Nonlinear Multi-Scale Super-Resolution Using Deep Learning
2019 IEEE INTERNATIONAL CONFERENCE ON ACOUSTICS, SPEECH AND SIGNAL PROCESSING (ICASSP)(2019)
摘要
We propose a deep learning architecture capable of performing up to 8x single image super-resolution. Our architecture incorporates an adversarial component from the super-resolution generative adversarial networks (SRGANs) and a multi-scale learning component from the multiple scale super-resolution network (MSSRNet), which only together can recover smaller structures inherent in satellite images. To further enhance our performance, we integrate progressive growing and training to our network. This, aided by feed forwarding connections in the network to move along and enrich information from previous inputs, produces super-resolved images at scaling factors of 2, 4, and 8. To ensure and enhance the stability of GANs, we employ Wasserstein GANs (WGANs) during training. Experimentally, we find that our architecture can recover small objects in satellite images during super-resolution whereas previous methods cannot.
更多查看译文
关键词
super-resolution, remote sensing data, GANs, dilated convolutions
AI 理解论文
溯源树
样例
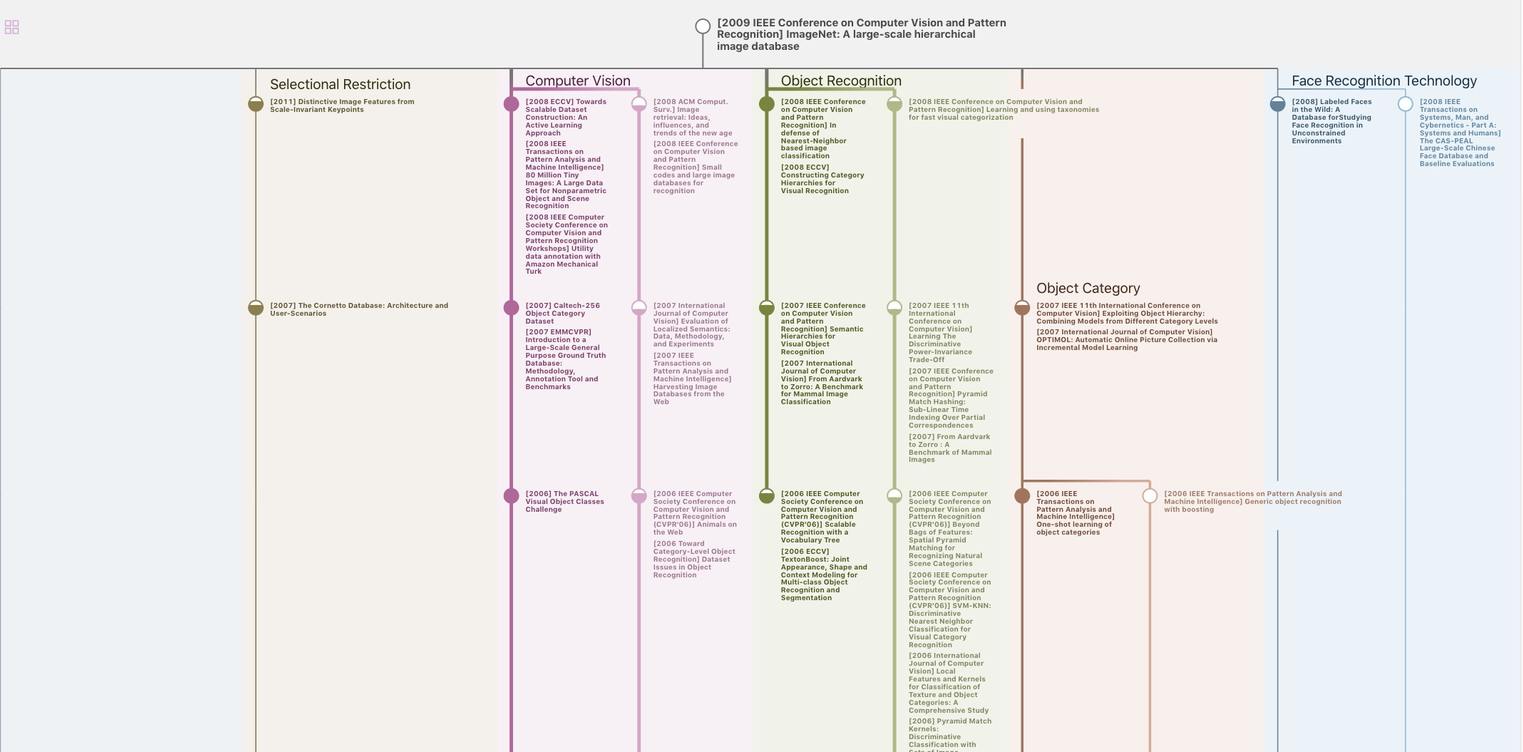
生成溯源树,研究论文发展脉络
Chat Paper
正在生成论文摘要