生成论文摘要