DEEP LEARNING APPLIED TO SEISMIC ATTRIBUTE COMPUTATION
INTERPRETATION-A JOURNAL OF BIBLE AND THEOLOGY(2019)
摘要
We have trained deep convolutional neural networks (DCNs) to accelerate the computation of seismic attributes by an order of magnitude. These results are enabled by overcoming the prohibitive memory requirements typical of 3D DCNs for segmentation and regression by implementing a novel, memory-efficient 3D-to-2D convolutional architecture and by including tens of thousands of synthetically generated labeled examples to enhance DCN training. Including diverse synthetic labeled seismic in training helps the network generalize enabling it to accurately predict seismic attribute values on field-acquired seismic surveys. Once trained, our DCN tool generates attributes with no input parameters and no additional user guidance. The DCN attribute computations are virtually indistinguishable from conventionally computed attributes while computing up to 100 times faster.
更多查看译文
AI 理解论文
溯源树
样例
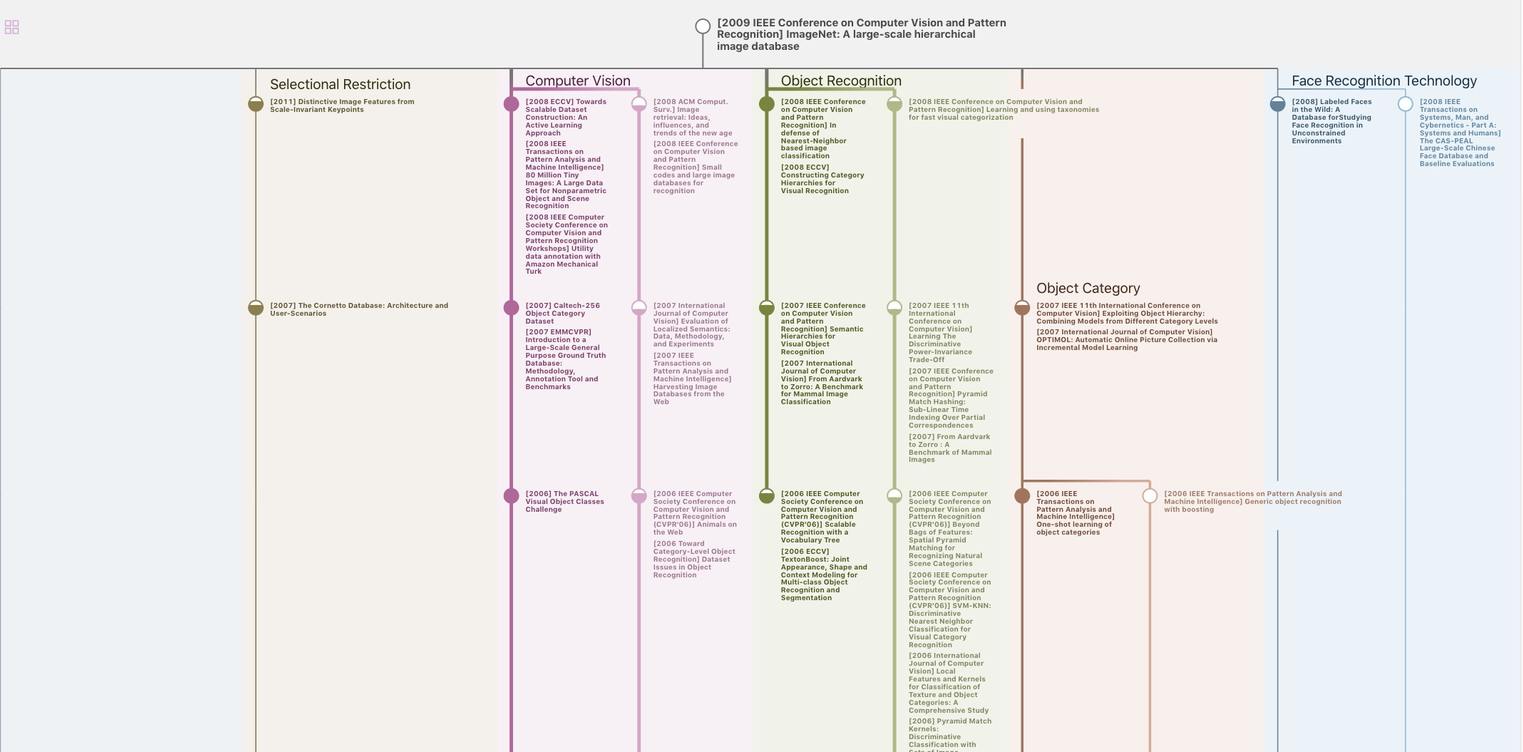
生成溯源树,研究论文发展脉络
Chat Paper
正在生成论文摘要