Applications of machine learning in drug discovery and development
NATURE REVIEWS DRUG DISCOVERY(2019)
Abstract
Drug discovery and development pipelines are long, complex and depend on numerous factors. Machine learning (ML) approaches provide a set of tools that can improve discovery and decision making for well-specified questions with abundant, high-quality data. Opportunities to apply ML occur in all stages of drug discovery. Examples include target validation, identification of prognostic biomarkers and analysis of digital pathology data in clinical trials. Applications have ranged in context and methodology, with some approaches yielding accurate predictions and insights. The challenges of applying ML lie primarily with the lack of interpretability and repeatability of ML-generated results, which may limit their application. In all areas, systematic and comprehensive high-dimensional data still need to be generated. With ongoing efforts to tackle these issues, as well as increasing awareness of the factors needed to validate ML approaches, the application of ML can promote data-driven decision making and has the potential to speed up the process and reduce failure rates in drug discovery and development.
MoreTranslated text
Key words
Diseases,Drug discovery,Imaging,Machine learning,Biomedicine,general,Pharmacology/Toxicology,Biotechnology,Medicinal Chemistry,Molecular Medicine,Cancer Research
AI Read Science
Must-Reading Tree
Example
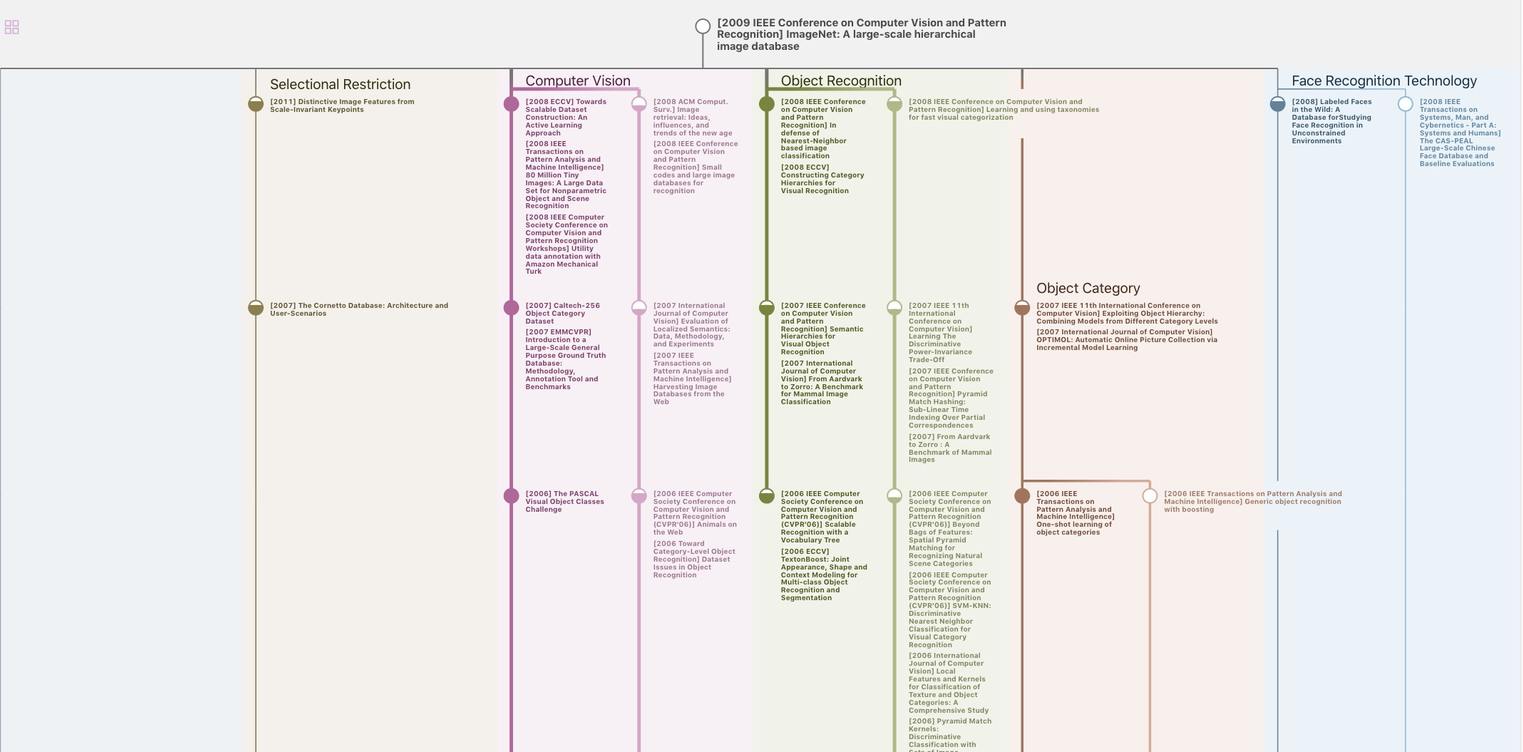
Generate MRT to find the research sequence of this paper
Chat Paper
Summary is being generated by the instructions you defined