Boundary Discriminative Large Margin Cosine Loss For Text-Independent Speaker Verification
2019 IEEE INTERNATIONAL CONFERENCE ON ACOUSTICS, SPEECH AND SIGNAL PROCESSING (ICASSP)(2019)
摘要
Deep neural network based speaker embeddings have attracted much attention in text-independent speaker verification task. In addition to the network architecture, an appropriate design of the loss function is crucial for the deep discriminative embedding extractor. Inspired by the success of Large Margin Cosine Loss (LMCL) in face recognition, we propose an enhanced LMCL named boundary discriminative LMCL (BD-LMCL) to emphasize the discriminative information inherited in the speaker boundaries. Unlike LMCL, where all training samples contribute equally for the objective function, only the samples around the speaker boundaries are considered during the network training with BD-LMCL. Specifically, those samples close to the boundaries are dynamically selected using top-k zero-one loss. Experimental results on a short duration corpus Android Cellphone and NIST SRE 2012 demonstrate better performance compared to LMCL and other popular loss functions.
更多查看译文
关键词
speaker embedding, loss function, boundary, top-k loss, speaker verification
AI 理解论文
溯源树
样例
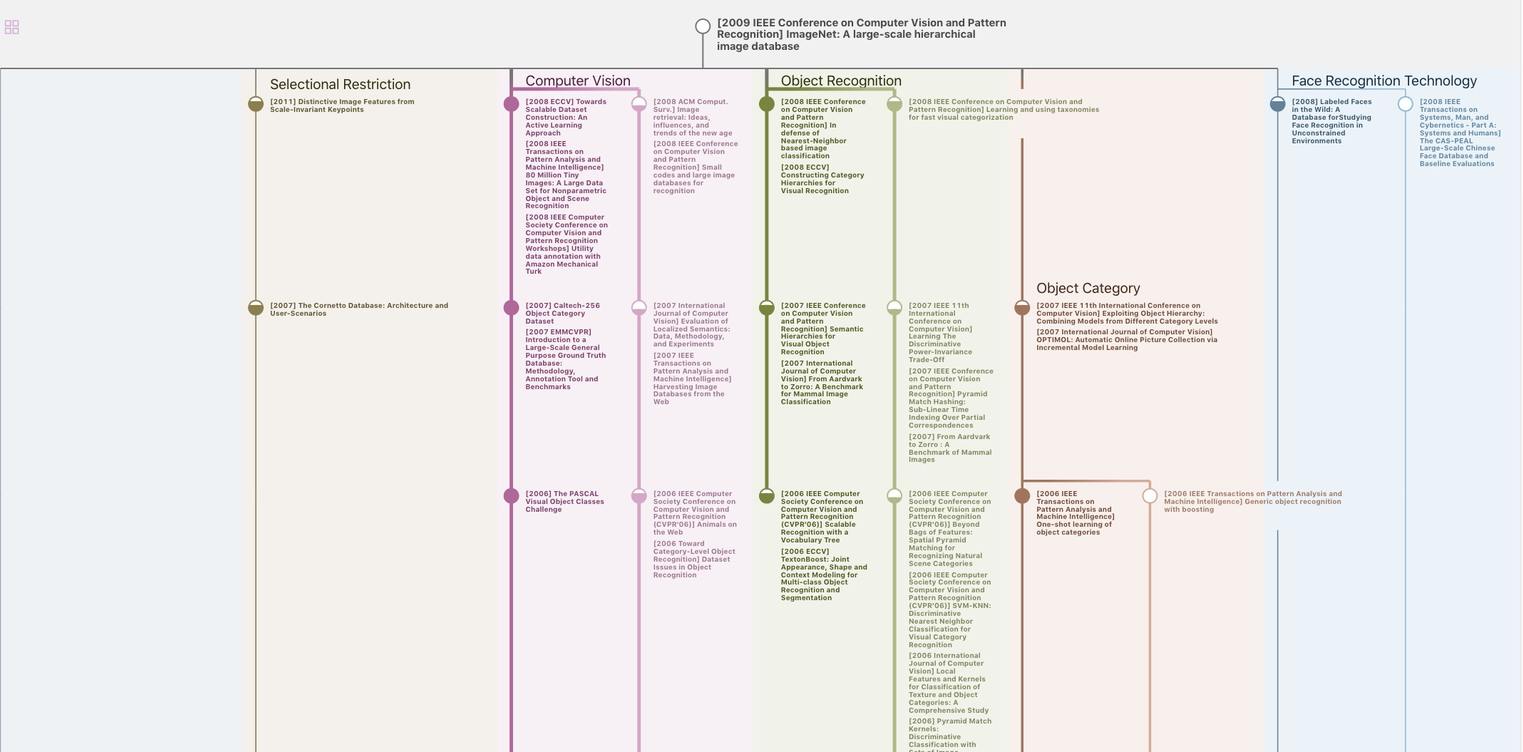
生成溯源树,研究论文发展脉络
Chat Paper
正在生成论文摘要