PolSAR Image Classification Based on Multi-scale Stacked Sparse Autoencoder
Neurocomputing(2019)
摘要
Recently, many deep learning methods are applied with the spatial information to learn features for polarimetric synthetic aperture radar (PolSAR) image classification. However, without considering the multi-scale information, the classification performance of these methods are limited. Hence, this paper proposes a multi-scale feature extraction method based on stacked sparse autoencoder (SSAE), named the multi-scale SSAE (MS-SSAE), to improve the classification performance. This method extracts the deep multi-scale features by a two-stage framework. In the first stage, the SSAE uses training data at different scales to extract the multi-scale features. Then, a 1-D average pooling strategy is proposed to reduce the feature dimensionality at the second stage. Therefore, the MS-SSAE can capture discriminative multi-scale features. The experimental results certify that the MS-SSAE can not only improve the classification accuracy, but also remain the details in the image.
更多查看译文
关键词
Polsar classification,Multi-scale,Stacked sparse autoencoder,Deep learning,Feature learning
AI 理解论文
溯源树
样例
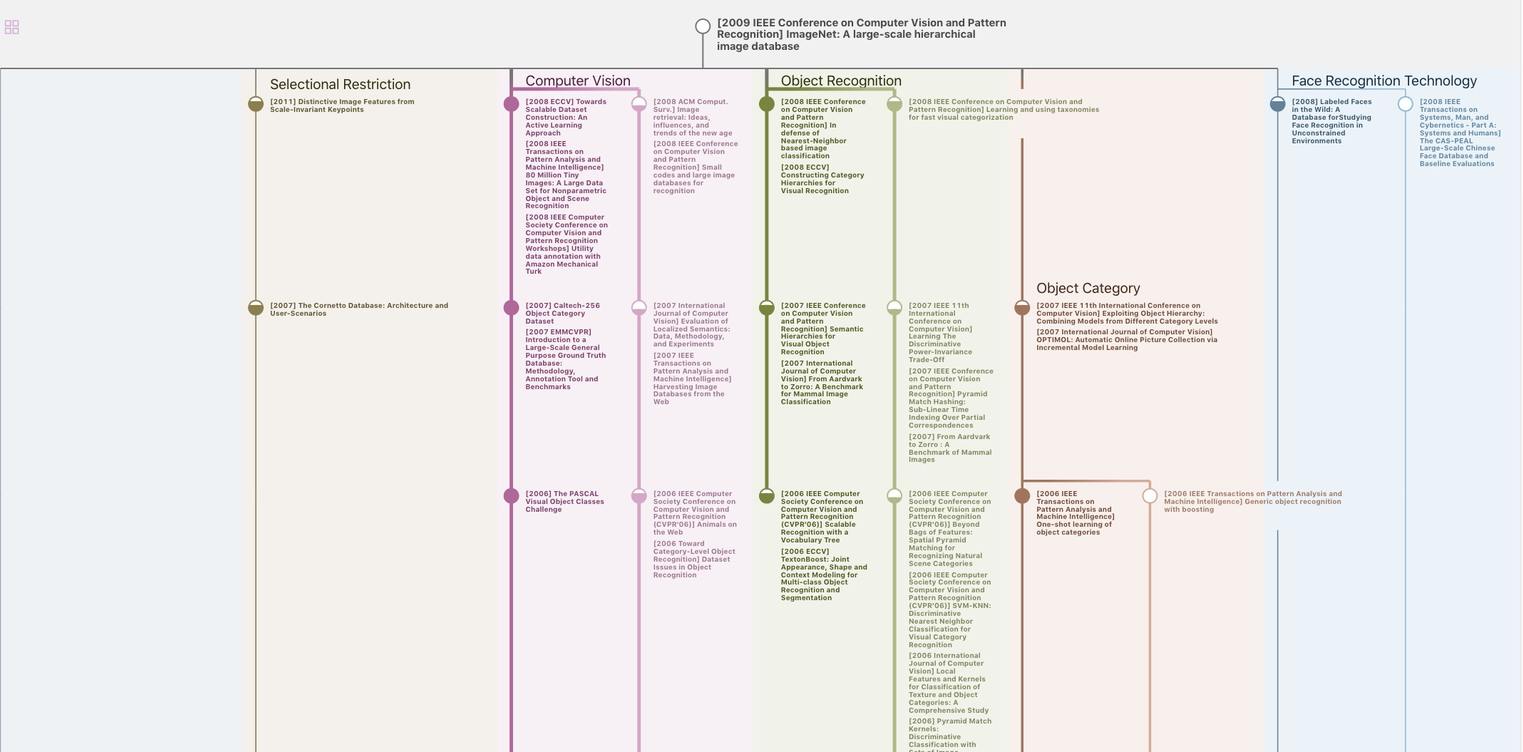
生成溯源树,研究论文发展脉络
Chat Paper
正在生成论文摘要