Supervised and unsupervised relevance sampling in handcrafted and deep learning features obtained from image collections
Applied Soft Computing(2019)
摘要
Image collections are currently widely available and are being generated in a fast pace due to mobile and accessible equipment. In principle, that is a good scenario taking into account the design of successful visual pattern recognition systems. However, in particular for classification tasks, one may need to choose which examples are more relevant in order to build a training set that well represents the data, since they often require representative and sufficient observations to be accurate. In this paper we investigated three methods for selecting relevant examples from image collections based on learning models from small portions of the available data. We considered supervised methods that need labels to allow selection, and an unsupervised method that is agnostic to labels. The image datasets studied were described using both handcrafted and deep learning features. A general purpose algorithm is proposed which uses learning methods as subroutines. We show that our relevance selection algorithm outperforms random selection, in particular when using unlabelled data in an unsupervised approach, significantly reducing the size of the training set with little decrease in the test accuracy.
更多查看译文
关键词
Image classification,Relevance sampling,Feature learning,Clustering
AI 理解论文
溯源树
样例
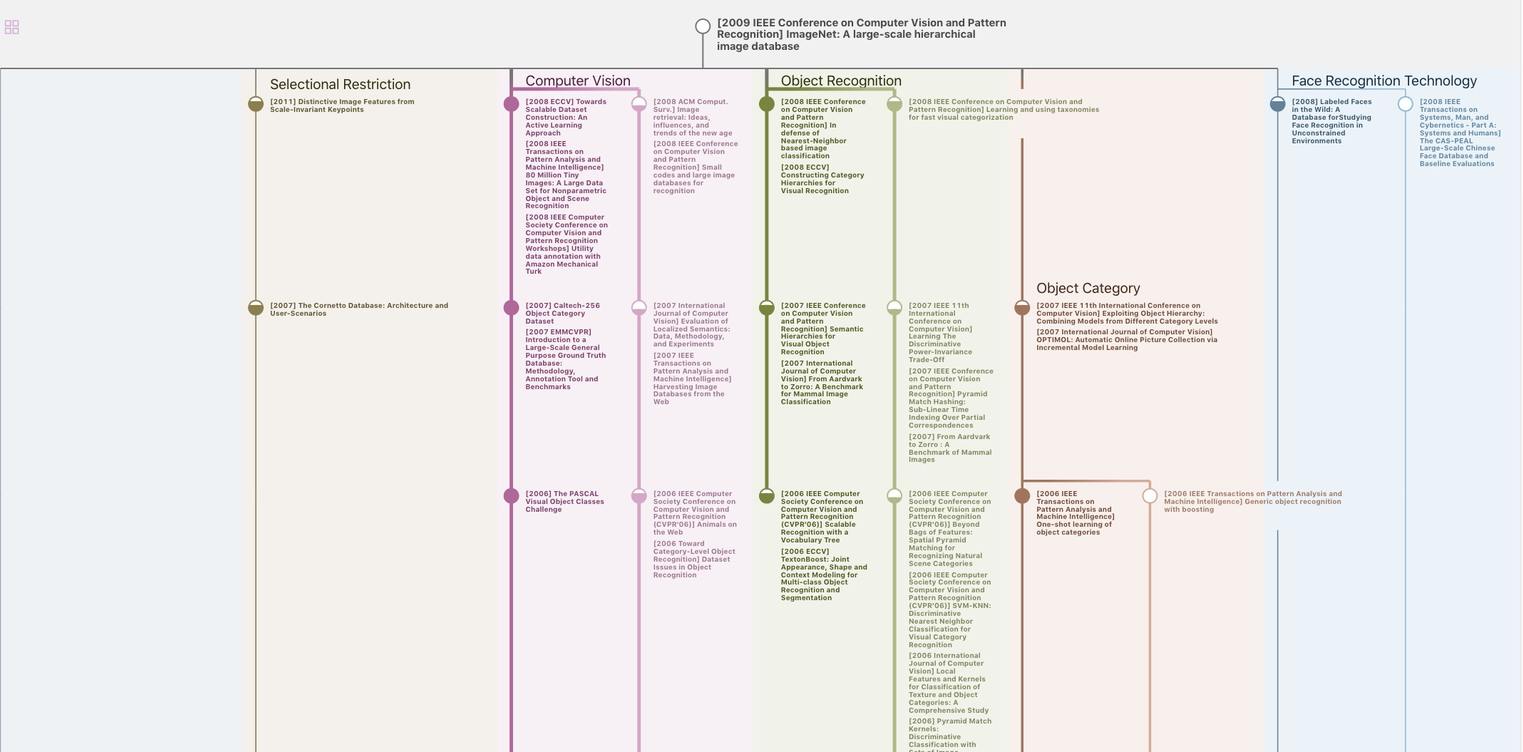
生成溯源树,研究论文发展脉络
Chat Paper
正在生成论文摘要